In light of the overdiagnosis and overtreatment associated with widespread prostate-specific antigen–based screening, controversy persists surrounding the detection and diagnosis of prostate cancer (PCa). Given its anatomic proximity to the prostate, urine has been proposed as a noninvasive substrate for prostatic biomarkers. With greater understanding of the molecular pathways of carcinogenesis and significant technological advances, the breadth of potential biomarkers is substantial. In this review, the authors aim to provide an evidence-based assessment of current and emerging urinary biomarkers used in the detection and prognostication of PCa and high-grade PCa, with particular attention on clinically relevant findings.
Key points
- •
Prostate cancer antigen 3 scores less than 20 seem to reliably rule out the presence of prostate cancer and particularly higher-risk prostate cancer on repeat biopsy; complementary RNA-based markers, such as TMPRSS2:ERG, and DNA-based markers, such as GSTP1, may improve its predictive ability and require additional study.
- •
In addition to traditional RNA, DNA, and protein-based biomarkers, emerging areas of study include urinary microRNA, long noncoding RNA, metabolomics, exosomes, and microbiota.
- •
Optimal diagnostic ability is generally obtained when novel biomarkers are added to multivariable models, including clinical factors, such as age, prostate-specific antigen, digital rectal examination, and prostate volume.
- •
Studies comparing urinary biomarkers with other promising diagnostic tools, such as the Prostate Health Index and multi-parametric MRI, are limited but will be necessary to optimize accurate and efficient disease detection.
- •
Future biomarker studies should consistently report the rate of biopsy avoidance with marker use, rate of undiagnosed cancers if biopsy omitted, performance associated with specific threshold values, marker utility in multivariable models, and marker utility in diagnosing high-grade cancers.
Introduction
Significant technological advances in analytical methods and a greater understanding of molecular carcinogenesis has paved the way toward a new era of disease detection. Potential biomarkers of human disease range from whole-cell analysis to characterization of cell-free components, such as proteins and nucleic acids. In addition to traditional serum or plasma, urine has been proposed as an easily obtained substrate for prostatic biomarkers. To date, several urinary biomarkers have been identified and considered for use in prostate cancer (PCa), each with varying levels of evidence. In the subsequent review, the authors’ primary aim is to assess the evidence basis and potential applications of urinary biomarkers for PCa.
Urine as a Substrate
Before considering the multitude of molecular isolation and quantification techniques, successful urine-based screening largely depends on the (1) the shedding of PCa cells or their components into urine and (2) successful acquisition, processing, and preservation of urine. The technical aspects of such methodologies have been previously reviewed in significant detail. Initial questions considering the type (single void vs 24 hour) and timing (first catch vs midstream) of specimen collection have come out largely in favor of single-void, first-catch sampling. From a clinical perspective, evidence supports collection of urine after prostatic manipulation (eg, digital rectal examination [DRE]) to optimize assay yield, and collection after transrectal ultrasonography (TRUS)–guided biopsy has proven feasible as well. The authors have herein considered potential urinary markers of PCa with attention to clinically relevant factors impacting their use.
Introduction
Significant technological advances in analytical methods and a greater understanding of molecular carcinogenesis has paved the way toward a new era of disease detection. Potential biomarkers of human disease range from whole-cell analysis to characterization of cell-free components, such as proteins and nucleic acids. In addition to traditional serum or plasma, urine has been proposed as an easily obtained substrate for prostatic biomarkers. To date, several urinary biomarkers have been identified and considered for use in prostate cancer (PCa), each with varying levels of evidence. In the subsequent review, the authors’ primary aim is to assess the evidence basis and potential applications of urinary biomarkers for PCa.
Urine as a Substrate
Before considering the multitude of molecular isolation and quantification techniques, successful urine-based screening largely depends on the (1) the shedding of PCa cells or their components into urine and (2) successful acquisition, processing, and preservation of urine. The technical aspects of such methodologies have been previously reviewed in significant detail. Initial questions considering the type (single void vs 24 hour) and timing (first catch vs midstream) of specimen collection have come out largely in favor of single-void, first-catch sampling. From a clinical perspective, evidence supports collection of urine after prostatic manipulation (eg, digital rectal examination [DRE]) to optimize assay yield, and collection after transrectal ultrasonography (TRUS)–guided biopsy has proven feasible as well. The authors have herein considered potential urinary markers of PCa with attention to clinically relevant factors impacting their use.
RNA-based markers
Prostate Cancer Antigen 3
From bench to bedside
In 1999, Bussemakers and colleagues first described Differential Display Code 3 as a potential urinary biomarker for PCa. Based on differential display analysis, the investigators described a messenger RNA that was highly overexpressed in 95% of PCa tissue and absent from benign prostate tissue and other tumor types. Subsequently identified as PCa antigen 3 (PCA3), it was further characterized as a noncoding RNA that was indeed highly specific for PCa. Early quantification with quantitative real-time–polymerase chain reaction (qRT-PCR) demonstrated a 34-fold increased expression in malignant prostate tissue and high discriminative value as demonstrated by an area under the receiver operating characteristic curve (AUC) of 0.98 ( Table 1 includes key definitions in the assessment of diagnostic tests). These findings were subsequently replicated by other investigators, and the introduction of a novel urinary assay to detect PCA3 helped lead its transition to the clinical setting.
