Abstract
Background: Artificial intelligence (AI) is exponentially gaining interest and utilization in medical fields. Deep learning, a particular branch of AI under machine learning, started a revolution in AI by learning to recognize complex features itself, without dependence on a priori human-generated rules of classification. In recent years, several applications of AI are emerging in gastrointestinal endoscopy. Computer-aided detection and diagnosis might be the solution for the operator dependency in endoscopy. In this review, we aim to provide an introduction for gastroenterologists to the complex terminology that is linked to AI, the current state of play in AI-assisted endoscopy, and its future directions. Methods: We performed a literature search on MEDLINE and PUBMED through May 2019 for relevant articles using keywords as AI, deep learning, computer-aided detection and diagnosis, and gastrointestinal endoscopy. Results: AI-applications in endoscopy described in the literature included colorectal polyp detection and classification, assessment of cancer invasiveness, video capsule endoscopy, detection of esophageal and gastric cancer, and Helicobacter pylori gastritis. Conclusion: AI-assisted endoscopy is a strongly evolving field and recent innovations and research on this subject are promising. Initial important steps along the AI-road have been taken by initiating the first prospective studies on AI-assisted endoscopy to minimize the risk of selection bias and overfitting of the AI-models. Future research will investigate if AI-assisted endoscopy will refine our current endoscopic abilities.
1
Introduction
Artificial intelligence (AI) is exponentially gaining interest for the utilization in medical fields. In recent years, there has been a proliferation of technical advances in AI. Several applications of AI are already rooted in our everyday life; the automatic detection of spam in our e-mail, Google’s predictive searches when you type a search term, personalized online shopping based on your shopping habits and preferences, and many more. Applications of AI are thought to be a potentially great asset for health care, especially in image-based specialties as gastroenterology. The question remains what AI will change for future gastroenterology practice–and when. In this review, we aim to elucidate the complex terminology that is inextricably linked to AI, the current state of play in AI-assisted endoscopy and future directions.
2
Background AI
AI can be defined as forms of intelligence, which are normally associated with humans such as “learning” and “problem solving”, performed by a computer . Although AI, machine learning (ML), and deep learning (DL) are often being mixed up by media, they are distinct entities. AI is the theory and development of general computer systems of which ML is a branch. ML is a collection of computer algorithms that normally require human intelligence. DL is a subset of ML that is based on a specific ML method called “artificial neural networks”, which are loosely inspired by the interconnected neurons in the human brain . ML is characterized by the ability of a machine to automatically dissect data, to recognize, and learn certain patterns and eventually, after a certain amount of training, to correctly apply the learned pattern recognition on new data . Although the theory and idea of DL date back to 1950, it only recently became possible to solve very complex problems once new optimization algorithms, large scale and well annotated data, and computational sources (hardware) became available. With the increasing availability of digital healthcare data, it is highly promising that DL models will automate many tasks such as performing simple, cost-effective, and widely available examinations and analysis as well as more complicated tasks including performing surgery and detecting and diagnosing tumors in medical images .
After the first breakthrough of DL methods in 2012 , many traditional ML methods (random forest, support vector machines [SVM], and Gaussian mixture models) started to be replaced by DL approaches due to their superior efficiency and accuracy. While traditional ML approaches rely largely on predefined engineered features (ie, expert knowledge are encoded through these features), DL algorithms do not require explicit feature definition but instead they utilize data and combine high-dimensional hard-to-interpret features to derive a conclusion. In this manner, DL algorithms are often called “black-box.” In other words, DL algorithms learn rules directly from data, not from experts to encode specific features.
2.1
Deep learning
The majority of the DL algorithms are based on supervised learning , in which data and its associated labels are available for finding an appropriate mapping between them. There are alternative learning strategies as well; for instance, unsupervised and semi-supervised learning algorithms are being explored by ML experts, when either labels are not–or sparsely–available for the data. To train a DL system (a neural network) with a high degree of accuracy, well-curated large volume datasets with precise annotation (eg, polyps labeled as hyperplastic or adenoma) are required (supervised). The more complex the DL model is, the more data are needed. The most commonly used form of DL is based on neural networks with convolutional filters, namely Convolutional Neural Networks (CNN). A typical CNN contains a series of layers mapping input images to output labels while learning increasingly higher-level imaging features . Layers between input and output layers are called “hidden” layers, which basically include thousands of filtering operations (convolutions) to derive a variety of image features at different resolution, scale, and level of details. Considering that a layer is a nonlinear mathematical function, having hundreds of layers in a neural network is capable of modeling a very complex mathematical relationship in the data due to compositional effects of layers. Features from hidden layers are processed and combined at the last layer to produce an output label (eg, type of polyp). Depending on the application, this output label can be a segmentation map, diagnosis, staging, and even survival prediction of a patient.
