Abstract
Artificial intelligence (AI)-based technologies are developing rapidly, offering great promise for gastroenterology and particularly endoscopy. However, there are complex barriers and pitfalls that must be considered before widespread real-world clinical implementation can occur. This review highlights major ethical concerns related to data privacy and sharing that are essential for the development of AI models, through to practical clinical issues such as potential patient harm, accountability, bias in decisions, and impact on workforce. Finally, current regulatory pathways are discussed, recognizing that these need to evolve to deal with unique new challenges, such as the adaptive and rapidly iterative nature of AI-based technologies, while striking a balance between ensuring patient safety and promoting innovation.
1
Introduction
Artificial intelligence (AI)-based technologies in medicine are being developed at a rapid pace. The subspeciality of gastroenterology is well positioned to benefit from this innovation. This is particularly relevant to the field of endoscopy, where AI technology could address variable performance due to human operator dependence. There have been a number of endoscopy-related AI publications, predominantly in the area of colonoscopy to aid colorectal polyp detection and characterization . Crucially, there are now prospective clinical trials that demonstrate an improvement in endoscopy-related performance [ , ].
Considering the speed at which AI technologies and supporting evidence are evolving, it is likely that deployment in routine clinical practice will become a reality in the near future. The real-world implementation of AI, however, is complex and produces a number of key ethical and regulatory issues. The future success of AI in gastroenterology will rely heavily on our ability to carefully consider and address these challenges. This review provides an overview and explores some potential solutions for these major barriers.
2
Ethical concerns
This section will cover some of the broad ethical issues related to the implementation of AI in gastroenterology commencing with complexities related to data privacy and sharing that are essential for the development of AI models, through to practical issues including potential patient harm, liability, bias in decisions, and impact on the workforce.
3
Data privacy, consent, sharing, and ownership
AI algorithms, particularly those based on deep learning, require large datasets not only for initial training but also for continued validation and fine-tuning or calibration. Furthermore, to demonstrate generalizability of models, it is necessary to collect and share data from different geographic locations. Given the scale at which this must occur, this places issues around data and information governance at the center of debates surrounding AI in gastroenterology .
Digital innovation in healthcare is leading to the generation of an unprecedented quantity of personal patient data. This is leading to increasing challenges for organizations to deal with data privacy concerns where at an individual level, patients may wish to understand who has access to their data, whether it is being used for secondary purposes, the degree to which it has been anonymized and potential commercialization of data. In addition, there are some concerns surrounding unintended consequences of data sharing for AI research such as the downstream discovery of information that may be clinically relevant to future care. Moreover, data breaches could have a dramatic impact on the patient-doctor relationship and even harm an individual patient, for example, discrimination due to a disclosure of a medical diagnosis. It is important, however, to mitigate and balance these risks against the potential wider future benefits to society from AI development.
It is likely that we need to reconsider the traditional models of fully informed consent that may pose challenges for AI development. Data access may be limited to a specific project at the time when consent was provided leaving uncertainty about secondary future use for alternative AI research. This can be frustrating for data scientists where datasets often become more valuable over time by providing insights into longer term outcomes. Moreover, a future request to remove individual data can be difficult once data has already been incorporated into an algorithm. Obtaining individual patient consent can be impractical or even impossible to obtain on the scale that is required for meaningful AI research, whereas limiting training data to only those who specifically consent could inadvertently introduce bias into AI algorithms through the generation of unrepresentative datasets. In the world of gastroenterology, a large number of endoscopic procedures are undertaken daily, yet few of these have consent for video storage and reuse. Ideally, datasets with a vast number of endoscopic videos are needed to create and test AI models, and better consent procedures are needed to manage this issue.
Approaches to this problem include adopting a potential “broad consent” type of policy, where patients may consent to secondary uses of healthcare data without explicit knowledge of all future projects, with the assurances that data are only used in a responsible and appropriate manner that is protected by a data custodian, for example, in a fully anonymized format where there is deemed to be a wider benefit to society.
