Jeremy R. Chapman, Deepa Usulumarty
Outcomes of Renal Transplantation
Improving the outcome for patients with end-stage renal disease (ESRD) is the only justification for renal transplantation. If there were not objective and relevant data on outcomes that are meaningful to the patient, then few would take on trust the benefits of a procedure that has the risk of multiple adverse events. The first patients in the 1950s were comparing the potential benefits of transplantation with certain death from ESRD at that time in the absence of dialysis therapy1; some chance of life was better than no chance. Today’s patients face a more complex decision with excellent dialysis treatment available in many parts of the world, and an improving and much better understood alternative in the form of renal transplantation.
The field of renal transplantation is rich with data, and increasingly sophisticated outcome measures and analyses are available to inform patient decisions. Nevertheless, when a patient is faced with a particular clinical problem, it is still common that the evidence base is insufficient to inform a specific decision. Transplantation guidelines that have been created by a number of societies and by Kidney Disease: Improving Global Outcomes (KDIGO) demonstrate through the grading of evidence that many specific decisions can be informed only by “consensus opinion.” At the very least this emphasizes that there is often no right answer; but it also provides a challenge to prove more about the assumptions that are made in clinical practice every day.
This chapter reviews the techniques and factors that deliver the outcome measures available today to inform patients’ decision making about renal transplantation.
Methods of Measurement and Analysis
Types of Outcome Analyses
If the outcomes of 1000 people undergoing transplantation at different times and in different places are measured, some of the patients will inevitably die over the succeeding years. They may die because of the transplant, they may have an accident, or some other intervention may lead to death. How can this be related to the success or failure of the transplant? The most conventional method is to use a Kaplan-Meier plot that yields an actuarial estimate of outcome, as opposed to an actual measure.2,3 Events—deaths in this case—are recorded and the time to that event from the transplant is measured. The Kaplan-Meier method then uses the time of follow-up for each individual to contribute to the analysis, resulting in a better estimate of what will happen to the entire group of patients and not just the small group of patients who have reached a particular time point. An example of such an analysis is shown in Figure 109-1.4 When one is comparing two groups of individuals, there may be issues that should be taken into account such as age. For example, if one group had an average age of 30 years and the other 60 years, differing death rates would be predicted from expectations of what would happen in the general, non-ESRD population. Thus it may be important to show “adjusted” results—for example, adjusted for age and gender—to get appropriate comparisons—for example, between the results in different transplant programs. A multivariate analysis will provide the opportunity to adjust the results for all of the factors that, on their own, appear to affect the outcome. In the 1000-patient example, it may be that there are statistically significant differences in death rates if the group is divided based on age, the presence or absence of diabetes, gender, or the center where the patients underwent transplantation.5,6 With a multivariate analysis, it is possible to see which of these outcomes is influenced by the particular distribution of the others. It may be that one transplant center that appears to have worse results performs transplants mostly on older patients and those with diabetes, whereas another center with excellent results selects only young nondiabetic recipients for transplantation. The multivariate analysis may indicate that both centers would likely achieve the same results if they performed transplants in similar patients.
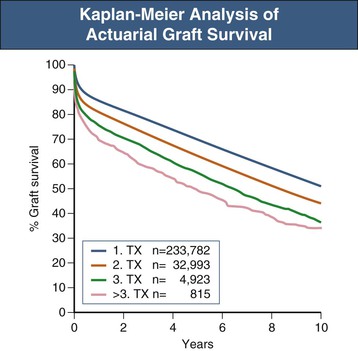
Half-Life Analysis
The half-life analysis is the second type of analysis that is used to extrapolate results beyond where the data stop. In the 1000-patient example it may be that no one has passed the 10-year mark, yet patients seek an estimate of how long their kidney may last. Actuarial analysis may indicate an 80% chance of a transplant lasting 10 years but does not provide information about what happens after 10 years. A half-life analysis allows extrapolation from the known data. There are two different half-life calculations in the literature: the median survival, which is the time for which the middle patient in the series has survived; and the conditioned half-life, which is the median survival of the patients who have survived 1 year or more. Figure 109-2 provides an example of the use of half-life calculations to demonstrate the difference in outcomes related to human leukocyte antigen (HLA) matching between donor and recipient.7
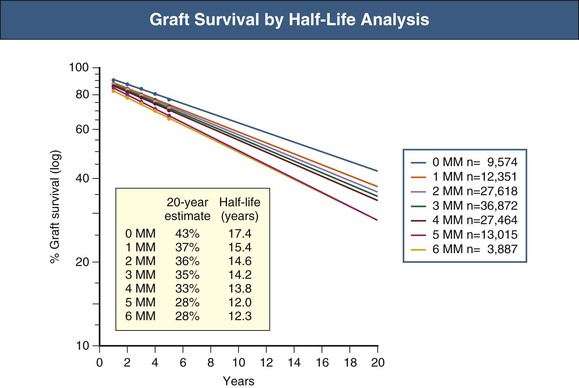
Graft Survival