Meaning | Equation | Practical Use | Threshold a | |
---|---|---|---|---|
Sn | Ability of a test to correctly identify those who have the disease | TP TP + FN | Emphasize sensitivity when penalty for missing a case is high (eg, disease spreads easily and is fatal but can be successfully treated) | Decrease threshold = test is more sensitive, less specific |
Sp | Ability of a test to correctly identify those who do not have the disease | TN TN + FP | Emphasize specificity when consequence (eg, treatment, additional testing) of positive test is significant (eg, invasive, toxic) | Increase threshold = test is more specific, less sensitive |
PPV b | The proportion of patients who truly do have the disease out of all who test positive | TP TP + FP | If a person tests positive, what is the probability that he or she does have the disease? | –– |
NPV b | The proportion of patients who truly do not have the disease out of all who test negative | TN TN + FN | If a person tests negative, what is the probability that he or she does not have the disease? | –– |
AUC a | The probability the test score of a randomly selected diseased subject will be greater than that of a randomly selected nondiseased subject | –– | Quantifies the diagnostic performance of a test in terms of sensitivity and specificity independent of a specific threshold value | –– |
a Assuming higher/increased values are positive test results.
b Predictive values (ie, positive predictive value, negative predictive value) are not fixed characteristics of a test; they depend on the disease prevalence in the tested population.
In 2006, a functional platform for clinical use was introduced as the Progensa PCA3 assay. Urine specimens were obtained after attentive DRE (3 strokes to each prostate lobe), and PCA3 was quantified based on transcription-mediated amplification and hybridization. Using prostate-specific antigen (PSA) transcripts to control for RNA quality and confirm the presence of nuclear material, PCA3 scores were generated as a simple PCA3 to PSA ratio. Promising data emerged suggesting that PCA3 may be superior to PSA in the diagnostic setting. Deras and colleagues demonstrated univariable AUC of 0.69 for PCA3 as compared with 0.55 for PSA. In a multivariable model with other clinical factors, PCA3 conferred an AUC up to 0.75. Furthermore, PCA3 was shown to be independent of age, prostate volume, and PSA. Follow-up studies also suggested a role for PCA3 in men with previous negative biopsies. In this population, Marks and colleagues reported an AUC of 0.68 for PCA3 as compared with 0.52 for PSA. Importantly, reports of PCA3 have consistently yielded informative rates ranging from 94% to 99%.
Repeat biopsy: diagnosis
Based on these findings and others, PCA3 was approved by the Food and Drug Administration in 2012 for use in men with a previous negative biopsy. Although multiple studies demonstrated improved diagnostic accuracy compared with PSA, the optimal threshold for clinical use was the focus of further investigation. Eleven studies of moderate to high quality were included in a 2014 meta-analysis from Luo and colleagues. The investigators examined performance characteristics using PCA3 threshold values of 20 and 35. Based on a threshold score of 20, sensitivity and specificity measured up to 93% and 64%, respectively. High sensitivity and negative predictive values (NPVs) approaching 90% across multiple populations (ie, predictive values are not inherent to diagnostic tests but also vary with disease prevalence in the study population; see Table 1 ) suggested potential use for excluding PCa in men who tested negative. The performance characteristics of PCA3 across multiple studies using threshold scores of 20 and 35 are listed in Table 2 .
Study, Year | N | Threshold PCA3 = 20 | Threshold PCA3 = 35 | ||||||
---|---|---|---|---|---|---|---|---|---|
Sn (%) | Sp (%) | PPV (%) | NPV (%) | Sn (%) | Sp (%) | PPV (%) | NPV (%) | ||
Wei et al, 2014 | 297 | 76 | 52 | 32 | 88 | 54 | 73 | 36 | 84 |
Pepe & Aragona, 2013 | 100 | 93 | 17 | 30 | 86 | 79 | 24 | 29 | 74 |
Goode et al, 2013 | 167 | –– | –– | –– | –– | 42 | 71 | 16 | 91 |
Wu et al, 2012 | 103 | 67 | 64 | 52 | 78 | 38 | 77 | 50 | 66 |
Pepe et al, 2012 | 118 | 91 | 28 | 32 | 89 | 72 | 42 | 32 | 80 |
Bollito et al, 2012 | 509 | 88 | 44 | 41 | 90 | 75 | 70 | 52 | 87 |
Barbera et al, 2012 | 177 | 92 | 26 | 32 | 90 | 73 | 42 | 35 | 81 |
Auprich et al, 2012 | 127 | 85 | 25 | 38 | 78 | 75 | 58 | 49 | 81 |
Pepe & Aragona, 2011 | 74 | 93 | 21 | 43 | 89 | 70 | 44 | 42 | 72 |
Aubin et al, 2010 | 1072 | 71 | 57 | 26 | 90 | 48 | 79 | 33 | 88 |
Haese et al, 2008 | 463 | 73 | 51 | 36 | 83 | 47 | 72 | 39 | 78 |
Composite range | 67–93 | 17–64 | 26–52 | 78–90 | 38–79 | 24–79 | 16–52 | 66–91 |
Based on their findings, Luo and colleagues concluded that a cutoff score of 20 was superior to 35 in the repeat biopsy setting. As others had observed, the investigators reported that use of PCA3 could reduce unnecessary repeat biopsies by more than 50% in some settings. Similar results were replicated in prospective multicenter cohorts of Italian and American men. In the repeat biopsy cohort of the National Cancer Institute Early Detection Research Network validation trial, Wei and colleagues confirmed that PCA3 scores below a threshold of 20 were associated with a very low rate of high-grade cancers; this finding was not observed, however, in men undergoing initial biopsy. In addition to corroborating previous findings in the repeat biopsy population, the study by Wei and colleagues added to the growing literature aiming to clarify the prognostic role of PCA3.
