2 Julie Morris University of Manchester, Manchester, UK A basic knowledge of statistics is an essential skill for medical practitioners. Definitions and worked examples of key statistical concepts are provided, including descriptive summary statistics, hypothesis testing, statistical comparison tests, and correlation. Measures of diagnostic accuracy tests and elements of study design, including sample size and randomisation, are also presented. Keywords summary statistics; confidence intervals; significance tests; correlation; diagnostic tests; study design; systematic reviews Statistics and statistical methodology have a central role to play in many areas of the medical world, from research to evidence‐based medicine to published papers in medical journals. It is therefore essential that medical practitioners have a basic understanding of statistical concepts, issues, and techniques. This chapter explains basic statistical principles and covers summary statistics, significance tests, and the design of studies. Examples from the field of urology illustrate each statistical point. Data can be summarised in various ways. The most appropriate statistics depend on the type of data and the distribution of values. For categorical data, such as presence or absence of disease or stage of disease (mild, moderate, or severe), data are summarised by the number of subjects in each category and the percentage in each category. Example 2.1 emphasises the need to look at both the actual numbers as well as the percentages when interpreting categorical data. We define rate as the number of events (e.g. cases of disease) per unit of population during a particular period of time. Table 2.1 includes the definition of two specific rates, ‘incidence’ and ‘prevalence’. Table 2.1 Summary statistics: Definitions. For quantitative data (numerical data such as length of stay or systolic blood pressure), there is a greater selection of appropriate summary statistics. Example 2.2 shows data from a cohort of patients undergoing a surgical procedure. There are a number of different summary statistics available, and they are shown in Table 2.1. You may ask, ‘Why are different summary statistics used?’ The answer is that the appropriate summary statistics to choose depend on how the data are distributed. If data are normally distributed, then the appropriate summary statistics are the mean, the standard deviation (SD), or the variance. [Note the variance is the square of the SD]. If data are not normally distributed, then the appropriate summary statistics are the median, the range, or the interquartile range. The latter is the 25th to the 75th percentile (i.e. when the data are ordered from the smallest to the largest, the lower value of the interquartile range is the ‘quarter’ point, and the higher value is the ‘three‐quarter’ point). It contains the middle 50% of the data and is sometimes used instead of the range when the sample size of the data set is very large (n = 100 or more). Hence, for quantitative data, the distribution of data needs to be assessed before deciding on the appropriate summary statistics. The easiest way to assess the distribution of data is to look at a histogram of the values (Figure 2.1). Figure 2.1 Distribution of blood loss. The data are separated into sections (bars) – in this example the bars correspond to 5 ml categories – and the height of the bars correspond to the number of people in that particular section (i.e. blood loss category). Data are said to be normally distributed if the shape of the histogram is a symmetric upturned U‐shape or bell‐shape. This is true for the blood loss data, and hence, blood loss would be said to be normally distributed. Figure 2.2 shows a histogram of the operating time, and is an example of not normally distributed data. Figure 2.2 Distribution of operating times. The histogram shows a very skewed distribution (not a symmetric bell shape). These data are ‘positively skewed’ (the tail of the distribution is towards the right), which is a common occurrence in medical data where just a few patients have high abnormal values. These values are called ‘outliers’ and would have a large influence on the average (mean) value, which would make it an inappropriate summary statistic. Median values are unaffected by outliers. For ‘negatively skewed’ data, the tail of the distribution is towards the left, and there would be a few small abnormal values (outliers). There are a number of ways of checking data distributions. Any combination of these four properties of a normal distribution can be used. Figure 2.3 shows a flow chart to aid the selection of the most appropriate summary statistics. Figure 2.3 Flow chart I: the process for selecting the appropriate summary statistics. ‘The estimated mean change in maximum voiding pressure (MVP) after treatment with a muscle relaxant was 16 cmH 2 0 with 95% confidence interval (8 cmH 2 0, 24 cmH 2 0).’ The main purpose of confidence intervals (CIs) is to indicate the precision with which an estimate is calculated from the study sample. It presents a range of values in which the population value (the ‘true’ value) may lie with a reasonable level of confidence. The (im)precision is indicated by the width of the CI; the narrower the interval is, the better the precision. The width depends on three factors. The width decreases (and precision increases) with a larger sample size, lower variability between subjects, and lower confidence (a 90% CI will be narrower than a 95% CI). It is important to note that the interpretation of the CI does not directly relate to the actual observations. That is, a 95% CI does not contain 95% of observations. Instead, it relates to the accuracy of the estimated effect size (e.g. the mean difference pre to post for a single group of patients). CIs can be thought of as bridging the gap between summary statistics and formal significance tests (our next topic). If the 95% CI in the example is changed to (−4 cmH 2 0, 36 cmH 2 0), then, because it contains zero, it means that a zero‐change in MVP is feasible. Hence, the study has not shown good evidence that there is any increase in MVP with muscle relaxant. This reflects the result that would be obtained by a formal significance test. That is, there is no significant change in MVP. When carrying out a clinical study, the aim is to measure the strength of evidence provided by the data for and against a specific proposition. Suppose we have two types of surgery, X and Y, and wish to find out whether the complication rate after X is lower than after Y. The results of the study are depicted in Table 2.2: Table 2.2 Results post surgery. Do these data show enough evidence that, in general, patients having surgery X have a significant chance of a lower complication rate than those having surgery Y? To answer this question, we carry out a statistical significance test. In carrying out such a test, we are looking to see whether the data from the study supports one of two scenarios or hypotheses. One scenario is that the complication rates are the same (the null hypothesis). The other scenario is that the complication rates are different (the alternative hypothesis). In general terms: Null hypothesis: Effects of X and Y are the same Alternative hypothesis: Effects of X and Y are different To find out which scenario is best supported by the data, we calculate a ‘p‐value’. The p‐value is a probability. Probabilities correspond to the chance of something (e.g. an event/situation) happening or being true. It takes values between 0 and 1. A probability of 0 means that there is no chance of the event happening or the situation being true. A probability of 1 means that we are certain that the event does happen or that the situation is true. In the context of significance tests, the p‐value corresponds to the probability of the null scenario/hypothesis being true, given the evidence from the study data. It is derived using a mathematical formula on the study data. If the p‐value is small (by convention ≤ 0.05), then we say that the null scenario (that X and Y have the same effect) is unlikely to be true. Hence, we say the alternative scenario (that X and Y have different effects) is likely to be true. We state, therefore, that the difference between X and Y is ‘statistically significant’. If the p‐value is large (by convention > 0.05), then we say that the null scenario (that X and Y have the same effect) could be true. We state, therefore, that the difference between X and Y is ‘not statistically significant’. In the example, the p‐value for the comparison of complication rates was 0.15. How should this be interpreted? It is greater than 0.05, and hence, we conclude that the data have not given us sufficient evidence to determine that there is a difference between X and Y. Thus, patients having surgery X do not have a significant chance of a lower complication rate than those having surgery Y, and we say the difference is not statistically significant. This depends on the type of data and the specific comparison being made. Figure 2.4 shows the process for selecting the appropriate test for the comparison of different groups of subjects when the outcome is a quantitative or numerical measure (Example 2.3).
Principles of Medical Statistics
Abstract
2.1 Introduction
2.2 Descriptive Statistics
2.2.1 Qualitative or Categorical Data
Incidence rate
The number of new cases of disease developing during a particular time period. For example, the annual rate of newly diagnosed cases per 10 000 population.
Prevalence rate
The number of cases of disease that exist at a particular point in time.
Mean
Sum of all the observations divided by the total number of observations.
Median
The middle value (the 50th percentile) when data are ordered from the smallest to the largest.
Variance
The sum of the squares of the difference between each observation and the mean, divided by the total number of observations minus one.
Standard deviation
The square root of the variance.
Range
The interval defined by the minimum and maximum values.
Interquartile range
The interval defined by the 25th and 75th percentiles.
