Abstract
Endoscopic tissue resection is a rapidly evolving field. En bloc resection techniques, specifically endoscopic submucosal dissection, allow for organ-sparing curative endoscopic resection for early gastrointestinal cancers. However, using current techniques to quantify depth of invasion, it remains difficult for endoscopists to reliably select optimal endoscopic submucosal dissection candidates. In this review, we highlight that artificial intelligence platforms can now quantify the depth of invasion of esophageal, gastric, and colorectal neoplasia. While real-time performance evaluation is needed, this represents a significant advancement in endoscopic tissue resection and carries the potential to provide real-time guidance for selecting the appropriate tissue resection technique.
1
Introduction
With the integration of deep learning methodology, artificial intelligence (AI) has rapidly proliferated throughout medicine. This is readily apparent in endoscopy, with computer-aided detection and computer-aided diagnosis (CADx) systems for gastrointestinal lesions .
CADx has the potential to differentiate lesion histopathology, but also to quantify the depth of invasion of early gastrointestinal cancers of the esophagus, stomach, and colorectum . Early gastrointestinal cancers, whether confined to the mucosa or superficially invading into the submucosa carry a low risk of lymph node positivity. In the absence of other high-risk features (poor differentiation; lymphovascular invasion; and tumor budding), R0 resection is considered curative . Given the morbidity and mortality of gastrointestinal surgery , most notably esophagectomy, gastrectomy, and distal colorectal surgery, organ-sparing curative endoscopic resection is a ground-breaking advancement in the field of endoscopic tissue resection.
Allowing for this advancement is the ability to perform safe, effective, and size-independent en bloc removal of gastrointestinal lesions by endoscopic submucosal dissection (ESD) [ , ]. Utilizing an electrosurgical knife within the fluid-expanded submucosal plane, ESD is a meticulous cap-assisted tissue resection technique allowing for precise control over the deep and lateral margins; thereby empowering the endoscopist to perform radical excision without surgery. However, it is technically demanding, and is associated with a heightened risk of adverse events including bleeding and perforation . Therefore, it is predominantly advocated for the removal of early gastrointestinal cancers as benign lesions, especially in the colorectum , are effectively and efficiently removed by endoscopic mucosal resection (EMR).
To delineate a selective resection algorithm, incorporating EMR, ESD, and surgery, the endoscopist has to differentiate between benign lesions, early cancers amenable to curative endoscopic resection and deep cancers which should be referred directly to surgery. Biopsy, although commonly performed, is of limited clinical benefit. It is prone to false negativity due to sampling error and may complicate endoscopic resection by precipitating fibrosis [ , ]. Moreover, radiographic and endosonographic evaluations have limited ability to reliably differentiate clinically relevant depths of invasion and are prone to both under staging and over-staging .
Given the above limitations, real-time optical evaluation is used as the primary modality for delineating endoscopically curable disease. Using high-definition endoscopes with advanced imaging techniques (optical magnification, chromoendoscopy, and virtual chromoendoscopy), pit pattern and vascular pattern changes consistent with invasive disease can be identified. However, optical evaluation is operator dependent and has modest performance characteristics in quantifying depth of invasion, even among expert endoscopists . Moreover, magnifying endoscopy and other advanced imaging techniques (confocal laser endomicroscopy, and endocytoscopy) are not readily available worldwide. This has forced endoscopists to stratify the risk of invasive disease by lesion location, size, morphology, and topography in an attempt to diagnose invisible or “covert” cancer .
Accurate computer-aided depth of invasion evaluation would be a transformative paradigm shift. By providing real-time guidance on selecting the appropriate resection technique, it carries the potential to define selective resection algorithms throughout the gastrointestinal tract. Therefore, we sought out to appraise the literature in this space.
2
Esophagus
2.1
Histological criteria for curative endoscopic resection
For squamous neoplasia, the frequency of lymph node positivity for M1 (intraepithelial) or M2 (lamina propria) disease is negligible. It increases to 8%-18%, 11%-53%, and 30%-54% for M3 (muscularis mucosae), SM1 (submucosa ≤ 200 μm), and ≥SM2 (submucosa > 200 μm) disease, respectively . It is imperative to recognize that these data are commonly not stratified by the absence of high-risk features. In their absence, the frequency of lymph node positivity for M3-SM1 disease approximates the risk of M1-M2 disease . Concordantly, the European Society of Gastrointestinal Endoscopy (ESGE) recommends M1-M2 and M3-SM1 squamous neoplasia, without other high-risk features, as absolute and relative indications for curative endoscopic resection, respectively .