The contemporary era
Studies of PCA3 since 2012 generally varied from the previous era in 3 ways. First, PCA3 was more frequently assessed in the setting of initial biopsy. At the same time, many contemporary reports considered the presence of high-grade PCa (HGPCa, defined herein as Gleason sum ≥7) in addition to PCa. Finally, additional studies examined PCA3 in the setting of multiple biomarkers as part of a multivariable model or nomogram. In addition to total PSA, other PSA isoforms and permutations have been frequently considered, including percent free PSA (%fPSA = fPSA/total PSA), percent (−2)proenzyme PSA (%percent pro PSA = [(percent pro PSA pg/mL/10)/fPSA ng/mL]), and the Prostate Health Index (PHI = [(percent pro PSA pg/mL/fPSA ng/mL) × (total PSA ng/mL) 1/2 ]).
Initial biopsy: diagnosis and prognosis
In 2013, Scattoni and colleagues found that PCA3 did not improve the predictive accuracy of a baseline multivariable model (BMM) including PSA, %fPSA, and prostate volume (PV) in men presenting for initial biopsy (AUC 0.79 vs 0.80, P = .69). PHI, on the other hand, improved the model by 5%, although statistical significance was not reached (0.79 vs 0.84, P = .144). Ferro and colleagues further explored these markers in men with PSA 2 to 10 ng/mL, reporting AUC values of 0.77, 0.76, and 0.73 for PHI, %p2PSA, and PCA3, respectively. Although PHI did not significantly outperform PCA3 on a pairwise comparison ( P = .247), PHI did outperform PCA3 on decision curve analysis when the threshold probability (ie, the probability of cancer at which the benefit of biopsy and no biopsy are considered equal) exceeded 25%. For example, using a threshold probability of 30%, the use of PHI conferred 21% fewer biopsies without increasing PCa underdetection as compared with only 11% when using PCA3.
Several reports have considered the role of PCA3 in PCa diagnosis and in prognosis through assessment of HGPCa. Hansen and colleagues explored various PCA3 thresholds and noted improvements ranging from 5.0% to 7.1% (all P <.001) compared with the BMM. Similarly, prediction of HGPCa improved by 3.6% to 5.4% (all P <.001). For both outcomes, the PCA3 threshold score of 21 optimized predictive accuracy. Ruffion and colleagues examined PCA3 in conjunction with established nomograms in a large French cohort. The projected rates of biopsies avoided and HGPCa underdiagnosed were as follows: 43% and 6% for the Hansen nomogram, 48% and 6% for the Chun nomogram, and 22% and 3% for the Prostate Cancer Prevention Trial (PCPT) risk calculator (PCPTrc). In the largest such study to date, Chevli and colleagues demonstrated that PCA3 significantly improved the baseline model for both PCa (AUC 0.70 vs 0.75, P <.001) and HGPCa (AUC 0.80 vs 0.81, P = .015). Notable studies of PCA3 in initial biopsy populations are summarized in Table 3 .
N | BMM Variables | BMM | BMM + PCA3 | Bx Avoided (%) | Sn (%) | Sp a (%) | NPV (%) | Optimal Threshold | |
---|---|---|---|---|---|---|---|---|---|
Scattoni et al, 2013 | 116 | PSA, %fPSA, PV | 0.79 | 0.80 | –– | –– | –– | –– | –– |
Ferro et al, 2013 | 300 | Age, PSA, fPSA, DRE, PV | 0.72 | 0.77 | –– | –– | –– | –– | –– |
Hansen et al, 2013 | 692 | Age, PSA, DRE, PV | 0.74 | 0.81 | 23 | 94 | 36 | 87 | 21 |
HGPCa | Age, PSA, DRE, PV | 0.78 | 0.83 | 23 | 98.5 | 36 | 99 | 21 | |
Ruffion et al, 2013 | 594 | Age, PSA, DRE, PV | 0.71 | 0.78 | 13 | 97 | 22 | 92 | 21 |
HGPCa | Age, PSA, DRE, PV | 0.77 | 0.80 | 13 | 99 | 22 | 98 | 21 | |
Chevli et al, 2014 | 3073 | Age, PSA, fPSA, DRE, PV, FH, BMI | 0.70 | 0.75 | –– | –– | –– | –– | –– |
HGPCa | Age, PSA, fPSA, DRE, PV, FH, BMI | 0.80 | 0.81 | –– | –– | –– | –– | –– |
a Specificity is equivalent to the proportion of biopsies avoided among the population that did not have PCa/HGPCa.