In some jurisdictions, fully anonymized data are not considered to be personal data; therefore, explicit consent is not required for data sharing and secondary use. However, some concerns do exist regarding the ability to truly achieve full anonymization, particularly for smaller cohorts involving rare diseases as an example. Despite this, many groups have invested considerable efforts in creating collaborative anonymized databases or biobanks to accelerate AI innovation. Furthermore, such datasets could serve as benchmarks for particular clinical problems, allowing for external validation of an algorithm. Additional efforts are on-going in devising standards for data privacy, storage, access, and security at the international level.
While the efforts to create large datasets are being encouraged, the initiatives are often limited by the infrastructure required for data sharing and secure transfers. Many groups, particularly commercial groups, are adopting cloud computing-based solutions to store data and run AI models. However, the financial incentives for healthcare institutions to invest in such infrastructure are often lacking and this may hinder progress. A major barrier is the inherent risk of data breaches, leading healthcare institutions to be cautious about adopting innovative solutions. More recent computer science initiatives such as the use of secure software containers or federated learning attempt to reduce risks by overcoming the need for data to be released .
The development of successful AI will require significant resources and investment. Deployed AI clinical systems will need auditing, maintenance, and updating both from a hardware and software perspective. It is likely that translational success at this early stage will depend upon collaborations between governments, academia, and industry. Data ownership and commoditization is becoming a critical issue, given that access to well-curated and labeled datasets are fundamental for training of clinically relevant AI models. Ownership of the data is a complex ethical issue, with multiple stakeholders involved including patients, healthcare providers, society, government, and AI developers (academic or industrial). Data custodians may charge for transferring data, which often can be limited directly to the recovery of costs for anonymization and secure transfer. There are some suggestions that charges could exceed this, effectively commoditizing data, as long as the profits are reinvested to provide healthcare benefits and patient confidentiality is maintained. Alternatively, some advocate that data access can be provided to AI developers in return for rights to use the technology created. However, it can be argued that much of the value generation occurs when raw data are preprocessed and labeled, an arduous and expensive task often performed by the AI developer. For example, a single endoscopy procedure video may contain thousands of individual frames that need to be carefully manually reviewed and annotated by a human reader.
Without commercial partners, it may not be possible, at least initially, to develop AI software at scale for clinical use. The code and design used in academic research projects seldom meet the rigorous quality required for a medical device. For regulatory purposes, this usually requires a quality management system to be in place from the outset. At this moment in time, the expertise required and associated costs almost invariably result in partnerships with industry to achieve clinical translation.
A new data ecosystem will need to be created, not only with improved quality of data capture but also mechanisms for secure data sharing and linkage for longitudinal outcomes, to truly harness the full potential of AI in healthcare. The future success of AI will depend upon consistent data policies that instill public confidence and trust without being excessively prohibitive against sharing for purposes of wider societal benefit.
4
Patient safety and accountability
There is great promise that AI will ultimately provide safer and better care for patients. Proponents of AI technology argue that it could reduce unwarranted variation and raise quality standards of all endoscopists to those of the best, for example, by improving adenoma detection rates during colonoscopy. Equally, algorithms providing decision support could promote best clinical practice by recommending tests and treatments based on the latest evidence and guidelines. A strong case can be made for an environment where AI works synergistically to augment the performance of human health professionals. This could result in tasks being performed with greater speed, accuracy, and consistency, while also allowing human resources to be directed toward more complex clinical issues. Additional opportunities may arise, for example, in the field of endoscopic imaging, with the discovery of AI-related optical biomarkers that we are currently unaware of or insensitive to as human observers.
Conversely, there is the risk that AI could result in significant patient harm at scale if not developed and evaluated rigorously before being deployed. AI is not infallible, and errors can occur. For example, an AI model designed to predict which patients with pneumonia could be discharged, incorrectly learnt that those with asthma had a lower risk of mortality. This was reflected in the training data used, where patients with pneumonia and asthma were commonly admitted to intensive treatment units, and as a result received more aggressive care that effectively lowered their risk of death from pneumonia . The inability of algorithms to take contextual information into account demonstrates that clinician judgment is still important. Furthermore, algorithmic errors could be compounded by the phenomenon of automation bias, where clinicians may favor AI decisions even if they are incorrect . As AI decision support becomes more widespread, algorithms may even become targets for malicious cyberattacks. Deep-learning-based systems may be susceptible to adversarial attacks, where inputs to models are specifically crafted to force the model to make classification errors . This could be leveraged to manipulate systems to cause deliberate patient harm or for fraudulent purposes related to reimbursement.
