Administrative databases, registries, and clinical databases are designed for different purposes and therefore have different advantages and disadvantages in providing data for enhancing quality. Administrative databases provide the advantages of size, availability, and generalizability, but are subject to constraints inherent in the coding systems used and from data collection methods optimized for billing. Registries are designed for research and quality reporting but require significant investment from participants for secondary data collection and quality control. Electronic health records contain all of the data needed for quality research and measurement, but that data is too often locked in narrative text and unavailable for analysis. National mandates for electronic health record implementation and functionality will likely change this landscape in the near future.
Studies that help define quality and population-based quality measurement both require large sources of data, including administrative databases, population-based surveys, disease or procedure-specific registries, and data aggregated from electronic health records. It is evident to all attempting to write evidence-based quality measures that ongoing studies are needed to define quality. Population-based quality measurement can enhance quality through promoting system change. Measurement of group or individual provider quality, although not producing quality by the measurement process alone, can enhance quality by motivating the providers to change behavior. As with population-based studies, measurement of individual quality often requires use of administrative data, quality-oriented registries, or electronic health records as data sources. The purpose of this article is to explore the use of these data sources to enhance quality, look for current examples of their use, and then consider the advantages and challenges of each.
Administrative databases, registries, and clinical databases are defined and differentiated by an axis representing purpose, administrative databases primarily used for billing, health care system enrollment and management, clinical databases as record repositories and for communication of clinical care, and registries as limited collections of data for specific research purposes. But there is significant overlap, truly a spectrum of large datasets available for enhancing quality; registries may contain data that is considered administrative, such as billing codes, along with data extracted from electronic health records, and so forth. For purposes of this article, the authors consider administrative data to be any data, coded or otherwise, collected for purposes of billing, scheduling, ordering services or supplies, or for compliance with regulatory agencies. They contain demographics and plausible records of medications dispensed and immunizations given. Registries are created for research, but differ from protocol-driven research databases by intent, used primarily for observational rather than interventional research. Although research using registries is still driven by research hypotheses, by nature they can be used to answer multiple questions and may evolve over time. Although registries usually include data that is also contained in the clinical record, registry data is often categorical and more analytically structured than the same data as captured during clinical care. Clinical databases are created to support clinical care, and although discrete (and therefore computable) data is the rule for laboratory results and demographics, narrative text is the rule for most documentation, including that of clinical encounters, pathology, and radiology reports and even of clinical orders, such as for pharmaceuticals.
Data gathered for all 3 purposes, however, can be used to enhance quality along a second axis representing the types of quality enhancement. Discovery of quality or lack of quality in the population can result in hypotheses for appropriate quality measures. Measuring disparities in quality in the population can promote measures for improving population health. Quantifying quality within health care facilities or plans, by provider group or individual provider, can promote quality through feedback, pay for performance, or requirements for licensing and certification.
The use of large databases to enhance quality: The National Healthcare Quality Report example
Large databases result from data collected for administrative purposes, from surveys performed for developing national agendas and other reasons, or from disease or procedure-specific registries. The authors are familiar with the use of these large databases for epidemiologic studies, including studies of the burden of gastrointestinal disease. The National Healthcare Quality Reports (NHQR) are an example of use of these sources for discovery of quality and of the trends in quality care.
The NHQRs have resulted from a 1999 congressional mandate to the Agency for Healthcare Research and Quality (AHRQ) and have been developed in coordination with the Department of Health and Human Services. The most recent report available containing measures for colorectal cancer screening is the 2008 report, which includes data from 2005 with comparison data from as early as 1999, depending upon the measure. The 2008 NHQR used 33 databases ( Box 1 ) to report on process and outcome measures aggregated at the national and state level. As defined in this report, process measures “track the receipt of medical services”; whereas, outcome measures “in part reflect the results of medical care.” Of 40 core report measures and 9 composite measures, 3 core measures for colorectal cancer are reported every other year along with a fourth noncore measure endorsed by the National Quality Forum concerning surgical resection of colorectal cancer. The 2008 report measures and findings include the following information:
- •
Colorectal cancer screening: adults aged 50 years and older who ever received colorectal cancer screening (colonoscopy, sigmoidoscopy, proctoscopy, or fecal occult blood test)
In 2005, 55.5% of adults aged 50 years and older (49.2% in individuals aged 50–64 years, 63.1% for adults aged 65 years and older) had received screening for colorectal cancer. This result was an increase from 49.8% in 2000.