Most recently, Seisen and colleagues directly compared PCA3 and PHI in an initial biopsy cohort of 138 men. Although PCA3 significantly outperformed PHI in predicting overall PCa (AUC 0.71 vs 0.65, P = .03), PHI was a superior predictor of significant PCa defined using Epstein criteria (AUC 0.80 vs 0.55, P = .03) and was the lone independent predictor of significant PCa on multivariable analysis. Recognizing the limitations of prostate biopsy, Cantiello and colleagues compared these markers in a radical prostatectomy (RP) cohort. The investigators found that PHI significantly and substantially improved the baseline model ability to predict tumor volume less than 0.5 mL and seminal vesicle invasion compared with PCA3. In men with a biopsy Gleason score of 6 or less, the use of PHI was associated with a 7.6% increase in the ability to predict significant PCa (defined by non–organ-confined cancer, volume ≥0.5 mL, or Gleason pattern ≥4) as compared with the baseline model (97.3% vs 89.7%, P <.05), whereas PCA3 did not improve the model. These findings were corroborated in a recent cohort of 78 men who underwent RP, as PHI was again superior to PCA3 in predicting tumor volume greater than 0.5 mL, Gleason score of 7 or greater, and pathologic T3 disease.
Unique applications
In addition to use in traditional settings, others have tested various adaptations of the PCA3 score. Like PSA density, Siegrist and colleagues investigated the ratio of PCA3 score to PV (ie, PCA3 density [PCA3D]). In 288 men undergoing initial (63.5%) or repeat (36.5%) diagnostic biopsy, they observed AUC of 0.72 for PCA3D as compared with 0.49 for PSA, 0.59 for PSA density (PSAD), and 0.69 for PCA3. In a follow-up study, PCA3D of 1 or greater was associated with a 70% risk of positive biopsy as compared with 29% if PCA3D was 1 or less. Notably, PSAD was superior to PCA3D in predicting HGPCa. Elsewhere, De Luca and colleagues investigated trends in PCA3 over time; over a median period of 16 months, intraindividual PCA3 scores were stable relative to threshold values (eg, 20, 35, and 50) in 80% of men. In a subsequent study of 108 men in which repeat PCA3 either upgraded (ie, increased from below a threshold to above it) or downgraded (ie, decreased from above a threshold to below it), they observed upgrading in 83% of men found to have PCa. As in other settings, however, the ease of clinical use was limited by the absence of a robust threshold value. Finally, among men with high-grade prostatic intraepithelial neoplasia, PCA3 had limited application individually but demonstrated increased utility in multiplex models considering additional markers.
Others have studied the impact of multi-parametric MRI (mpMRI) on PCA3 and other biomarkers. In 2013, Leyten and colleagues studied 290 men who underwent PCA3 testing and biopsy, including 115 men who also underwent mpMRI. The investigators reported that PCA3 predicted biopsy outcome based on the detection of PCa in 55% of men with a PCA3 of 35 or greater compared with 23% of men with a PCA3 less than 35 ( P <.001). Assessment of the 115 men exposed to all 3 tests, however, suggests that mpMRI was vastly superior to PCA3 ( Table 4 ). Porpiglia and colleagues examined PCA3, PHI, and MRI in 170 men with a history of negative biopsy. They found that PCA3 and PHI were independent predictors of PCa only when mpMRI was not considered. In the multivariable model including all 3 tests, mpMRI was the only significant predictor of PCa (odds ratio 98.1, P <.001). This study used binary (ie, suspicious vs nonsuspicious) MRI reporting from a single expert radiologist as opposed to a standardized reporting system, such as the Prostate Imaging and Data System (PI-RADS) or PI-RADS2. Therefore, additional study will be necessary to determine the reproducibility of their findings.
Sn (%) | Sp (%) | PPV (%) | NPV (%) | |
---|---|---|---|---|
PCA3 | 69 | 46 | 74 | 39 |
mpMRI | 95 | 49 | 81 | 81 |
Active surveillance
There are limited data exploring emerging biomarkers in active surveillance (AS) populations. One preliminary study measured PCA3 in 294 men with very-low-risk disease. In this cohort, PCA3 was obtained at a median of 2.5 years after diagnosis and subjects were followed for a median of 3.7 years from diagnosis and underwent yearly biopsy. PCA3 showed a nonsignificant trend toward being increased in the 38 (13%) men who were reclassified during follow-up (mean 50.8 in nonreclassified vs 60.0 in reclassified, P = .131). In a limited number of subjects, it is notable that the PCA3 scores were higher in men who underwent grade reclassification (ie, Gleason score upgrading) as compared with those who underwent volume reclassification (mean 72.0 vs 51.2, respectively; P = .174) or no reclassification (mean 72.0 vs 50.8; P = .304) during follow-up. The relationship of PCA3 with tumor multifocality has obvious applications to the AS setting as well. The limited data available underscore the need for additional research.