Accountability for medical decisions involving AI is an area of debate. If harm occurs who should be held responsible? Should it be the clinician, the healthcare institution, the algorithm developer, the vendor creating the platform for deployment, or the organization who provided the training data? Clearly, one has to consider each individual case and the degree of AI automation involved. A distinction also has to be made between improper use of the device and faults attributed to incorrect outputs from algorithms.
Some experts have adapted the Society of Automotive Engineers (SAE) classification for self-driving cars to identify levels of autonomy in healthcare [ , ]. Here, 5 levels of autonomy are described, where level 5 indicates full autonomy with no requirement for human intervention. It should be noted that the majority of AI systems are currently being designed to provide decision support where clinicians are still expected to provide oversight of algorithmic interpretations. The use of endoscopy decision support software that provides the clinician real-time assistance to detect and classify lesions is a good example of a level 2 application. In reality, it has been suggested that level 3 (conditional automation) is the maximum equivalent that will be implemented clinically. Here, the AI system may complete specific tasks with the expectation that a clinician will intervene in certain scenarios. An example may be large-scale reading of capsule endoscopy reports primarily by AI, with human observers only intervening when results are positive or indeterminate. It should be appreciated that as higher levels of autonomy are attained possibly in the future, the burden of responsibility may shift toward the stakeholders involved in AI development and deployment.
As we move toward an implementation phase, it is crucial that assurances are provided for algorithm efficacy and safety with relevant limitations and warnings highlighted. Moreover, efforts should focus on incorporating AI into the existing clinical environment and culture that facilitates learning from errors. If careful steps are taken at this early development stage, it is likely that AI can be harnessed to ultimately improve patient safety. To this end, engagement with clinical users and patients is key during the development of any AI solution to ensure that potential risks are spotted early before they become embedded in the delivery of care.
5
Transparency and bias
Transparency is an important concept that relate is applicable not only to the methods and data used to develop the algorithm but also interpretation of decisions or outcomes reached by the AI model.
The “black-box” nature of many algorithms, particularly those using deep-learning-based approaches, is a common area of concern. Some methods are being developed to create “explainable AI”; examples include techniques that help gain insight into the function of intermediate layers of deep neural networks and demonstrate what the network is perceiving to inform decisions . The European Union (EU) has introduced the General Data Protection and Regulation, which through article 22, “automated decision-making, including profiling,” states that data subjects should be provided with further information including meaningful information about the logic involved in the decision-making process . It is unclear exactly how this may relate to AI algorithms in practice, but if a right to an explanation or interpretation is required then this could have a significant impact on AI in healthcare. While it is understandable that such transparency might help build trust in clinicians and patients, there is a risk that mandating this in algorithms could stifle progression and also lead to the selection of models that may not necessarily provide the best performance.
The data used to train algorithms can contain inherent biases and may poorly represent the wider population. As a result, this could be reinforced into algorithms deployed for clinical use and potentially lead to discrimination. This may be important in healthcare where individuals who are typically under-represented in research or those with rare conditions may not be included in training datasets . Furthermore, hidden biases could be incorporated inadvertently into datasets, for instance, the selection of a particular drug or manufacturer. Transparency in both the dataset and the interpretability of the algorithm are, therefore, important. Moreover, many researchers are optimistic that the use of AI itself could compensate for identified biases and ultimately help overcome human prejudice.
6
Impact on clinical workforce
Despite being in its infancy, AI in healthcare could dramatically change the future role of clinicians and their relationships with patients. While it is difficult to fully anticipate the potential disruptive effects of AI, it is clear that our workforce needs to be educated and prepared to critically appraise and work alongside such technology. Ideally, future medical curricula should be updated to include a basic understanding of AI methodology and limitations. Specific training modules may need to be implemented in the endoscopy suite. Mechanisms need to be developed for clinicians to register their uncertainty or disagreement with AI-based outputs. This may involve including AI-based recommendations as part of the medical record. For example, it is not inconceivable that images with AI-based interpretations for endoscopic lesion characterization could be presented in complex multidisciplinary meetings to help inform future treatment. This would occur alongside a global assessment of the patient which still requires human physician experience.