- •
Advanced stage colorectal cancer: colorectal cancer diagnosed at advance stage (tumors diagnosed at regional or distant site) per 100,000 population aged 50 years and older
In 2005, 80.8 advanced stage colorectal tumors were diagnosed per 100,000 population. There has been a steady decrease in this number since first reported in 2000 at 95.2 per 100,000 population.
- •
Colorectal cancer mortality: colorectal cancer deaths per 100,000 population per year
The United States Healthy People 2010 goal for colorectal cancer deaths is 13.7 per 100,000. A steady downward trend has occurred since 1999 with 17.5 per 100,000 in 2005.
Survey data collected from populations
- •
AHRQ, Medical Expenditure Panel Survey, 2000 to 2005
- •
US Centers for Disease Control and Prevention (CDC), Behavioral Risk Factor Surveillance System, 2001 to 2006
- •
CDC-National Center for Health Statistics (NCHS), National Health and Nutrition Examination Survey, 1999 to 2006
- •
CDC-NCHS, National Health Interview Survey, 1998 to 2006
- •
CDC-NCHS/National Immunization Program, National Immunization Survey, 1998 to 2006
- •
Centers for Medicare and Medicaid Services (CMS), Medicare Current Beneficiary Survey, 1998 to 2004
- •
National Center for Education Statistics, National Assessment of Adult Literacy, Health Literacy Component, 2003
- •
National Hospice and Palliative Care Organization, Family Evaluation of Hospice Care, 2005 to 2007
- •
National Institutes of Health (NIH), National Institute of Mental Health, Collaborative Psychiatric Epidemiology Surveys, 2001 to 2003
- •
Substance Abuse and Mental Health Services Administration, National Survey on Drug Use and Health, 2002 to 2006
- •
US Census Bureau, American Community Survey, 2006
Data collected from samples of health care facilities and providers
- •
American Cancer Society and American College of Surgeons, National Cancer database, 1999 to 2005
- •
CDC-NCHS, National Ambulatory Medical Care Survey, 1997–2006
- •
CDC-NCHS, National Hospital Ambulatory Medical Care Survey-Emergency Department, 1997 to 2006
- •
CDC-NCHS, National Hospital Ambulatory Medical Care Survey-Outpatient Department, 1997 to 2006
- •
CDC-NCHS, National Hospital Discharge Survey, 1998 to 2006
- •
CMS, End Stage Renal Disease Clinical Performance Measures Project, 2001 to 2006
Data extracted from data systems of health care organizations
- •
AHRQ, Healthcare Cost and Use Project, Nationwide Inpatient Sample, 1994, 1997, 2000 to 2005, and State Inpatient Databases, 2001 to 2005
- •
CMS, Home Health Outcomes and Assessment Information Set, 2002 to 2006
- •
CMS, Hospital Compare, 2006
- •
CMS, Medicare Patient Safety Monitoring System, 2004 to 2006
- •
CMS, Nursing Home Minimum Data Set, 1999 to 2006
- •
CMS, Quality Improvement Organization program, Hospital Quality Alliance measures, 2002 to 2006
- •
HIV Research Network data, 2003 to 2005
- •
Indian Health Service, National Patient Information Reporting System, 2002 to 2005
- •
National Committee for Quality Assurance, Health Plan Employer Data and Information Set, 2001 to 2005
- •
NIH, United States Renal Data System, 1998–2004
- •
SAMHSA, Treatment Episode Data Set, 2002–2005
Data from surveillance and vital statistics systems
- •
CDC-National Center for HIV, Viral Hepatitis, STD, and TB Prevention, HIV/AIDS Reporting System, 1998 to 2006
- •
CDC-National Center for HIV, STD, and TB Prevention, TB Surveillance System, 1999 to 2004
- •
CDC-National Program of Cancer Registries, 2000 to 2004
- •
CDC-NCHS, National Vital Statistics System, 1999 to 2005
- •
NIH-National Cancer Institute, Surveillance, Epidemiology, and End Results program, 2000 to 2005
Data from National Healthcare Quality Report 2008. Agency for Healthcare Research and Quality. Available at: http://www.ahrq.gov/qual/nhqr08/nhqr08.pdf . Accessed February 14, 2010.