Prostate cancer antigen 3: summary
Although PCA3 has demonstrated incremental superiority to PSA in many settings, in-depth analyses have concluded there is insufficient evidence of improved health outcomes associated with its use. Other limitations include the need for DRE before collection and the observation that, although based on very limited data, PCA3 may be less informative than other tests, such as PHI and mpMRI. Regardless, the marker is making a strong case for selective use in specific clinical settings; but what are those settings, and what is the risk of its use?
The strength of PCA3 lies in the rarity of false-negative tests when a low threshold value, such as 20, is used. At this threshold, as evident in Table 2 , the test is highly sensitive and carries a high negative predictive value in most populations. To patients and providers, this means that if a PCA3 score is less than 20, the possibility that a cancer exists is low, ranging from 10% to just more than 20% in the repeat biopsy population. In the setting of multivariable models with clinical factors, these risks become even smaller. Perhaps most importantly, the risk of missing high-grade cancer seems to be even lower. Which values are low enough to avoid or delay biopsy is of course a shared decision to be made in the context of each patient’s overall health and personal preferences. The accumulation of data from additional populations will help to characterize these risks and further clarify the potential uses of PCA3.
TMPRSS2:ERG
From bench to bedside
Previously described in hematologic malignancies, Tomlins and Colleagues first reported the presence of gene fusions in PCa in 2005. Their landmark report described fusions of the androgen-regulated transmembrane protease, serine 2 (TMPRSS2) gene and members of the E26 transformation specific (ETS) oncogene family, which were strongly associated with marked overexpression of the latter. Of the ETS transcription factors, ERG (v-ets avian erythroblastosis virus E26 oncogene homolog) is regarded as a key PCa oncogene and is located in tandem to TMPRSS2 on chromosome 21. Throughout the literature, TMPRSS2:ERG has been identified in 50% of tumor foci and 75% to 80% of PCa cases, with greater than 99% histologic specificity for PCa. In 2006, a urinary assay for TMPRSS2:ERG fusion transcripts was developed based on the transcription-mediated amplification platform used for PCA3 testing. An initial study of this assay demonstrated 37% sensitivity, 93% specificity, and 94% positive predictive value (PPV) for PCa. As there exists heterogeneity of TMPRSS2:ERG status among intraprostatic tumor foci, Young and colleagues importantly demonstrated that TMPRSS2:ERG scores were strongly correlated to total ERG-positive cancer tissue.
The contemporary era
Given early findings reflecting its high specificity, it was proposed that TMPRSS2:ERG could be most useful in combination with a more sensitive marker, such as PCA3. A baseline assessment can be gained from the work of Salami and colleagues. In 45 men scheduled for biopsy, they found PCA3 was the most sensitive marker (sensitivity = 93%, specificity = 37%), whereas TMPRSS2:ERG (sensitivity = 67%, specificity = 87%) and PSA (using threshold 10.0 ng/mL; sensitivity = 40%, specificity = 93%) were substantially more specific. Ultimately, TMPRSS2:ERG had the highest discriminatory value for diagnosis as compared with PCA3 and PSA (AUC of 0.77, 0.65, and 0.67, respectively). A multivariable model including all 3 markers conferred an AUC of 0.88 (95% confidence interval: 0.75–0.98). In a similar setting, however, Stephan and colleagues fixed sensitivity at 90% and found that PCA3 yielded greater specificity, particularly in the repeat biopsy cohort (PCA3 36%, PHI 19%, TMPRSS2:ERG 4%). The performance characteristics obtained from these studies are listed in Table 5 .
Study, N (Prevalence) | Setting | Variables | AUC PCa | Sn (%) | Sp (%) | PPV | NPV |
---|---|---|---|---|---|---|---|
Salami et al, 2011, 45 (33%) | IBx (53%) RBx (47%) | PSA | 0.67 | 40 | 93 | –– | –– |
PCA3 | 0.65 | 93 | 37 | 42 | 92 | ||
TMPRSS2:ERG | 0.77 | 67 | 87 | 71 | 84 | ||
PSA + PCA3 + T2E | 0.88 | 80 | 90 | 80 | 90 | ||
Stephan et al, 2013, 246 (45%) | IBx (55%) | PCA3 | 0.70 | 90 | 32 | –– | –– |
PHI | 0.68 | 90 | 29 | ||||
TMPRSS2:ERG | 0.68 | 90 | 21 | ||||
RBx (45%) | PCA3 | 0.77 | 90 | 36 | –– | –– | |
PHI | 0.69 | 90 | 19 | ||||
TMPRSS2:ERG | 0.53 | 90 | 4 |
Building on their novel discovery, Tomlins and colleagues showed that TMPRSS2:ERG significantly increased AUC for PCa detection compared with PSA in their academic biopsy cohort (AUC 0.71 vs 0.61). Furthermore, the addition of PCA3 led to further improvement of the TMPRSS2:ERG model (AUC = 0.77). In multivariable models considering the PCPTrc, optimal discriminative ability was obtained in the model including both PCA3 and TMPRSS2:ERG (AUC = 0.79). Several other studies have explored the impact of these markers on multivariable models; these findings are listed in Table 6 . Cornu and colleagues found that PSAD, PCA3, and TMPRSS2:ERG each contributed to the BMM, yielding an AUC of 0.73. In the HGPCa model, the combined model AUC was 0.80. Tallon and colleagues subsequently found that PCA3, TMPRSS2:ERG, and PHI independently predicted a tumor volume of 0.5 mL or greater in men who underwent RP; but PHI was the only test predictive of a Gleason score of 7 or greater.