AI-based software already demonstrates high performance particularly in visual tasks, such as colorectal polyp detection. It has been suggested that such assistance in the endoscopy room could lead to over-reliance and de-skilling of future endoscopists. Conversely, there is a strong argument that the technology will act as an adjunct, which will improve human cognitive pattern recognition and serve as a valuable educational tool particularly for nonexpert operators . This may ultimately drive up quality and if this is supported by clinical outcomes, then efforts should focus on widespread integration of AI to avoid inequalities among healthcare providers. There is a risk that a two-tier health system could emerge where more AI-enabled healthcare organizations provide superior patient care. For this reason, approaches need to be sought to ensure the widest take up of proven AI technologies.
It is not uncommon for healthcare professionals to feel threatened by the emergence of AI with a perception that their autonomy may be challenged. This is unlikely to be a major issue currently, where the majority of systems are being developed as clinical support tools with physician oversight. However, the clinician-patient relationship could be affected particularly if the physician and AI algorithm disagree, potentially leading to diminishing patient trust and loss of confidence in clinicians. Alternatively, the implementation of AI technology could improve the clinician-patient relationship, due to the “gift of time,” where the machine automation of routine clinical tasks could allow healthcare professionals to invest more time to directly interact with patients .
While the true impact on the clinical workforce may not become apparent until implementation starts occurring at scale, it is important that organizations and policymakers start considering how healthcare staff can become positively AI enabled. A leading example is the Topol Review, “preparing the healthcare workforce to deliver a digital future,” which was commissioned by the UK Secretary of State for Health, making a number of key recommendations relating to AI technology .
7
Regulatory environment
The traditional pathways for medical device regulation (MDR) are not well designed for the rapid cycles of iterative modification for software-based devices. AI-based technology can present unique challenges given its potential to adapt and continuously learn in real time. Regulatory pathways differ globally, and this review will focus on the US and EU perspectives. However, it should be noted that a voluntary group known as the International Medical Device Regulators Forum is attempting to develop harmonized principles and common regulatory frameworks for software as a medical device (SaMD).
The Food and Drug Administration (FDA) in the United States categorizes devices into 3 classes according to risk. Class III constitutes the highest risk requiring a greater degree of regulatory oversight to provide assurances of safety and effectiveness. Manufacturers are required to submit a marketing application based on risk (510(K) notification, de novo, or premarket approval pathway). The process can be complex and lengthy with further review required for proposed modifications to the device. To address this, the FDA is piloting a software precertification program, as highlighted in the Digital Innovation Action Plan, which is designed to provide a more streamlined and efficient pathway for SaMD . This novel pathway places an emphasis on the technology developer rather than focusing on the product. Organizations will be appraised for excellence based on 5 principles: patient safety, product quality, clinical responsibility, cybersecurity, and proactive culture. Those achieving organizational excellence may be exempt from premarket review for low-risk devices or benefit from a streamlined process for higher risk technology on the basis of the International Medical Device Regulators Forum risk categorization. The organization would need to be able monitor postmarketing real-world performance to verify the continued safety and effectiveness of the device.
A more recent FDA whitepaper also proposes a new regulatory framework for modifications to AI-based software as a medical device . A total product lifecycle regulatory approach incorporates the iterative improvement process that AI algorithms may use. This highlights the commonly neglected issue that AI-based software can function on a spectrum from being locked to continuously learning. Three categories of modification have been identified following initial approval of the SaMD: changes in performance, inputs, and intended use. Although there is no formal approved guidance yet, it is possible that some modifications may only require documentation, while others mandate a new submission or approval, for example, if the intended use or risk categorization changes.
The EU regulatory approach involves processes that ensure products meet the requirements for the stated intended use. The new EU MDR came into force in 2017, with a transition deadline of May 2020, replacing the existing Medical Device Directive . The new regulation can be applied directly to EU member states without the need for national legislation for implementation . The MDR defines medical devices into classes based on risk, similar to the FDA but with the additional subcategories of Classes IIa and IIb within the medium risk category. The class determines the subsequent conformity assessment route, requiring the involvement of an independent notified body in most cases.