Concerning Barrett’s neoplasia, in the absence of high-risk features, disease limited to the mucosa carries a limited risk of lymph node positivity [ , ]. This appears extendable to lesions confined to SM1 (submucosa ≤ 500 μm) , based on long-term follow-up data after endoscopic resection. Therefore, the ESGE considers mucosal disease and disease limited to SM1, without other high-risk features, as absolute and relative indications for curative endoscopic resection, respectively .
2.2
Depth of invasion platforms
Three AI platforms have automated depth of invasion analysis for squamous cell cancer (SCC) and adenocarcinoma (AC) of the esophagus.
Utilizing a deep convolutional neural network, Horie et al evaluated the ability to differentiate early (T1) vs advanced (T2-T4) esophageal SCC and AC ( Figure 1 ). High-definition nonmagnified white-light and narrow-banding imaging (NBI) images were used to create the training (397 SSC, 32 AC: 8428 images) and validation (41 SSC, 8 AC, 50 normal: 1118 images) datasets. Median lesion size in the validation dataset was 20 mm (range 5-70 mm). The diagnostic accuracy for differentiating early vs. advanced esophageal cancer was 98%. Diagnostic accuracy varied between SCC (99%) and AC (90%).

Involving contributors from the study above, Nakagawa et al evaluated the performance of a deep convolutional neural network to differentiate depth of invasion among superficial squamous neoplasia. The platform was trained and validated using nonmagnified and magnified white-light, NBI, and iodine-chromoendoscopy images (training dataset: 804 SCC, 8660 nonmagnified images, and 5679 magnified images; Validation dataset: 155 SCC, 405 nonmagnified images, and 509 magnified images). The median lesion size in the validation dataset was 18 mm (4-95 mm). When differentiating M-SM1 disease vs SM2-3 disease, the AI platform’s accuracy, sensitivity, specificity, positive predictive value (PPV), and negative predictive value (NPV) were 91%, 90%, 96%, 99%, and 64%, respectively. In comparison, the accuracy, sensitivity, specificity, PPV, and NPV of 16 board-certified experienced endoscopists were 90%, 90%, 88%, 98%, and 66%, respectively. When differentiating mucosal (M) disease vs submucosal (SM) disease, accuracy, sensitivity, specificity, PPV, and NPV were 90%, 89%, 93%, 98%, and 65%, respectively.
Zhao et al evaluated the ability to automate the classification of intrapapillary capillary loops (IPCLs) in squamous neoplasia. Emanating from the subepithelium, IPCLs are capillary loops which are perpendicularly aligned from smooth branching vessels . Progressive variation in IPCL caliber and configuration correlates with depth of invasion and has been classified by the Japan Esophageal Society (JES) : type A (normal epithelium, inflammation, low-grade intraepithelial neoplasia), type B1 (high-grade intraepithelial neoplasia/M1-2 disease), type B2 (M3-SM1 disease), and type B3 (≥ SM2 disease). High-definition magnified NBI images (207 type A, 970 type B1, and 206 type B2) were evaluated. Utilizing a deep convolutional neural network, 3-fold cross validation was performed whereby 3-image groups were created, and 3 AI models were trained independently (training with 2 image groups, validation with remaining image group). Due to limited numbers of IPCL type B3, this grouping was excluded from analysis. Mean sensitivity, specificity, and accuracy were 87%, 84%, and 89%, respectively. Automated performance was most similar to senior endoscopists (>15 years experience: sensitivity 91%, specificity 94%, and accuracy 92%), in comparison to midlevel endoscopists (10-15 years experience: sensitivity 79%, specificity 71%, and accuracy 82%) or junior endoscopists (5-10 years experience: sensitivity 68%, specificity 76%, and accuracy 73%). Automated IPCL classification accuracy was significantly higher than midlevel endoscopists and junior endoscopists ( P < 0.001).