Study | N (Prevalence) | Setting | Variables | AUC PCa | AUC HGPCa |
---|---|---|---|---|---|
Tomlins et al, 2011 | 606 (44%) | Bx (70% IBx) | PSA | 0.61 | –– |
TMPRSS2:ERG | 0.71 | ||||
PCA3 + T2E | 0.77 | ||||
PCPTrs | 0.64 | ||||
PCPTrs + PCA3 + T2E | 0.79 | ||||
Cornu et al, 2013 | 291 (59%) | Bx (63% IBx) | PSAD | 0.57 | –– |
PCA3 | 0.66 | –– | |||
TMPRSS2:ERG | 0.67 | –– | |||
BMM a + greater | 0.73 | 0.80 | |||
Tallon et al, 2014 | 154 (100%) | Pre-RP | PCA3 | –– | –– |
PHI | 0.66 | ||||
TMPRSS2:ERG | –– | ||||
BMM b | 0.81 | ||||
BMM + PHI | 0.86 | ||||
Leyten et al, 2014 | 497 (44%) | Bx (79% IBx) | PCA3 | 0.72 | 0.53 |
TMPRSS2:ERG | 0.59 | 0.64 | |||
ERSPCrc | 0.80 | 0.80 | |||
ERSPCrc + PCA3 | 0.83 | –– | |||
ERSPCrc + T2E | 0.84 | 0.84 | |||
Tomlins et al, 2015 c | 1225 (42%) | Bx (80% IBx) | PSA | 0.59 | 0.65 |
PSA + T2E | 0.69 | 0.73 | |||
PSA + PCA3 | 0.73 | 0.75 | |||
PSA + T2E + PCA3 | 0.75 | 0.77 | |||
PCPTrc | 0.64 | 0.71 | |||
PCPTrc + T2E | 0.72 | 0.75 | |||
PCPTrc + PCA3 | 0.74 | 0.75 | |||
PCPTrc + T2E + PCA3 | 0.76 | 0.78 |
a BMM including age, family history, and bioavailable testosterone.
b BMM including age, PSA, DRE, biopsy GS.
c Separate analyses performed in a single validation cohort.
In conjunction with the European Randomized Study of Screening for Prostate Cancer risk calculator variables (PSA, DRE, TRUS, and PV), Leyten and colleagues considered TMPRSS2:ERG as a dichotomous variable; they demonstrated very high discriminative ability for both PCa (AUC = 0.84) and HGPCa (AUC = 0.84; PCA3 did not independently contribute to this model). Most recently, Tomlins and colleagues validated multivariable models, which include TMPRSS2:ERG and PCA3 scores along with serum PSA (deriving the Mi-Prostate Score [MiPS]) or the PCPTrc (MiPS-PCPT). Their findings were robust across multiple cohorts within the study population, and the clinical benefits were well described at various risk thresholds. For example, at a risk threshold of 40%, the MiPS-PCPT model provided avoidance of 47.0% of biopsies and delayed the diagnosis in only 2.3% of high-grade cancers. At more conservative thresholds, the model avoided 35% of biopsies and delayed the diagnosis of only 1% of HGPCa. Certainly, biomarker panels combining TMPRSS2:ERG and PCA3 seem to hold great promise.
Active surveillance
In 2013, Lin and colleagues studied PCA3 and TMPRSS2:ERG in 387 men enrolled in the Canary Prostate Active Surveillance Study. The investigators observed an increase in the median baseline PCA3 scores based on follow-up biopsy results: 27 for a negative biopsy, 31 for a Gleason score of 6 or less, and 48 for a Gleason score of 7 ( P = .02). The corresponding median TMPRSS2:ERG values were 5, 14, and 29, respectively ( P = .001). The AUC for a Gleason score of 7 was 0.68 for PSA versus 0.70 for PCA3 and TMPRSS2:ERG scores ( P = .08), demonstrating a trend toward significance. Although this study, like most in active surveillance (AS) populations, was limited by sample size and follow-up, there seems to be an association with follow-up biopsy results such that PCA3 and TMPRSS2:ERG could play a role in AS populations moving forward.
