Abstract
As AI becomes increasingly ubiquitous in our world, it is set to transform every aspect of how we do medical care, research and education. Physicians as a profession need to be active leaders and participants in this technology-driven transformation in order to ensure that the potential to dramatically improve health care is fulfilled. This article is focused on enabling that active participation by helping physicians gain understanding of the core concepts, issues, and trends related to AI (using the common board use of the term which includes Machine Learning, Deep Learning, Augmented Intelligence, and Artificial General Intelligence).
1
Introduction
“The philosophies of one age have become the absurdities of the next, and the foolishness of yesterday has become the wisdom of tomorrow.” – Sir William Osler
The history of medicine has many key inflection points where our profession needed to evolve and develop from when Louis Pasteur identified germs as cause of disease to when William Osler created the modern system of medical education to the Evidence-Based Medicine (EBM) movement that gave us the ability to deal with the explosion of published evidence.
At the turn of the last century, there was a concerted effort to imagine the “future of medicine” and how to best prepare the profession to succeed in modern times. One of the major outcomes was the reinforcement of the need for professionalism in medicine and the second was the realization of the increasing rise of nonclinical work as one of the core aspects of what the physicians do. In a model developed in Canada and since adopted around the world, the CanMEDS 7 roles recognize the key aspects of how the role of Medical Expert has evolved to include and integrate with the other 6: Communicator, Collaborator, Leader, Health Advocate, Scholar, and Professional.
The next inflection point is upon us–AI is set to transform medical care, research, and education and while most still see AI as a technology that will become mainstream one day in the future, we are already at the inflection point where some type of AI is deployed in many of our daily activities from occupation related to home and social. We need to prepare for how to best introduce AI in order to support all 7 of the CanMEDS roles of the competent physician of the future. Going beyond the hype and fears driven by so-called experts, headlines, and science-fiction, we must consider how can we engage with this emerging power force, and harness it to improve what we do. The greater use of AI has a tremendous potential to discover new insights about disease risk factors, diagnosis, progressions, and treatments where we have until now been stymied by complexity and mountains of data, especially in the most complex systems such as in gastroenterology, immunology, and endocrinology .
With the increasing use of AI in every aspect of our world, there is a sense of “déjà vu” for those of us who witnessed the beginnings of the digital world and how healthcare painfully digitized, a laggard behind most other industries. There are many reasons why healthcare is slower to adopt technology as it evolves–including regulatory and funding issues, as well as the complexity of the domain combined with the need for higher standards of performance. Some of these reasons are beyond our control, but the main reason that we can definitely manage proactively is our profession’s understanding of AI and ability to direct its use and evolution.
This is very similar to the EBM movement [ , ] mentioned earlier that rose the 1990s in response to limitations in the understanding and use of the exponentially increasing published evidence. The EBM approach included educating clinicians and the development of a suite of methods, tools, and solutions that collectively helped evolve the practice of medicine to better leverage our growing scientific knowledge.
Physicians need to be able to understand the core concepts of AI, where it can and should be applied and how to help medical AI evolve from early challenges to successful tools. We have to step into the roles of codesigners and active users, who can recognize AI that is well done vs not. We also need to be aware of the potential of such innovation, just as any other type of invention, to create disruption and change patterns of practice, payments and even entire specialty domains. We need to find ways of welcoming such disruptions by celebrating the better outcomes achieved and whenever necessary, by creating flexible career tracks to accommodate the changes in clinical practice that impact individual physicians.
Initial and future applications of AI to Gastroenferology (GI) are covered in the rest of this journal issue, so this article will focus on helping clinicians understand the core concepts of the AI domain and how to best proceed to learn more in order to be able to meet the opportunities that are made possible through this evolution in the technologies available to us.
2
Larger context
Experts agree that the rise of AI in all aspects of our world is a core driver of what Professor Klaus Schwab, the founder of the World Economic Forum has called the Fourth Industrial Revolution which is behind the current global transformation of our economies and societies. Water and steam power mechanized production and created the First Industrial Revolution. Electric power then created mass production, which led to the Second Industrial Revolution. The Third Industrial Revolution, the digital revolution that has been occurring since the middle of the last century, occurred when electronics and information technology enabled the automation of production. The current Fourth Industrial Revolution is building on the Third, and is a conversion of different technologies domains that is blurring the lines between the physical, digital, and biological spheres, reminiscent of the futurist author Professor Ray Kurzweil work on the Singularity concept as the moment at which machines intelligence and humans would merge.
The history of AI is relatively recent. It was only in 1950, that English mathematician and World War II hero of Bletchley Park fame (Britain’s codebreaking center) Alan Turing published a paper that famously began by posing the simple question “Can machines think?” . He then proposed a simple test that could be used to prove that machines could think in a way indistinguishable from an intelligent human, which the author called the “Imitation game”, but it became known world-wide as the Turing test. Entitled “Computing Machinery and Intelligence,” this paper established the domain that would come to be known as Artificial Intelligence (AI), a term first coined by Stanford Professor John McCarthy in 1956 when he held the first academic conference on the subject.