Despite remarkable success of DL, there also exist several limitations withholding further development and application of DL in healthcare such as lack of massive well-annotated data . While computer vision applications can easily use millions of imaging data with DL networks, radiology, pathology, and other healthcare imaging systems are only approaching thousands of data at best. Usually, this data scarcity problem is solved through 2 approaches: data augmentation and transfer learning . Data augmentation refers to the artificial expansion of training datasets by slightly modifying available images in order to create more training data. Transfer learning is a technique comprising the pretraining of algorithms on a general database of images (ImageNet), before fine-tuning the algorithm by training on task-specific images. Another problem in DL algorithms is the lack of interpretations in diagnostic systems. DL algorithms are good at predicting outcomes, yet they do not reveal the features upon which the prediction is based and this is referred to as the black-box nature of DL algorithms. Despite recent efforts in the literature towards opening the black-box of the DL algorithms, the problem is not solved at large. The last major problem of DL algorithms is that they are vulnerable to variation in the data. Recently, Finlayson et al showed that even small data manipulations can likely fool a targeted DL model, raising concerns about reliability of DL systems in diagnostic settings. Hence, there is a significant need for developing DL algorithms resilient to data manipulations.
3
Current state of play
3.1
Artificial intelligence in colonoscopy
Computer-aided detection and diagnosis (CAD) of polyps has been one of the primary areas of research on AI-assisted endoscopy. AI-assisted colonoscopy has mainly been evaluated in 3 different settings, namely polyp detection (CADetection or CADe in short), polyp characterization (CADiagnosis or CADx in short), and determination of invasiveness depth in cancerous lesions. Numerous AI-models are thoroughly investigated on still images or stored videos. However, the challenge remains to apply these models in real-time colonoscopy, without significant delay, in order to be a valuable addition in the diagnostic process. To date, only 2 studies have applied the AI-model during real-time colonoscopy [ , ]. Furthermore, only 1 study included sessile serrated polyps in a study examining the accuracy of a CADe system, while this subgroup is accountable for up to 30% of all colorectal cancers .
3.2
Polyp detection (CADe)
Colonoscopy screening aims to detect and prevent colorectal carcinoma (CRC) . However, adenomas are missed during colonoscopy extending to a miss-rate of 27% caused by several patient-, polyp-, and operator characteristics [ , ]. These missed polyps are an important target for colonoscopy improvement, as former literature showed that a 1% increase of adenoma detection rate (ADR) is associated with a 3% decrease in colon cancer risk and a 5% decrease in colon cancer death . Addition of a second observer during colonoscopy leads to significant increase in ADR, suggesting that lesions within the visible field are missed [ , ]. Considering the CADe model as a second observer, it could improve the detection of mucosal lesions and eventually even decrease the risk of interval cancer. Particularly in endoscopists with minor limited experience or a relatively low ADR, addition of a CADe system might improve ADR to proficiency or expert levels ( Figure 1 ). In 1999, Karkanis et al developed 1 of the first handcrafted CADe for polyp detection in colonoscopy and numerous research groups have developed more cutting-edge AI-based models since . The results were promising with detections rates ranging from 76.5%-100.0% . However, most studies were still limited by high false positive rates and their retrospective design, since ML models were applied on colonoscopy still images. The issue of false positive detection is less problematic in practice, as ML-annotated “abnormal areas” are always further evaluated by the endoscopist to confirm or exclude a polyp prior to resection.