TMPRSS2:ERG summary
With a remarkably high specificity for PCa, TMPRSS2:ERG has been largely explored in combination with the more sensitive PCA3 assay. Combining these markers with baseline predictive models like the PCPTrc have produced AUC values approximating 0.80 and upward, providing an opportunity to spare 50% of men from unnecessary biopsies with minimal delays in diagnosing clinically significant cancers. Although these data are encouraging, studies of TMPRSS2:ERG and PCA3 in the context of other promising diagnostic aids, such as PHI and mpMRI, remain limited. Further investigation is necessary to compare these tools and identify how to combine them for maximal clinical benefit.
Other RNA-Based Markers
Although PCA3 and TMPRSS2:ERG are the most thoroughly studied RNA biomarkers, other RNA-based markers have also been studied in urine, often in combination. In 2008, Tomlins and colleagues described outlier expression of serine peptidase inhibitor Kazal type 1 (SPINK1) indicating an aggressive subtype of PCa not secondary to ETS fusion events. The investigators subsequently demonstrated urinary measurement of SPINK1 and its association with biochemical recurrence after RP. Alpha-methylacyl-CoA racemase (AMACR) is a mitochondrial enzyme preferentially overexpressed in PCa tissue. Its use as a biomarker was derived by Zielie and colleagues using RNA transcripts in prostatic secretions, and an assay for use in urine was subsequently developed. Ouyang and colleagues later investigated AMACR in 43 men with PCa and 49 controls. The investigators established a threshold of 10.7 for diagnosis, at which AMACR had 70% sensitivity and 71% specificity for PCa. In combination with PCA3, these measures increased to 81% and 84%. Pertinent studies exploring these and other biomarkers detected in urine are listed in Table 7 . Most recently, Leyten and colleagues identified a 3-gene panel for prediction of a Gleason score of 7 or greater disease. The panel included HOXC6, TDRD1, and DLX1 and outperformed PCA3 (AUC 0.77 vs 0.68) and PSA (AUC 0.77 vs 0.72). In combination with PSA, these markers conferred an AUC of 0.81 for HGPCa and hold great promise moving forward.
