Abstract
Optimal success of colonoscopy for prevention of colorectal cancer is currently measured by adenoma detection rate (ADR), which reflects a colonoscopists ability to identify colorectal and remove precancerous polyps. Among colonoscopists in the same health care system and shared patient population, ADR varies from 7% to 53%. For every 1% increase in ADR, risk of interval colorectal cancer is reduced by 3%-6%. Beyond attaining excellent exposure of entire mucosal surface during colonoscopy, ADR can be improved with a second observer. Computer-aided detection (“facial recognition” for polyps) has potential to improve ADR as a second observer. Several groups are working to bring this technology into the endoscopy unit. Success will require real-time implementation of an affordable system with very high accuracy and proven benefit to improve ADR and reduce miss rate of precancerous lesions. In just the past year, computer-aided detection systems that run live during colonoscopy have been shown to improve ADR using affordable off-the-shelf computers.
1
Introduction
Colorectal cancer (CRC) is the second leading cause of cancer deaths in the United States . The vast majority of colorectal cancers start as benign precancerous polyps, such as adenomas . These polyps typically have a mean dwell time of around 10 years or more prior to transforming into CRC . Colonoscopy remains the gold standard for finding these precancerous polyps and is the only nonsurgical intervention capable of removing them. The National Polyp Study (NPS) showed that up to 90% of colorectal cancers are preventable with polyp removal . The same NPS colonoscopy cohort had a 53% decrease in CRC mortality compared to the SEER population, in whom an unknown percentage had colonoscopy .
Adenomas are the most common type of precancerous polyp . The adenoma detection rate (ADR) represents the percent of colonoscopies in which at least one adenoma is found. Ideally, ADR should equal adenoma prevalence, estimated to be greater than 50% among the screening age population [ , ]. Unfortunately, ADRs vary widely, with some colonoscopists having ADRs as low as 7% . Furthermore, in tandem colonoscopy studies, up to 24% of adenomas were missed . Missed adenoma rate is reflected, in part, by the gap between ADR and adenoma prevalence. Two large studies showed that for each 1% increase in ADR, the interval colorectal cancer rate was decreased by 3%-6% [ , ]. Kaminski et al further showed that colonoscopists can be trained to improve ADR and achieve lower interval colorectal cancer rates. These data emphasize the importance of “leaving no polyp behind” to achieve the potential for 90+% prevention of colorectal cancer with colonoscopy. Accordingly, ADR has become a key quality measure reportable to the Centers for Medicare and Medicaid and affects reimbursement under the Medicare Access and CHIP Reauthorization Act of 2015 and Merit Based Incentive Payments System since 2017 .
A colonoscopist’s ADR is positively correlated with level of training, time spent during withdrawal, inspection technique, and bowel preparation quality . Higher ADR can be achieved with special training [ , ], second look , Narrow Band Imaging , trained second observers , and knowledge that the colonoscopy is being recorded . Scope attachments and modifications that expose mucosal surfaces behind folds and stabilize views such as Endocuff and Amplifeye are also associated with increased ADR. Interestingly, multicamera systems designed to expose more of the mucosal surface on multiple viewing screens have failed to consistently improve ADR. These latter results suggest that the small angles of human central/macular vision (5% and 18% of entire visual field, respectively) may neutralize the benefit of technologies that improve mucosal surface area exposure if displayed on multiple or large screens during colonoscopy. Indeed, gaze pattern has been shown to affect ADR . Hawthorne effect as well as human variability in gaze pattern and limitations in central/macular vision may explain improved ADR with second observers.
2
Computer-aided detection
Computer-aided detection (CADe) of polyps has the potential to function as a second observer and reduce the miss-rate of polyps. When utilized in conjunction with quality colonoscopy practices, CADe has the potential to close the gap between ADR and adenoma prevalence and therefore reduce interval colorectal cancer rates. To achieve these lofty goals, a CADe technology must be real time (<10 ms latency), easy to implement, reliable, provide near 100% sensitivity, and a nondistracting low false positive rate. Widespread use and demand for this technology will depend on proven ability to increase ADR, clearance by regulatory agencies, and financial benefit for users. Beyond the potential for increased reimbursement through merit-based incentive systems, a reimbursement code would seal the deal for many potential users.
3
The past, present, and future
CADe of polyps has a surprisingly long history. In 2003, Karkanis et al described computer-assisted polyp detection software that used color and texture analysis to identify polyps . Their system, Colorectal Lesion Detector, had an accuracy greater than 95% for polyp identification but was applicable only on static images due to high latency. Later studies evaluated shape [ , ], spatiotemporal features , and edge features for polyp detection . For example, based on just 25 unique polyp images, Tajbakhsh et al created a CADe system using a hybrid shape analysis and achieved 88% sensitivity and 300 ms latency , still much too long for real-time feedback on a video stream.
These early CADe systems relied on brute force programming and early machine learning techniques, in which human programmers inform the computer about unique polyp features. Inherently, these techniques are resource intensive, time consuming, and impose human bias and error onto the software. A viable future of CADe for polyps had to wait until this decade when deep learning models using convolutional neural networks (CNNs) became available. CNNs take on the task of discovering polyp-specific features, independently of human input, learning much like a human learns to recognize a face. Now, with increasingly powerful and affordable graphics processing units (GPUs), we are at the cusp of developing and running highly accurate CNNs for polyp detection with latencies less than 10 ms, easily capable of real-time analysis during colonoscopies running at 60 frames per second.