The application of large databases for quality enhancement requires significant resources and analytical expertise. The quality of these data sources must be judged in the same ways as all data found in clinical and research settings, and accurate interpretation of the data demands availability of analysts with appropriate expertise with both the sources’ database structure and content. The possible effects of such reporting, however, can be seen in system changes after feedback of measurement results to providers; national, state, and local administrative units and health care organizations; and from the informed implementation of clinical decision support systems that support just-in-time quality care.
Challenges in the use of administrative data
The credibility of administrative data, which is usually insurance claims data, for quality reporting and research has long been the subject of debate. The term powerful is often used to describe the role of administrative data in studying population-based patterns of health, disease, and medical care. This powerful data has potential advantages for research and quality, including the ability to measure large samples of geographically dispersed patients, the ability to assemble longitudinal records of both inpatient and outpatient care and across providers, and the fact that the data is already collected and inexpensive for investigators to obtain relative to the collection of clinical data through chart abstraction.
Administrative data, however, is not collected for the purpose of measuring quality and its validity for this purpose has been questioned. Of particular concern with US administrative databases is the use of 2 coding systems: the International Classification of Diseases, 9th Revision, Clinical Modification (ICD-9-CM), which is used for coding diagnoses by both hospitals and physicians and for coding procedures by hospitals; and the American Medical Association’s Current Procedural Terminology (CPT), which is used by physicians for coding procedures. For insightful review of the use of administrative data for assessing quality across a decade, see the review and editorial by Iezzoni. This author describes how administrative files often explicitly aim to minimize data collection by limiting the number of slots available for codes that may affect completeness of comorbidity documentation, and that coding inaccuracy may occur in an effort to maximize payment, as from the Medicare Prospective Payment System using diagnosis-related groups, or to minimize the perception of poor quality through underreporting of complications. The coding systems for procedures (ICD-9-CM for hospitals and CPT for physicians) do not readily link, “hindering comparisons between hospital-generated and physician-generated data.” Until 2007, diagnoses present on admission could not be differentiated from complications occurring during hospitalization in Medicare billing data.
Administrative data has been shown in some circumstances to lack either specificity or sensitivity for identifying conditions important in quality measurement. Jollis and colleagues demonstrated that claims data failed to identify more than 50% of patients with any of several important conditions when compared with a clinical information system. Other studies reported by Jollis found that clinical criteria for acute myocardial infarction were found in only 43% to 87% of records having this diagnosis coded at discharge. Keating and colleagues used 3 accepted measures of quality care for diabetes and compared the use of administrative data alone with administrative data plus medical record data from 3 health plans. Underdetection of quality indicators was noted for all 3 health systems using administrative data alone, and, importantly, the ranking of the health systems changed for some indicators when data from both sources was used. In addition, the degree of underdetection varied by patient age and race, pointing out the complexity of accurate quality reporting.
In quality measurement, inclusion of cases in the denominator of a measure that do not accurately represent the condition being tested can falsely conclude underperformance on the measure. Solberg and colleagues explored algorithms for improving the positive predictive value of case identification for 3 conditions using administrative records, after discovering that using a single code to identify cases of diabetes might include up to 80% of cases without diabetes. The algorithms developed for accurate case identification required for all conditions that more than a single code be used. For example, accurately identifying cases with diabetes required either 2 or more outpatient or 1 inpatient ICD-9 code for diabetes in a year or a filled prescription for a diabetes-specific medication (excluding a medication that may be used for diabetes and other conditions), and assumed health plan enrollment for at least 11 months of the year being studied.
Incomplete physician documentation can lead to omissions in administrative codes. Davenport and colleagues compared data on surgeries performed at a single institution as recorded in 2 volunteer systems; the University HealthSystem Consortium Clinical Database, which relies on administrative data, primarily ICD-9 codes entered by hospital billing coders; and the National Surgical Quality Improvement Program, which requires that nurses extract the data from the medical record and code it to risk and outcome variables specifically designed for risk-adjusting surgical outcomes. To justify billing, the billing coders are required to code only conditions and procedures that are clearly documented by physicians, sometimes with specific wording, and therefore cannot use conditions and procedures that are identifiable in nursing and other notes.