The new MDR includes some key changes such as increased scrutiny and demand on notified bodies, reclassification of devices to a higher risk, an emphasis on postmarket surveillance, traceability of devices, and more rigorous evidence requirements for class III and implantable devices. Unlike the FDA, there has been no formal publication of the EU regulatory approach or view on AI. The MDR introduces a new classification rule 11, related to software providing information used to take decisions with diagnosis or therapeutic purposes, which broadly fall into Class IIa devices . Exceptions relate to scenarios where such decisions have an impact that may cause irreversible deterioration of a person’s state of health or serious deterioration in health or a surgical intervention, where categorization will be Class III or IIb, respectively. The interpretation of this rule is challenging, with some predicting that it may lead to excessively stringent classification of software into higher risk categories, potentially hindering AI-related innovation.
A number of AI algorithms for image analysis have been approved by regulatory bodies, although these are predominantly in the fields of radiology and ophthalmology. Of note, a diagnostic system for diabetic retinopathy (IDx-DR) became the first fully autonomous AI-based software to be approved by the FDA in United States . Only a few gastroenterology-related AI systems have been approved internationally, such as EndoBRAIN® which is a computer-aided diagnostic system for predicting colorectal polyp pathology based on in vivo microscopic imaging obtained from an endocytoscope . This was recently approved by Japan’s Pharmaceuticals and Medical Devices Agency as a Class III device.
Given the impressive speed at which endoscopy-related AI software is being developed, it is highly likely that we will see more products on the market in the near future. Crucially, it is clear that the regulatory pathways for AI are currently evolving and the associated uncertainty around requirements may be delaying the typical rapid pace of technological development observed in other sectors. This currently represents a major barrier to progression. However, in healthcare, a balance must be achieved between protecting patients and promoting innovation. Perhaps greater collaboration between regulators, AI developers, clinicians, and patients may lead to more pragmatic pathways, ideally using international frameworks that deal better with the dynamic nature of AI software.
8
Conclusions
AI-based technologies offer great promise to revolutionize gastroenterology. The earliest translation will undoubtedly occur in endoscopy owing to advances in computer vision techniques particularly deep-learning-based approaches. The implementation into real-world clinical practice, however, involves overcoming some major barriers related to ethical and regulatory issues. It is going to be crucial to gain the trust and confidence of patients and clinicians. This involves undertaking rigorous clinical studies, to evaluate not only efficacy but also safety and impact on workflow. Building mechanisms to facilitate machine interpretability and clear policies on accountability will encourage adoption. To truly harness the power of AI, investment is required to develop the infrastructure to encourage data sharing with consistent information governance policies that promote innovation while safeguarding patient confidentiality. We also need to lay the foundations to start preparing and educating the workforce for a future AI-enabled healthcare system. It is likely that dedicated AI committees are required within gastroenterology and endoscopy, perhaps at an international level, to start identifying solutions to overcome barriers. Finally, regulatory policies urgently need to be updated and clarified to cope with the unique challenges that AI technologies pose so that devices can be evaluated and translated more efficiently.
This work was supported by the National Institute for Health Research University College London Hospitals Biomedical Research Centre. This work was also supported by the CRUK Experimental Cancer Medicine Centre at UCL and the Wellcome/EPSRC Centre for Interventional and Surgical Sciences (WEISS) at UCL (203145Z/16/Z).
Conflicts of interest: Omer F. Ahmad: None. Danail Stoyanov: Shareholder Odin Vision and Digital Surgery Ltd. Laurence B. Lovat: Minor shareholder in Odin Vision. Research grants from Medtronic, Pentax Medical, and DynamX. Scientific Advisory Boards: Dynamx, Odin Vision, and NinePoint Medical.