In 2016, Li et al were one of the first to describe a deep learning system used for polyp detection based on images. However, their system was only able to achieve an accuracy of 86%, sensitivity of 73% . In 2017, Wang et al presented their CNN, developed using SegNet Architecture, trained on greater than 5000 annotated images and validated on >27,000 images [ , ]; however, their described system had a latency of 77 ms operating at 25 frames per second. In 2018, Misawa et al described their version of a deep learning algorithm with a false positive rate of 60% .
In 2018, Urban et al described the development of a CNN algorithm theoretically capable of operating in real time with a latency of 10.2 ms (98 frames per second), achieving an accuracy of 96%, sensitivity of either 96.9% (assuming a 5% false positive rate) or 88.1% (assuming a 1% false positive rate) . Their algorithm was further validated on over 5 hours of colonoscopy videos. The algorithm missed no polyps found by expert reviewers and had a false positive rate of 7%. When videos were reviewed with overlaid CNN, expert reviewers found 20% additional polyps. While flawed as a retrospective single-center video validation study, the high performance achieved by their CNN demonstrated the potential of this new technology.
In 2019, Wang et al published results of the first randomized trial of CADe for polyp detection in a single center of 1058 colonoscopies in China . Their CNN achieves a per-image sensitivity of 94.4% and per-image specificity of 95.9% with a latency of 77 ms (25 frames per second). Suboptimal latency necessitated use of 2 viewing screens, one showing the native colonoscopy video stream viewed by the colonoscopist, and the other showing the AI-processed video stream. An alarm system was developed to notify the colonoscopist when the AI predicted the presence of a polyp. The artificial intelligence (AI) system significantly increased ADR (29.1% vs 20.3%, P < 0.001) and the mean number of adenomas per patient (0.53 vs 0.31, P < 0.001). This study is the first to demonstrate the utility of CADe live during colonoscopy, and the first to show that its use improves ADR.
Progress is rapid toward a viable CADe system that achieves accurate polyp detection in real time on one screen. This has likely already been achieved but unpublished. Current CADe CNNs for polyp detection already demonstrate performance characteristics that are at the cusp of live implementation after regulatory clearance. But what of the other AIs being developed for colonoscopy and other endoscopic procedures. How will these AI “apps” play together in a single hardware/software solution? Can they even be run simultaneously with current hardware/GPU limitations in an affordable and compact form-factor? There is no doubt that they will, perhaps sooner than we expect!
4
Conclusion: Embracing AI
New technologies designed to reduce colorectal cancer deaths often strike fear and skepticism in colonoscopists. Will novel stool and blood tests, virtual colonoscopy, colon pill cameras and x-ray devices, or polyp chemopreventive drugs put my career at risk? None of these technologies have reduced the demand for expert colonoscopy for definitive screening, diagnosis, and intervention. In many cases, these technologies have increased demand by engaging more of the population in screening.
We are at the cusp of reaping benefit from AI for colonoscopy. A CADe that behaves as an expert second observer and improves our detection of polyps will reduce the risk of interval colorectal cancers. However, even the most accurate CADe system cannot assist detection of polyps that are not exposed, it cannot compensate for inadequate cleaning, low cecal intubation rate, fast withdrawal time, and poor inspection technique. Colonoscopists remain responsible for the care of his or her patient, for deliberately exposing all mucosal surfaces, interpreting potential pathology, and determining appropriate intervention. It will be a long time before AI and robotics can replace a skilled colonoscopist.
But what if the use of CADe desensitizes our acuity to recognize polyps? What if we ignore important lesions unrecognized by CADe? What if we become dependent on and overly trusting of CADe and remove all identified lesions, even those that require no intervention such as a suction pseudopolyp or inverted diverticulum? Future studies will be needed to determine if use of CADe systems sensitize or desensitize acuity for recognition, interpretation and appropriate intervention of suspected abnormalities, especially among those in fellowship training. In the meantime, we should embrace CADe systems only as a “second observer,” one that questions us: “what is this; is it important?”
The near future is likely to bring other benefits of AI to colonoscopy, including automated cecal intubation rate , withdrawal time , Boston bowel prep score , polyp size , polyp pathology , Mayo Endoscopic Score , etc., enabling automated endoscopic reports, standardized scoring, and quality measure reporting. For example, CADe systems are under development by several groups capable of both polyp detection and polyp optical pathology prediction ( Figure 1 ). The future appears bright for AI-assisted improvements in the delivery of efficient, accurate, and cost-effective patient care .
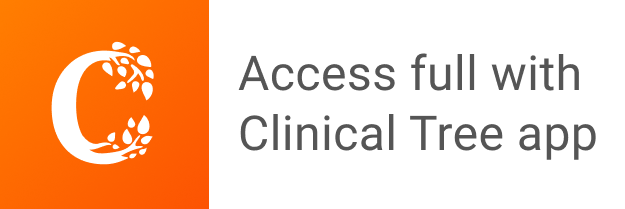