Ultimately, the lesson learned is that for administrative data to be used for enhancing quality, its use is subject to the same caveats as for successful conduct of research using any large databases; the investigator must have adequate knowledge of the database structure and its contents, in particular, knowledge of the completeness and accuracy of the data contained and must be cautious in the conclusions drawn. Comparison with clinical databases, including chart validation of a subset of cases of interest, should be considered before determining the accuracy of the codes used, although this may be untenable for many administrative databases, especially those in the public domain without the ability to link to identified health records.
A recent study on the association of colonoscopy and death from colorectal cancer (CRC) highlights some of the advantages and limitations of using administrative databases in research. The design was a population-based case-control study in Ontario, using administrative claims to ascertain exposure to colonoscopy and diagnosis of CRC. Exposures in more than 10,000 case subjects (with CRC) were compared with controls matched for age, sex, geographic region, and socioeconomic status. The analysis found that subjects with prior colonoscopy had a 67% reduction in risk of death from left-sided CRC compared with controls and no reduction in risk of death from right-sided cancer. The study raised serious concerns about the potential benefits of screening with colonoscopy.
The strengths of this study include the large number of cases with matched controls. Cases and controls came from typical practice settings, suggesting that data could be generalizable. There is some biologic plausibility to the outcome; cancers of the right colon may be biologically different from distal cancers.
The limitations are also significant and are related to the administrative nature of the data. First, the indications for colonoscopy are unknown. It is unlikely that most examinations were screening examinations during the time period of the study (1992–2003), when little screening colonoscopy was performed in Canada. If rectal bleeding was a common indication, the procedures may have been more likely to detect left-sided lesions because these may present with bleeding, and exerted a more protective effect in the left colon because of selection bias. A second limitation is that we cannot determine the quality or completeness of the colonoscopy examination from the administrative database. For example, it is quite possible that some examinations coded as colonoscopy were incomplete, and did not fully visualize the proximal colon. It is also possible that the bowel prep was poor in the proximal colon. In both situations, the outcome could be a poor quality examination of the proximal colon, which could result in failure of colonoscopy to exert a protective effect. Finally, it is not known if there were polyps seen but not removed at colonoscopy. Based on the National Polyp Study, polypectomy was associated with a lower than expected rate of CRC during surveillance, suggesting that one of the primary benefits of colonoscopy is the detection and removal of cancer precursor lesions. Using this administrative database, the authors cannot determine if all detected polyps were removed. The limitations of this study are largely caused by the lack of data precision in the administrative database, and potentially compromise the outcome. The authors cannot determine if the result is caused by poor quality colonoscopy, or true biologic differences in the behavior of proximal and distal CRC.
Challenges in the use of administrative data
The credibility of administrative data, which is usually insurance claims data, for quality reporting and research has long been the subject of debate. The term powerful is often used to describe the role of administrative data in studying population-based patterns of health, disease, and medical care. This powerful data has potential advantages for research and quality, including the ability to measure large samples of geographically dispersed patients, the ability to assemble longitudinal records of both inpatient and outpatient care and across providers, and the fact that the data is already collected and inexpensive for investigators to obtain relative to the collection of clinical data through chart abstraction.
Administrative data, however, is not collected for the purpose of measuring quality and its validity for this purpose has been questioned. Of particular concern with US administrative databases is the use of 2 coding systems: the International Classification of Diseases, 9th Revision, Clinical Modification (ICD-9-CM), which is used for coding diagnoses by both hospitals and physicians and for coding procedures by hospitals; and the American Medical Association’s Current Procedural Terminology (CPT), which is used by physicians for coding procedures. For insightful review of the use of administrative data for assessing quality across a decade, see the review and editorial by Iezzoni. This author describes how administrative files often explicitly aim to minimize data collection by limiting the number of slots available for codes that may affect completeness of comorbidity documentation, and that coding inaccuracy may occur in an effort to maximize payment, as from the Medicare Prospective Payment System using diagnosis-related groups, or to minimize the perception of poor quality through underreporting of complications. The coding systems for procedures (ICD-9-CM for hospitals and CPT for physicians) do not readily link, “hindering comparisons between hospital-generated and physician-generated data.” Until 2007, diagnoses present on admission could not be differentiated from complications occurring during hospitalization in Medicare billing data.