Graft survival may be presented in two different ways, each valid in its own right, but yielding quite different answers. One of the problems in the field is that many graft survival analyses do not make clear which is being used. It is possible to understand the problem using the 1000-patient example. Assume that 100 patients have died with their transplant still functioning at the time of death and 80 have lost their grafts from rejection and other causes. If one counts death as a cause of graft loss, then 180 of the 1000 patients have lost their grafts; if one ignores death as a cause, then only the 80 have lost their grafts. The latter calculation is best described as death-censored graft survival, whereas the inclusive analysis is described as patient and graft survival. When the analysis is not specified, the findings can be misleading and may make no sense. For example, in the illustrative analysis, the actual patient survival would be 90% (100 deaths in 1000 patients) and the death-censored graft survival would be 92% (80 grafts lost in 1000 transplants), so it might be concluded that 2% or 20 kidneys somehow survive in the absence of the recipient! Both approaches are of course relevant to different situations—on the one hand to understand what causes kidneys to fail, and separately to understand what causes patients to die—but there must be methodologic clarity.
Randomized Controlled Trials
Randomized controlled trials (RCTs) are a conventional way of determining differences between two or more therapies and are used in transplantation especially to evaluate new pharmaceutical products, which require testing in randomized studies before they can be registered for use. The majority of RCTs therefore compare two drug regimens—one with and one without the new agent. Two critical problems compromise the value of RCTs in renal transplantation: First, the relatively small number of transplants performed in any one center means that it is hard to mount studies with sufficient power to detect significant differences; and second, good early surrogate markers for long-term outcomes are lacking. In cardiovascular studies, in contrast, it is often possible to randomize many thousands of patients and detect differences in meaningful outcomes other than death; and in cancer studies it is possible to use short-term evidence of tumor response as a surrogate for longer-term outcomes. In an earlier era of renal transplantation, relatively small studies could be highly informative. An example of an RCT from the 1980s is the Oxford cyclosporine trial,8 in which azathioprine and prednisolone could be compared with cyclosporine with sufficient power to detect a difference with less than 100 patients randomized to each group. This was because the survival rates in the control arm were susceptible to large improvements—5-year graft and patient survival improved by 20% from a baseline around 47% to 67% with the addition of cyclosporine. Today the results of standard of care therapy are so high that recruitment of sufficient patients to detect either graft or patient survival differences at 1 or 2 years is not only extremely expensive but, unless the trials are very large, likely to founder on small random variations in such issues as surgical complications.
In the 1990s the introduction of the composite end point controlled the required size of RCTs. The composite end point is an acceptable indicator of benefit for the U.S. Food and Drug Administration (FDA) and other regulatory authorities to register a drug for therapeutic use. The composite end point includes patient death, graft loss, acute rejection episodes, and loss to follow-up (the last index conservatively defaults the patients who are lost to follow-up as having failed the therapy). Analysis is by intention to treat—in other words, for outcome analysis the patient is assigned as randomized whether or not the patient ever received the assigned treatment. Three key studies confirming the benefit of mycophenolate mofetil (MMF) were conducted using this approach and recruited around 500 patients allocated randomly into three study arms.9,10
Moving forward another 10 years, the largest transplant RCT undertaken to date (the Symphony trial11) required more than 1500 patients to be randomized, and even then was powered only for a surrogate marker of long-term outcome, differences in renal function. It is now accepted that transplant RCTs cannot be sufficiently powered to detect differences in the “hard” end points of patient and graft survival and must all be powered for a composite end point and surrogates of long-term outcomes. Which short-term surrogates provide good predictors of long-term outcomes has yet to be determined. Acute cellular rejection was, in the 1990s, a short-term measure of importance for long-term results, but perhaps not when current immunosuppressive regimens are used.12
Cohort Studies
Some clinical issues are not susceptible to testing by RCT. For example an RCT design will never be able to test whether cancer is more prevalent in patients on dialysis compared with patients after transplantation, first because randomization to dialysis or transplantation is ethically unachievable, and second because the numbers required to power such a study would exceed global transplant capacity. However, it is still important to understand whether the risks change between dialysis and transplantation and thus to understand whether there is any action that can increase or decrease death from cancer after transplantation. An example of an analysis addressing these questions is the Australian cancer study in which data from the Australia and New Zealand Dialysis and Transplant (ANZDATA) registry on a cohort of patients who were first treated for ESRD between 1982 and 2003 were linked to data from the national cancer registries.13 It is critical to ensure the selection of the cohort is appropriate to the question being asked. All too often a cohort study is selected from a convenience sample and thus has critical systematic flaws. A typical convenience sample is “all patients being followed by a particular transplant unit.” The analysis becomes an assessment only of patients still alive and still coming to the transplant unit for follow-up. The cohort has been distorted, the flaw being that the patients not being followed, because of death or graft failure, may be crucial to the analysis.