Study, N (PCa Prevalence) | Candidates | AUC | Sn (%) | Sp (%) | PPV (%) | NPV (%) |
---|---|---|---|---|---|---|
Laxman et al, 2008, 234 (59%) | PSA | 0.51 | –– | –– | –– | –– |
AMACR | –– | –– | –– | –– | –– | |
ERG | –– | –– | –– | –– | –– | |
GOLPH2 | 0.66 | –– | –– | –– | –– | |
PCA3 | 0.66 | 75 | 56 | 71 | 61 | |
SPINK1 | 0.64 | –– | –– | –– | –– | |
TFF3 | –– | –– | –– | –– | –– | |
TMPRSS2:ERG | –– | –– | –– | –– | –– | |
MPM | 0.76 | 66 | 76 | 80 | 61 | |
Talesa et al, 2009, 90 (49%) | PSA a | 0.67 | –– | –– | –– | –– |
PCA3 | 0.68 | |||||
PSMA | 0.64 | |||||
HEPSIN | 0.48 | |||||
GalNAC-T3 | 0.64 | |||||
PSMA + PSA | 0.82 | |||||
PSMA + PCA3 | 0.80 | |||||
Rigau et al, 2010, 215 (34%) | PSA | 0.60 | –– | –– | –– | –– |
PSGR | 0.68 | 59 | 73 | –– | –– | |
PCA3 | 0.66 | 69 | 59 | –– | –– | |
PSGR, PCA3 | 0.73 | 77 | 60 | 50 | 83 | |
Rigau et al, 2011, 154 (37%) | PSMA | 0.62 | 81 | 41 | –– | –– |
PSGR | 0.65 | 63 | 64 | |||
PCA3 | 0.60 | 86 | 35 | |||
PSMA, PSGR, PCA3 | 0.74 | 89 | 45 | |||
Above + PSA | 0.77 | –– | –– | |||
Jamaspishvili et al, 2011, 104 (60%) | PCA3 | 0.57 | –– | –– | –– | –– |
AMACR b | 0.63 | 63 | 65 | 68 | 53 | |
TRPM8, MSMB | 0.67 | 68 | 62 | 72 | 54 | |
TRPM8, MSMB, AMACR | 0.73 | 77 | 62 | 75 | 63 | |
TRPM8, MSMB, AMACR, PCA3 | 0.74 | 72 | 71 | 79 | 60 | |
Above + SPINK1, EZH2, GOLM1 | 0.74 | 81 | 62 | 77 | 68 | |
Ouyang et al, 2009, 92 (47%) | PSA | 0.59 | 77 | 45 | 55 | 66 |
AMACR | 0.65 | 70 | 71 | 68 | 73 | |
PCA3 | 0.67 | 72 | 59 | 61 | 71 | |
AMACR, PCA3 | –– | 81 | 84 | –– | –– | |
Dimitriades et al, 2013, 66 (21%) | DRE | 0.70 | –– | –– | –– | –– |
PSA | 0.54 | |||||
PCA3 | 0.73 | |||||
TMPRSS2:ERG | 0.75 | |||||
DRE, PSA, PCA3, T2E | 0.89 |
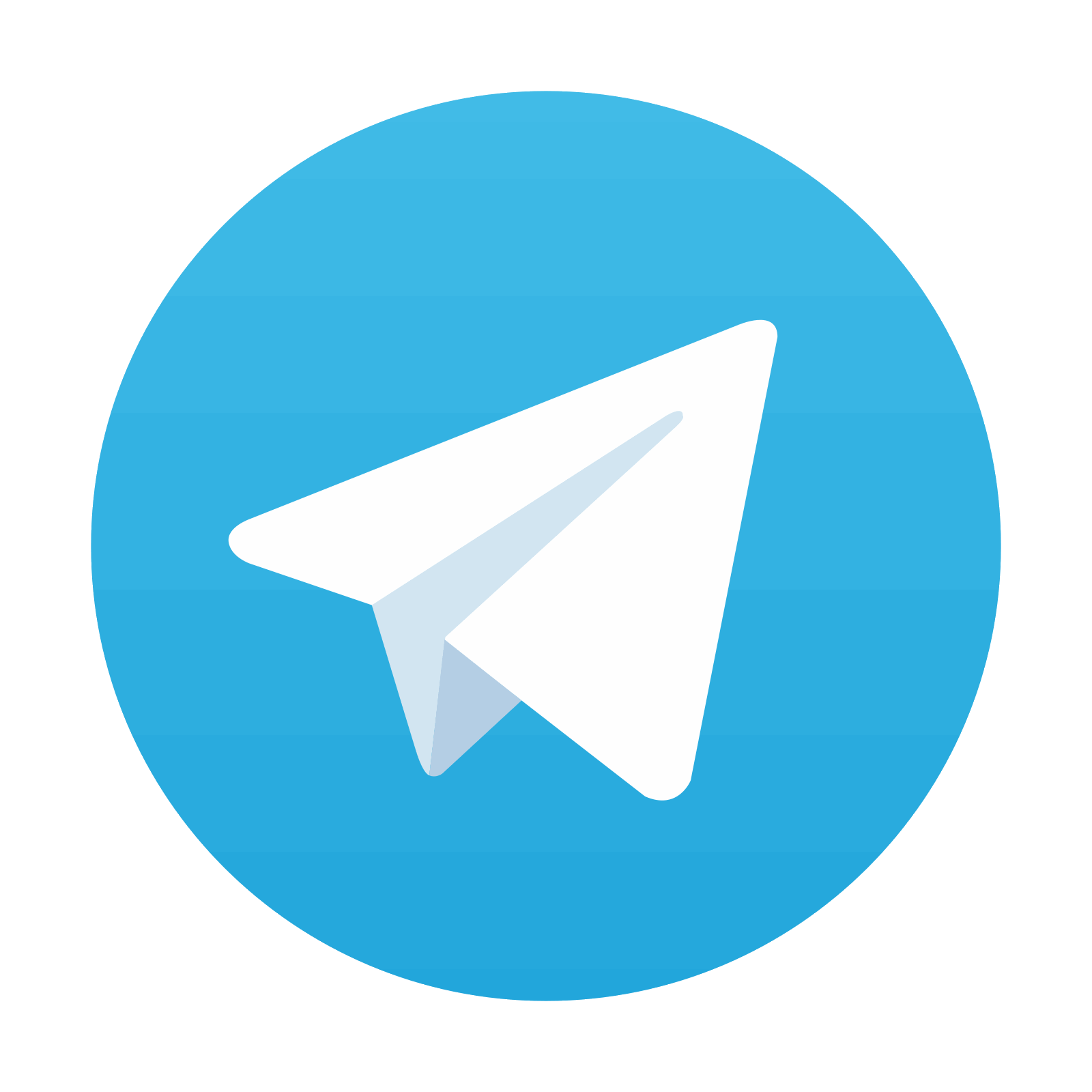
Stay updated, free articles. Join our Telegram channel

Full access? Get Clinical Tree
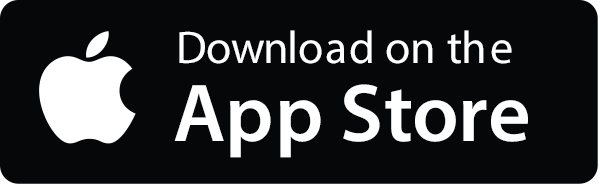

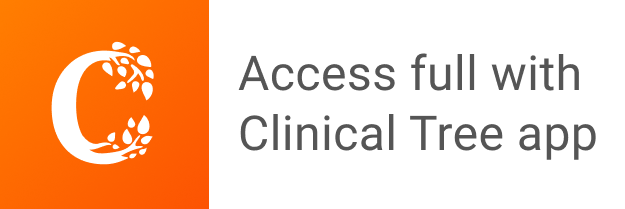