2.2.2 Quantitative or Numerical Data
2.3 Confidence Intervals
2.4 Significance Tests
Observed postoperative complication rates
After surgery X
After surgery Y
5%
10%
2.4.1 What Statistical Test Should Be Used?
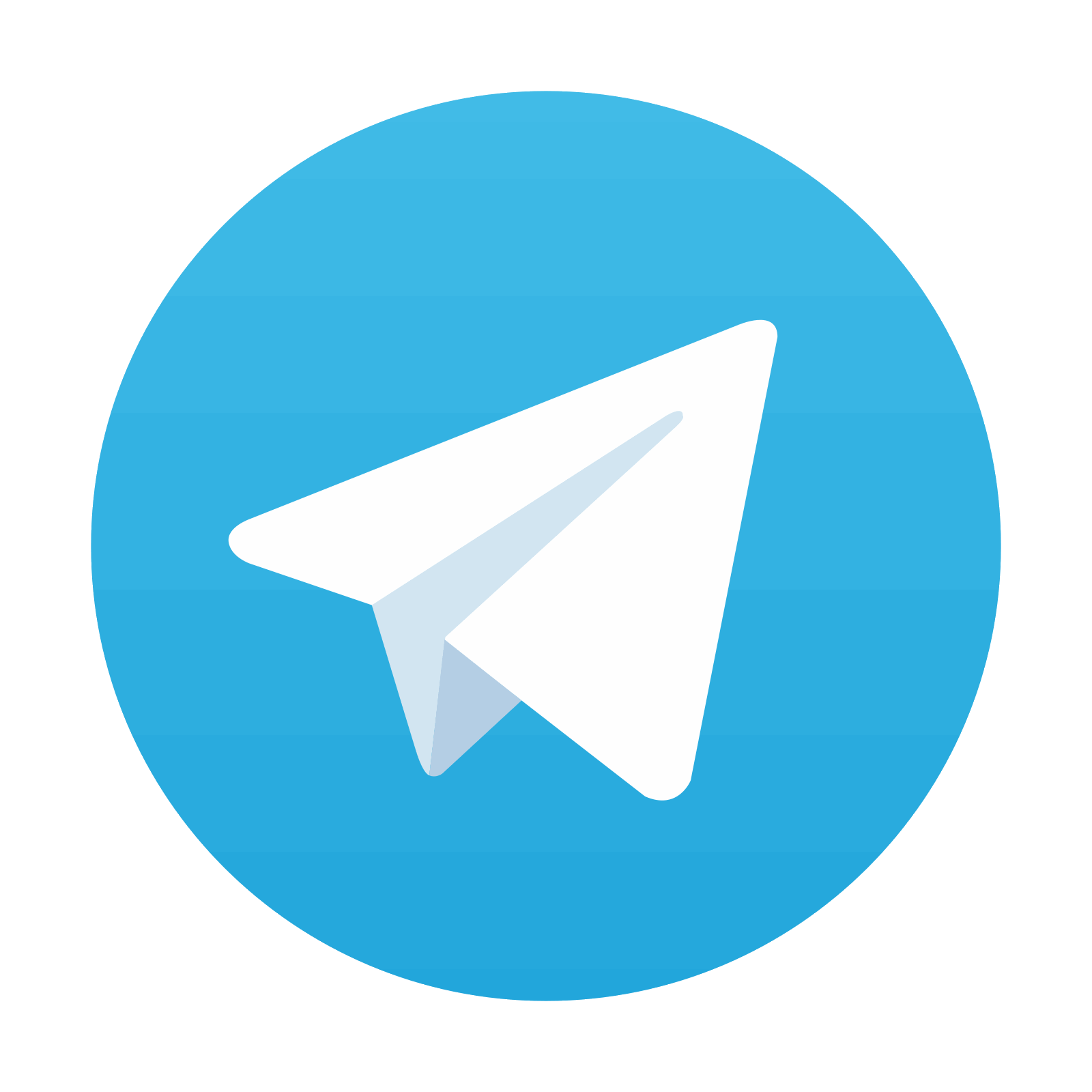
Stay updated, free articles. Join our Telegram channel

Full access? Get Clinical Tree
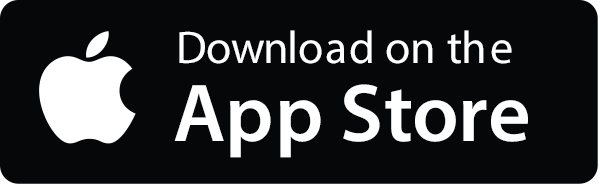