References
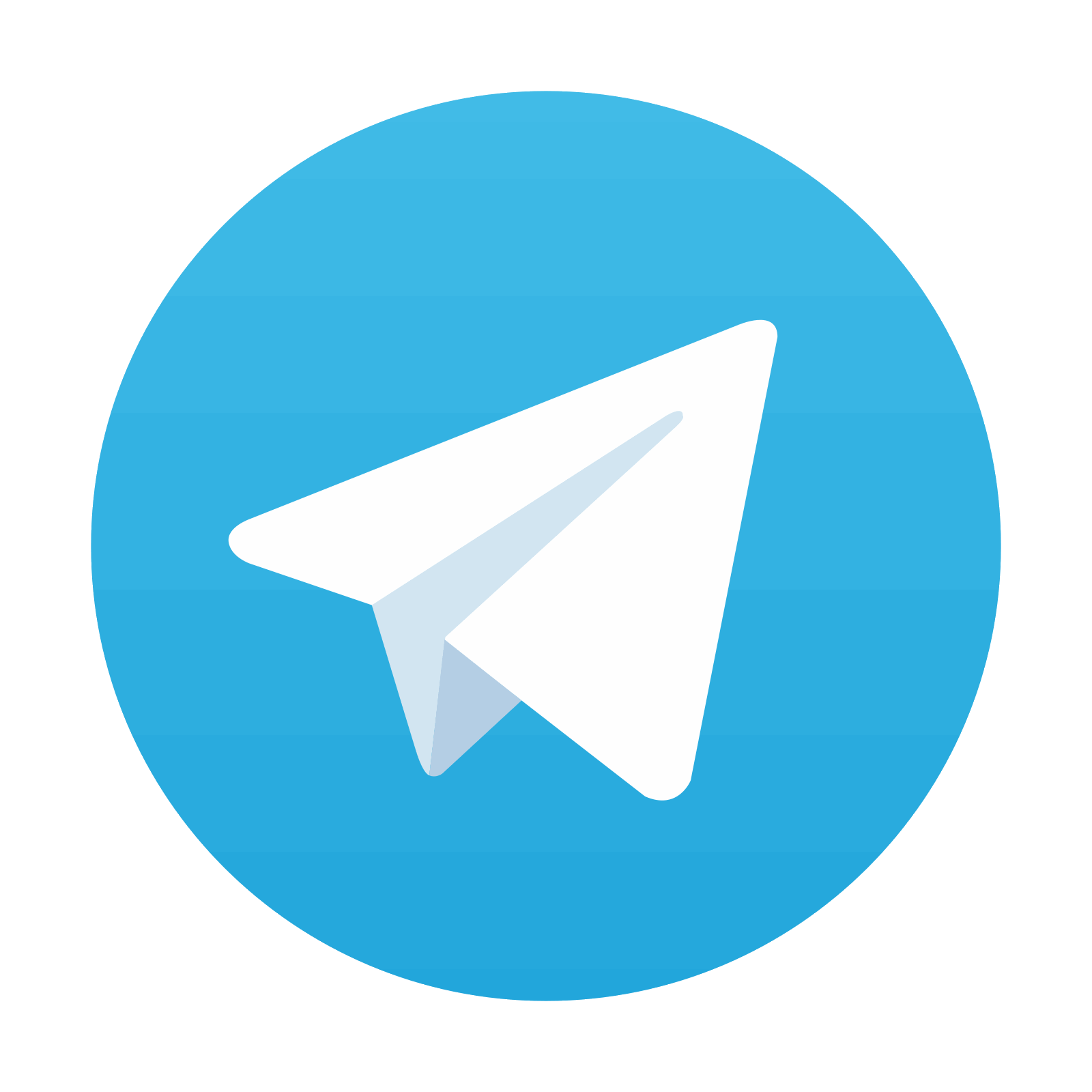
Stay updated, free articles. Join our Telegram channel

Full access? Get Clinical Tree
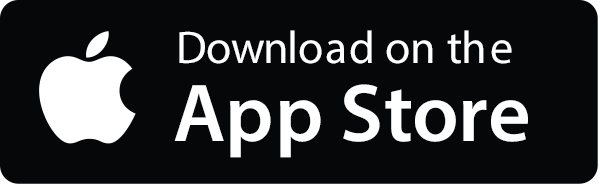

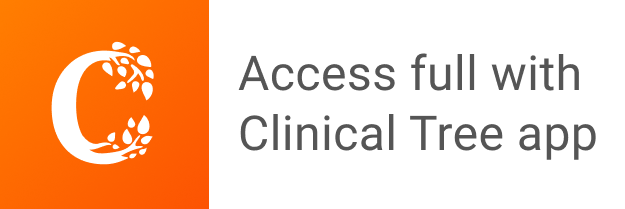