Recently, Wang and colleagues performed the first prospective, nonblinded randomized controlled trial examining a real-time CADe during diagnostic colonoscopy vs only colonoscopy. A DL-based CNN was formerly developed and validated for polyp detection , providing both visual as audial notices when a polyp is detected on the screen. In total, 536 routine colonoscopies were compared with 522 CADe colonoscopies, performed by 8 colonoscopists. The results showed a significant higher ADR (20% vs 29%) and PDR (29% vs 45%) in the CADe group. The increase in ADR was largely attributable to the increased detection of diminutive adenomas. The withdrawal time was slightly increased in the CADe group (6.9 minutes vs 6.3 minutes). No false negative cases and only a small number of false positive cases were reported in the CADe group (n = 39, 0.075 per colonoscopy), largely caused by bubbles, wrinkled mucosa, residual fecal material, and local inflammation. Furthermore, there was a 2-fold increase in the detection of diminutive hyperplastic polyps, which could be interpreted as unnecessary distraction, polypectomies, and work load, although it is likely that endoscopists rapidly recognize hyperplastic rectosigmoid polyps as benign and ignore the detected alert. An important limitation of this study is the quite low baseline ADR (0.20), which questions the external validity of this automatic polyp detection device to regions with higher reported ADR. Still, endoscopists with less experience or a low ADR might benefit. Combining both, a highly accurate CADe as a uniform CADx system might reduce the consequences of detecting more diminutive hyperplastic polyps. Mori et al combined their CADe algorithm, using WL, and their CADx algorithm, using endocytoscopy, with promising preliminary results. Future studies should investigate if a highly accurate CADe + CADx model will lead to generalized increases in adenoma detection and classification while limiting false positive alerts.
3.3
Polyp characterization
Screening colonoscopy and contingent polypectomy is considered one of the most effective methods to prevent CRC . However, histopathological examination of removed polyps leads to substantial costs and workload. Particularly histological analysis of diminutive polyps, which accounts for the majority of colorectal polyps found during colonoscopy, might be redundant. Optical biopsy is proposed to be helpful in reducing these associated costs and workload. Optical biopsy is the principle of assessing polyp histology based on visible features. Enhanced imaging tools are developed to aid in real-time assessment of polyp histology and might enable the “resect-and-discard” and “diagnose-and-leave” strategies for eligible diminutive polyps, resulting in a shorter procedure time, less adverse events due to unnecessary polypectomies and significant lower costs . However, outside of expert centers, the ASGE Preservation and Incorporation of Valuable endoscopic Innovations threshold for these technologies (>90% NPV) were not yet achieved [ , ]. Optical biopsy of colorectal polyps could be significantly improved by developing highly accurate computer-aided diagnosis (CADx) devices and might be the starting point for a universal shift to a resect-and-discard and diagnose-and-leave paradigm in eligible polyps. Several study groups evaluated CADx models on colonoscopy with enhanced imaging pictures and videos, using magnifying NBI , magnifying chromoendoscopy , and endocytoscopy . Diagnostic accuracy rates ranged between 84.5% and 98.5%. Since most of these technologies are not worldwide available, we shall not further discuss them in this review.
Sánchez-Montes and colleagues designed and assessed a CADx for the prediction of dysplasia based on surface patterns (using a SVM) using conventional WL endoscopy images . The study comprised 225 single polyp images and sensitivity, specificity, PPV, NPV, and accuracy were respectively 92.3%, 89.2%, 93.6%, 87.1%, and 91.1%. Renner and colleagues trained and tested a CADx applying a DL-CNN to differentiate between adenomatous and nonadenomatous polyps based on WL and NBI images . In total, 186 high-definition pictures of 100 polyps were included in the test set. Per case analysis showed a diagnostic accuracy, sensitivity specificity, PPV, and NPV of respectively 78%, 92.3%, 62.5%, 72.7%, and 88.2%. The diagnostic accuracy of the CADx model was compared with the accuracy of 2 expert endoscopists and turned out to be not significantly different. Interestingly, Byrne et al. developed a DL-CNN to distinguish colorectal polyps in adenomas and hyperplastic polyps on unaltered NBI videos ( Figure 2 ). In 106 of 125 videos, the CADx model achieved enough confidence to predict a histological outcome. In comparison with the definite histology, the diagnostic accuracy was 84%, sensitivity 98%, specificity 83%, NPV 97%, and PPV 90%. Although the CADx was retrospectively applied on the videos, it could perform almost in real-time with only 50 milliseconds delay.

3.4
Assessment of invasiveness depth CRC
In case of CRC, it is essential to assess the depth of invasiveness in order to guide either endoscopic or surgical removal. Current endoscopic assessment of depth of invasiveness consists of morphologic examination, NBI and if indicated, magnifying or near focus NBI and EUS. However, endoscopic assessment is operator dependent and some tumors are hard or impossible to image by EUS. Studies have been conducted on CAD models assessing invasiveness using confocal laser endomicroscopy and stained endocytoscopy . Ito and colleagues developed a CNN to objectify endoscopic diagnosis by distinguishing between no–or superficial invasion (cTis or cT1a) and deep submucosal invasion (cT1b). Using WL images of 41 lesions, the CNN achieved a diagnostic accuracy of 81.2%. If we compare this accuracy to that of endoscopists (59%-84%) and EUS (80%-90%) , DL-based diagnostic support systems might be a useful tool in future determination of tumor invasiveness.