Meta-Analyses
Some areas of transplantation are rich in both RCTs and nonrandomized studies of a particular intervention—for example, interleukin-2 (IL-2) receptor blockade for induction in renal transplantation. Because the studies have produced variable results, which one should be believed? This issue is approached by meta-analysis of available studies and exemplified by the work of the International Cochrane Collaboration.14 An example of a Cochrane review is shown in Figure 109-3.15 These types of analysis combine the results from patients who have undergone the same interventions from all similar studies, which thus gives much greater statistical power than each study alone can provide. The methodology requires searching for all studies of the question and especially capturing unpublished studies. The problem of publication bias plagues the field; studies with a positive finding for the intervention are more likely to be published than negative studies. The compulsory preregistration of all trials of a drug by commercial entities in a public database such as www.clintrials.gov is designed to avoid this publication bias, and it is now widely accepted that nondisclosure of data by commercial sponsors of clinical trials is neither scientifically acceptable nor tolerated by the regulators.
Case Reports
Can a case report provide valuable information about outcomes after transplantation? There are some instances of rare events where the knowledge from a case report has proved important. One example is the early anecdotal reports of hepatitis B transmission from hepatitis B surface antigen (HBsAg)–negative donors to recipients of their livers.16 A few case reports led to a cohort analysis, which in turn led to an understanding that hepatitis B can be transmitted from a donor who tests negative for HBsAg but positive for hepatitis B core antibody. The surveillance and vigilance strategy for the safety of cells, tissues, and organs of human origin is based on this concept—identifying and publicizing the rare cases so that the problems can be recognized (see www.notifylibrary.org).
Expert Opinion Guidelines
The final type of analysis that is pervasive in the transplantation literature is expert opinion, sometimes leading to clinical practice guidelines. Only the major questions have answers in RCTs, meta-analyses, or cohort studies. The clinician is faced with unanswered questions in day-to-day clinical decision making. Guidelines rate the available evidence on particular questions from good to nonexistent. An example of a widely disseminated set of guidelines is the KDIGO guidelines for the care of a transplant recipient,17 which clearly set out the limits of knowledge in the area. Much of the guidance provided on how to maximize outcomes for particular patients is based on the combined wisdom and experience of clinical practitioners and not on RCT or other data analyses.
Qualitative Studies
Some questions are not amenable to quantitative data collection yet are of great importance to the field. What causes potential living donors to decide to donate? What do physicians consider most important in assessment of living donors? Why does a particular community not donate many organs after death? These questions are amenable to qualitative research and can be assessed and measured, not to produce proof related to a particular question, but to generate testable hypotheses. Qualitative studies create insights into behaviors and attitudes and do not assume that there is a single reality to be discovered; people have different views on organ donation, and any system of measurement that expects everyone to be driven by the same perspectives is going to be disappointed. A guide to the approach of qualitative studies in transplantation has been published to assist scientists familiar mostly with quantitative studies.18
Types of Data Collection
All analyses of data are dependent on the methodology of data collection and completeness of the data. Clearly if the data entered into an analysis are flawed in some way, then the analysis will simply embed those flaws and provide spurious confidence in the outcomes of the analysis. Once the flaws of data collection have been hidden by an analysis, it is all too often that they are never again identified and clinical practice is influenced on the basis of a false assumption. It is thus essential that the reader of any analysis verify for himself or herself whether the data were collected in a manner that is trustworthy and relevant to the question. There are many ways to collect data, all of which have their usefulness when applied in the right manner.