AI as a field of innovation experienced some early enthusiasm in the 1960s and a long period of skepticism, that was termed “AI Winter”, until a few years ago and we are witnessing a resurgence of interest and progress. Most emerging innovations follow this pattern, including for example personal computers, internet, and smartphones. The Gartner Hype Cycle model ( Figure 1 ) Figures 2 and 3 , developed by Gartner group based on their research provides a clear time-line for the adoption of new technologies and we are in the mass adoption phase of AI. The Gartner Hype Cycle model has 5 phases: (1) Innovation Trigger that generates significant initial interest, (2) Peak of inflated expectations marked by over-enthusiasm in the absence of real results, (3) Trough of Disillusionment when the innovation is no longer considered promising, (4) Slope of Enlightenment for people who persist in understanding and developing the innovation, and (5) Plateau of Productivity as the opportunities created by the innovation are increasingly visible and use becomes widespread.

So the “Winter of AI” has led most of society to conclude that AI is still years away, while those closer to the field are seeing the clear signs that after a few decades of gradual progress along the fourth stage of Slope of Enlightenment, we are now finally hitting the Plateau of Productivity.
Why are we seeing a resurgence of AI right now? Beyond the several decades of innovators persisting to create breakthroughs in the long Slope of Enlightenment phase, AI success is enabled by 3 tech trends that are converging : first, exponential growth in the speed of computers (Moore’s Law), second, explosive growth in the amount of data available, and third, the wide adoption of cloud computing.
While health care is a perennial late comer to technology adoption, the additional challenges in the case of AI adoption in particular are because of 2 of the 3 technology trends identified: data issues and the slow adoption of cloud. Health organizations have limited data sets and are not set up to share them easily, as they are using older generation IT infrastructure instead of newer cloud-based systems. Even when they manage to integrate data sets, as they all label their data in different ways, an AI system is only going to be as good as the data it is drawing upon to learn from.
Unfortunately, most people, not just physicians, have limited understanding of the AI domain as it is a new and rapidly changing field. The current state of perception vs reality can be summarized in Figure 2 .

3
Key provisions
3.1
First–what is AI? A state of confusion
The most important step in working with AI is to always clarify what is meant by the term in that particular instance. In the media and in the workplace, AI has become a term that loosely refers to anything related to software driven automation and complex algorithms. The abbreviation itself is unclear as AI can refer either to Augmented Intelligence (AuI)–algorithms that support the work of humans with additional insights (also known as Human-in-the-Loop)–as well as true Artificial Intelligence as first defined by Alan Turing–the concept that machines develop human-like intelligence so they can act without humans and can replace and perform tasks that normally require complex human intelligence and reasoning, essentially, acting as smart as a human. To differentiate the 2, some experts are calling for the introduction of AuI to designate the first and Artificial General Intelligence (AGI) to designate the second.
In the United States, the recent legislation “Fundamentally Understanding the Usability and Realistic Evolution (FUTURE) of Artificial Intelligence Act of 2017” defines “general AI” as computational methods that produce systems that exhibit intelligent behavior at least as advanced as a human across the range of cognitive, emotional, and social behaviors. In contrast, the bill defines the term “narrow AI” as computational methods that address specific application areas, such as playing strategic games, language translation, self-driving vehicles, and image recognition. Thus, these AI methods and tools for the foreseeable future are better characterized as narrow AI that augments human intelligence (AI as AuI). The American Medical Association (AMA) has officially stated that its House of Delegates will use AI as the abbreviation of the term AuI. This state of confusion means that it is best to always confirm that is meant by the acronym in each particular instance.
The most common current use of AI is to designate something which is called by the experts Machine Learning (ML) , meaning the application of sets of algorithms to analyze a given situation, with the ability to learn from feedback about the outcome of the analysis in order to self-adjust and improve accuracy. For example, one can choose data such as x-ray images or patient symptoms from a questionnaire and create an algorithm that identifies red flags as defined by the clinician. As the algorithm is applied, the machine can be asked to learn from its success rate, based on the feedback of whether or not the clinicians accepted its answers as true or false negatives/positives. Deep Learning (DL) is the next generation version of ML. While ML uses algorithms to parse data, learn from that data, and make informed decisions based on what it has learned, DL structures algorithms in layers to create an “artificial neural network” that can learn and make intelligent decisions on its own.
Therefore, even as ML, DL, AuI, and AGI have in common the idea that computation is a useful way to model intelligent behavior in machines, they are not synonymous , contrary to how they are now commonly being used. They vastly differ in the extent of how capable and how independent they are: they can be simply executing what they are told vs continuously learning vs creating their own approaches that are usually incomprehensible to humans (ie, a black box). A visual description can help the reader understand how they are related ( Figure 3 ) .
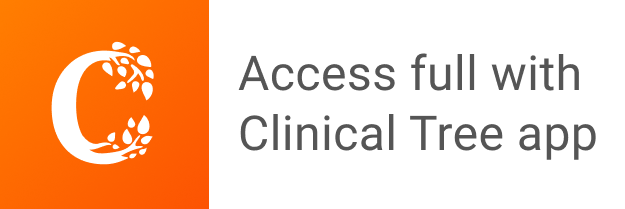