3.5
AI in video-capsule endoscopy
Video-capsule endoscopy (VCE) has revolutionized the ability to detect and localize several conditions in the small bowel. However, analysis of VCE images is a time-consuming process since the mean number of obtained images by VCE is >50.000. Although some manually designed, computerized tools have been utilized to decrease the burden of reading, they remained only moderate [ , ]. For these reasons, AI-assisted VCE analysis have been investigated in detecting various conditions as GI bleeding, polyps, cancer, ulcers, erosions, motility disorders, and hookworms . Leenhardt et al reported a sensitivity of 100% and specificity of 96% for their DL algorithm trained on detecting gastrointestinal angioectasias, when compared to a group of VCE experts. The mean reading time for a single image was 46.8 milliseconds, which would result in an estimated 39 minutes to interpret a full VCE video of 50.000 images. Although this study showed excellent performance in diagnosing typical gastrointestinal angioectasias, a clinical applicable device should also detect other causes of GI bleeding. Another promising study investigated a DL-CNN that could detect and localize GI anomalies in general using VCE images . A weakly supervised CNN was trained on detecting any anomaly (vascular, polypoid, or inflammatory) present on the images. Results showed an accuracy of 96.3% and 77.5% in the training phase (344 images). Further development of comparable algorithms might be a huge benefit in highlighting only those images in which review by a physician is necessary.
3.6
Artificial intelligence in upper endoscopy
To date, studies reporting on AI-assisted esophagogastroduodenoscopy (EGD) focus on 4 conditions, namely (a) neoplasia in Barret’s esophagus; (b) esophageal squamous cell carcinoma; (c) gastric cancer (GC), and (d) Helicobacter pylori gastritis.
3.7
Barret’s esophagus
Barret’s esophagus (BE) is a well-described risk factor of developing esophageal adenocarcinoma (EAC). Regular surveillance with endoscopy and biopsy of the BE lesion is indicated to enable early detection of neoplasia and subsequent endoscopic treatment. AI-assisted endoscopy is of special interest in this field because early neoplasia is, out of experts’ hands, often missed during surveillance . In 2016, van der Sommen et al. published the results of their CAD model for early neoplasia in BE, using a SVM discriminating based on color and texture features. Recently, this algorithm was improved , whereupon the algorithm was able to detect more subtle neoplasia lesions and it performed accurate in 91.7% of the images, with a sensitivity of 95% and specificity of 85%. The same study group initiated a consortium (ARGOS) with 4 expert BE centers that will further expand and improve the algorithm with DL techniques in order to evaluate BE neoplasia in real-time endoscopy in the future . Additionally, 2 studies [ , ] developed a CAD system for the detection of early Barret’s neoplasia using endoscopic optical coherence tomography.
3.8
Esophageal squamous cell carcinoma
Esophageal squamous cell carcinoma (ESCC) is still the most common type of esophageal cancer in Asia. ESCC is difficult to diagnose during conventional endoscopy and is often diagnosed in advanced stages, resulting in a poor prognosis. As for our knowledge, 4 studies investigated computerized diagnosis of ESCC during endoscopy and only 1 of them used conventional WL endoscopy . This study trained their supervised DL-CNN on both WL and NBI images of predominantly ESCC, but also a few cases of EAC . Evaluation of the algorithm was performed on a separate dataset of 1118 images from 41 ESCC lesions and 8 EAC lesions. The per-case analysis showed a nearly 100% accuracy, yet the per-image analysis showed a sensitivity of 77%, specificity of 79%, PPV of 39%, and NPV of 95%. Interestingly, the CNN required only 27 seconds for analyzing all of the 1118 images.