3
Stomach
3.1
Histological criteria for curative endoscopic resection
Histologic criteria for curative endoscopic resection of early gastric cancer (EGC) is stratified by lesion size, presence of ulceration, depth of invasion, and lesion differentiation. As defined by the Japanese Gastroenterological Endoscopy Society, absolute criteria are well differentiated, <2 cm intramucosal (T1a) EGC without ulceration or lymphovascular invasion . The risk of lymph node positivity is effectively zero . Under expanded criteria: (1) intramucosal EGC, well-differentiated, ulcer negative, >2 cm; (2) intramucosal EGC, well-differentiated, ulcer positive, <3 cm; (3) intramucosal EGC, poorly differentiated, ulcer negative, <2 cm, and (4) SM1 (≤500 μm) EGC, well-differentiated, ulcer negative, <3 cm are considered curative. This is due to the low risk of lymph node positivity, as supported by a large series of 5265 EGCs which underwent gastrectomy .
3.2
Depth of invasion platforms
Two AI platforms [ , ] have automated depth of invasion assessment in gastric cancer.
Kubota et al evaluated the ability to automate T-staging for 344 gastric cancers (mean size 40 mm; range 10-240 mm). A total of 902 preoperative endoscopic images were evaluated (T1 448, T2 106, T3 149, and T4 199). A multilayer neural network was trained and validated using a 10-fold cross validation method. Overall, depth of invasion accuracy was 65%. Stratified by T-stage, the diagnostic accuracies were 77%, 49%, 51%, and 55% for T1, T2, T3, and T4, respectively. When evaluating T1a vs T1b EGC accuracy was 69% and 64%, respectively. The PPVs were 80%, 42%, 51%, 56%, 69%, and 68% for T1, T2, T3, T4, T1a, and T1b, respectively.
Zhu et al evaluated the ability to differentiate intramucosal-SM1 (≤500 μm) vs ≥SM2 (>500 μm) using a deep convolutional neural network. Training (790 lesions) and validation (203 lesions) datasets using white-light images were used. For the training dataset, data augmentation was performed by rotating and flipping images, leading to an 8-fold increase in dataset size (6320 images). Automated sensitivity, specificity, accuracy, PPV, and NPV were 77%, 96%, 89%, 90%, and 89%, respectively. In comparison to 17 endoscopists with varying experience, sensitivity, specificity, accuracy, PPV, and NPV were 72%, 88%, 63%, 56%, and 91%. Significant differences in accuracy and specificity, favoring CADx, were identified.
4
Colorectum
4.1
Histological criteria for curative endoscopic resection
Within the colorectum, cancer is defined by invasion into the submucosa. This is because the mucosa does not have lymphatic drainage and therefore does not carry the potential for lymphatic or metastatic spread . The overall risk of lymph node positivity is 13% for T1 colorectal cancer. However, it decreases to 1.9% in well-differentiated lesions, confined to SM1 (≤1000 μm), without lymphovascular invasion or tumor budding . Both the Japanese Gastroenterological Endoscopy Society and the ESGE utilize the above criteria for curative endoscopic resection.
4.2
Depth of invasion platform
Takeda et al evaluated the ability of a machine learning platform to differentiate adenomatous lesions from invasive cancer ( Figure 2 ). Endocytoscopy images were used from 375 lesions (mean size ± standard deviation: adenoma 11 mm ± 10 mm; invasive cancer: 31 mm ± 14 mm). Endocytoscopy, performed after application of crystal violet and methylene blue dye, allows for x380 magnification and enables in vivo evaluation of both nuclei and gland lumens. A total of 5843 endocytoscopy images were evaluated, with 200 of them used for validation. Sensitivity, specificity, accuracy, PPV, and NPV were 89%, 99%, 94%, 98%, and 90%, respectively. High confidence (≥90% probability of being correct) was achieved in 72%, and under these conditions, sensitivity, specificity, accuracy, PPV, and NPV were 98%, 100%, 99%, 100%, and 99%, respectively.
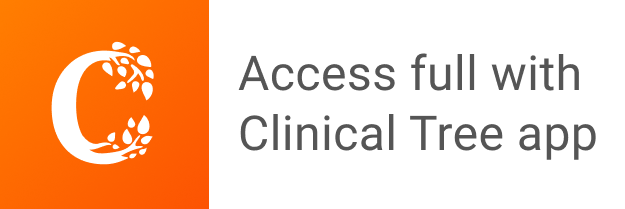