Registries
The methodology of registry data collection varies from voluntary to compulsory and from automated to manual. The completeness of data is related to the amount collected, the frequency with which it is updated, and the methods used. National transplantation registries, such as the ANZDATA registry in Australia and New Zealand19 or the similar Malaysian Dialysis and Transplant Registry, are often voluntary. Some, such as the North American UNOS (United Network for Organ Sharing), database are mandatory, in that financial penalties are threatened for failure to fill in critical data elements.20 The largest international registry is the Collaborative Transplant Study run from Heidelberg, Germany, which uses voluntary data collection on the repeatedly stressed basis that all sequential transplants are entered and followed.4 Donor registries tend to be complete because they capture all data at the time of organ donation as a part of the process of donation.21
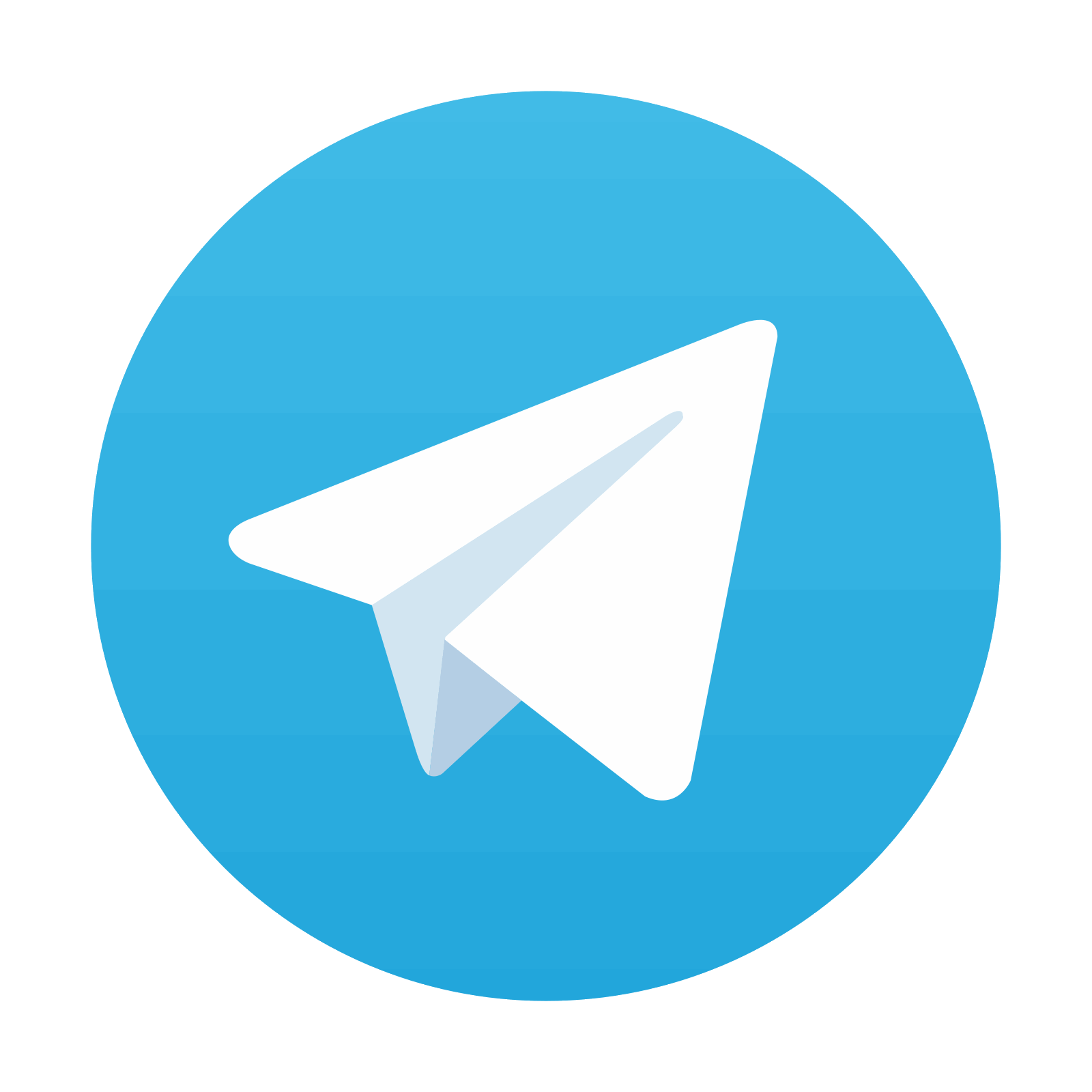
Stay updated, free articles. Join our Telegram channel

Full access? Get Clinical Tree
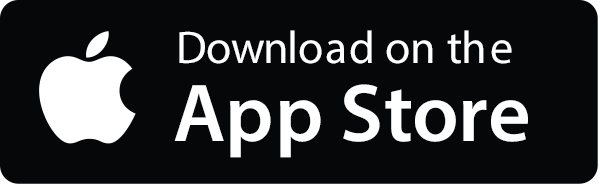