3.9
Gastric cancer
Early detection of GC is important to improve survival, since 5-year survival rate drops from >90% when diagnosed and treated in an early stage, to less than 20% in an advanced stage . Although EGD is the procedure of choice to detect GC, lesions are easily overlooked due to subtle morphologic changes . A meta-analysis from Menon and colleagues showed that 11.3% of the GC cases are missed during EGD . Several studies have been published on AI-assisted detection of GC , delineation of cancerous lesions [ , ], and assessment of depth invasiveness [ , ]. Hirasawa et al trained a DL-CNN on 13.584 clear images of 2639 histologically proven GCs (WL, NBI, and chromoendoscopy images). The algorithm was evaluated on 2269 gastric images, including both early and advanced GC lesions. In the per-case analysis, the algorithm correctly detected 71 of 77 cases with a sensitivity of 92.2% and PPV of 30.6%. The authors noted that all missed lesions were superficially depressed intramucosal cancers. The false positive lesions were predominantly images of gastritis with changes in color or irregular mucosa. One study group developed a DL algorithm to classify endoscopic WL images into normal gastric mucosa, ulcer, and cancer . The model could distinguish between normal mucosa and gastric ulcer/cancer with >90% accuracy and between gastric ulcer and cancer with an accuracy of 77.1%.
3.10
Helicobacter pylori gastritis
H. pylori infection could lead to chronic gastritis, ulcers, mucosal atrophy, intestinal metaplasia and is, by doing so, strongly related to GC . CAD of H. pylori gastritis is proposed to be a useful asset in improving the diagnostic accuracy of conventional EGD. Although screening endoscopy for H. pylori gastritis is in most countries screening not indicated, given the high accuracy of less invasive tests, it still is in certain eastern and developing countries. Three studies were published using DL models to detect H. pylori gastritis and the diagnostic accuracy of the models was comparable with that of experienced endoscopists .
3.11
Future potential of AI-assisted endoscopy
As showed in this review, AI-assisted endoscopy is a rapidly and strongly evolving field. Recent studies showed encouraging results of these technologies when applied on still endoscopy images and videos. Still, high-definition and clear pictures are often used in these studies leading to selection bias and probably overestimation of the accuracy.
Although initial important steps along the AI-road have been taken by initiating the first prospective studies on AI-assisted endoscopy, several problems related to AI-algorithms are needed to be addressed before implementation in our practice. In future research, it is important to define and unify our definitions of outcomes to correctly compare them with each other. Where some studies tested their CAD using several images of 1 polyp and assumed a correct outcome when a single image was correctly identified, other studies defined a positive outcome when >50% of images of the same polyp were recognized. Another certain challenge that needs to be overcome is the risk of overfitting, which occurs when the algorithm is overly customized to training data and not suitable anymore to new datasets. To build and validate a clinical robust and applicable CAD device, large, multicenter, prospective studies are needed, conducted on a proper representation of the target population. Future research should show if currently developed CAD-models are robust enough to be validated on external datasets and eventually to be generally applicable. Furthermore, future research will point out the impact of AI-assistance during endoscopy. How will the endoscopist react to automatic diagnosis during endoscopy? Will they learn from the algorithm or get indolent from a highly accurate algorithm? Finally, the skepticism of some physicians and patients towards DL-assistance, due to its black-box nature, is another barrier to break down. Certain techniques have been developed to identify characteristics and features on which the algorithm makes their predictions, which might lead to a more explainable deep-learning era .
In conclusion, numerous successes of AI-assisted endoscopy have been achieved in past years, especially since recent breakthrough in DL. Despite these successes, there are still several problems that need to be addressed before these applications will be utilized in daily practice. Instead of considering AI models as a substitute for human clinical reasoning, emphasis should be made on the fact that until now, these techniques serve as an enhancement of human intelligence. The algorithms are still predominantly trained on labeled data and thus their achievements are as good as its human annotators. Nonetheless, we are convinced that the future of endoscopy will be revolutionized by AI technology.
Appendix
Supplementary materials
Video 1. Detection of colorectal polyps during real-time colonoscopy using GI Genius (Medtronic, Minneapolis MN, USA). The green bounding box indicates a detected lesion.
Conflicts of interest: Sanne A. Hoogenboom: No conflict of interest. Ulas Bagci: No conflict of interest. Michael B. Wallace: Consultant, Cosmo pharmaceuticals, Virgo Inc. Research grants, Cosmo pharmaceuticals, Medtronic, Fujifilm, Olympus.
References
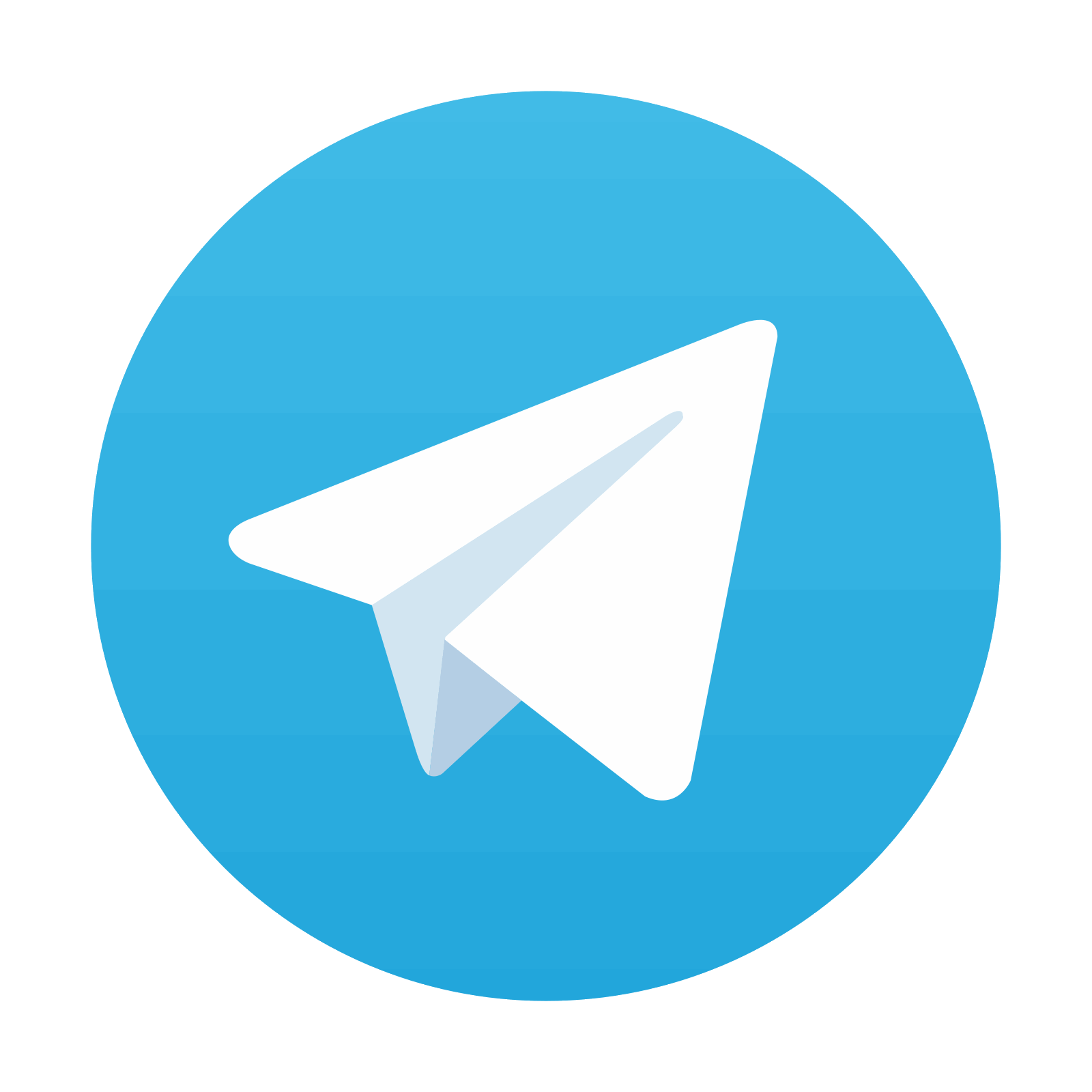
Stay updated, free articles. Join our Telegram channel

Full access? Get Clinical Tree
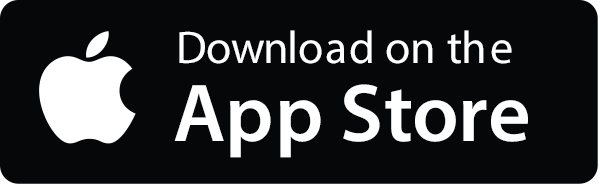

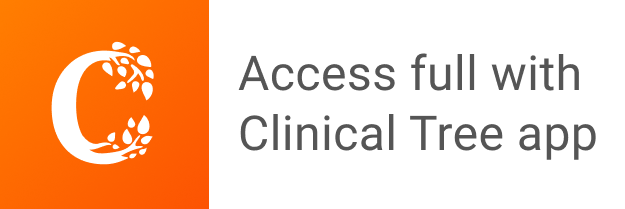