Administrative data has been shown in some circumstances to lack either specificity or sensitivity for identifying conditions important in quality measurement. Jollis and colleagues demonstrated that claims data failed to identify more than 50% of patients with any of several important conditions when compared with a clinical information system. Other studies reported by Jollis found that clinical criteria for acute myocardial infarction were found in only 43% to 87% of records having this diagnosis coded at discharge. Keating and colleagues used 3 accepted measures of quality care for diabetes and compared the use of administrative data alone with administrative data plus medical record data from 3 health plans. Underdetection of quality indicators was noted for all 3 health systems using administrative data alone, and, importantly, the ranking of the health systems changed for some indicators when data from both sources was used. In addition, the degree of underdetection varied by patient age and race, pointing out the complexity of accurate quality reporting.
In quality measurement, inclusion of cases in the denominator of a measure that do not accurately represent the condition being tested can falsely conclude underperformance on the measure. Solberg and colleagues explored algorithms for improving the positive predictive value of case identification for 3 conditions using administrative records, after discovering that using a single code to identify cases of diabetes might include up to 80% of cases without diabetes. The algorithms developed for accurate case identification required for all conditions that more than a single code be used. For example, accurately identifying cases with diabetes required either 2 or more outpatient or 1 inpatient ICD-9 code for diabetes in a year or a filled prescription for a diabetes-specific medication (excluding a medication that may be used for diabetes and other conditions), and assumed health plan enrollment for at least 11 months of the year being studied.
Incomplete physician documentation can lead to omissions in administrative codes. Davenport and colleagues compared data on surgeries performed at a single institution as recorded in 2 volunteer systems; the University HealthSystem Consortium Clinical Database, which relies on administrative data, primarily ICD-9 codes entered by hospital billing coders; and the National Surgical Quality Improvement Program, which requires that nurses extract the data from the medical record and code it to risk and outcome variables specifically designed for risk-adjusting surgical outcomes. To justify billing, the billing coders are required to code only conditions and procedures that are clearly documented by physicians, sometimes with specific wording, and therefore cannot use conditions and procedures that are identifiable in nursing and other notes.
Ultimately, the lesson learned is that for administrative data to be used for enhancing quality, its use is subject to the same caveats as for successful conduct of research using any large databases; the investigator must have adequate knowledge of the database structure and its contents, in particular, knowledge of the completeness and accuracy of the data contained and must be cautious in the conclusions drawn. Comparison with clinical databases, including chart validation of a subset of cases of interest, should be considered before determining the accuracy of the codes used, although this may be untenable for many administrative databases, especially those in the public domain without the ability to link to identified health records.
A recent study on the association of colonoscopy and death from colorectal cancer (CRC) highlights some of the advantages and limitations of using administrative databases in research. The design was a population-based case-control study in Ontario, using administrative claims to ascertain exposure to colonoscopy and diagnosis of CRC. Exposures in more than 10,000 case subjects (with CRC) were compared with controls matched for age, sex, geographic region, and socioeconomic status. The analysis found that subjects with prior colonoscopy had a 67% reduction in risk of death from left-sided CRC compared with controls and no reduction in risk of death from right-sided cancer. The study raised serious concerns about the potential benefits of screening with colonoscopy.
The strengths of this study include the large number of cases with matched controls. Cases and controls came from typical practice settings, suggesting that data could be generalizable. There is some biologic plausibility to the outcome; cancers of the right colon may be biologically different from distal cancers.
The limitations are also significant and are related to the administrative nature of the data. First, the indications for colonoscopy are unknown. It is unlikely that most examinations were screening examinations during the time period of the study (1992–2003), when little screening colonoscopy was performed in Canada. If rectal bleeding was a common indication, the procedures may have been more likely to detect left-sided lesions because these may present with bleeding, and exerted a more protective effect in the left colon because of selection bias. A second limitation is that we cannot determine the quality or completeness of the colonoscopy examination from the administrative database. For example, it is quite possible that some examinations coded as colonoscopy were incomplete, and did not fully visualize the proximal colon. It is also possible that the bowel prep was poor in the proximal colon. In both situations, the outcome could be a poor quality examination of the proximal colon, which could result in failure of colonoscopy to exert a protective effect. Finally, it is not known if there were polyps seen but not removed at colonoscopy. Based on the National Polyp Study, polypectomy was associated with a lower than expected rate of CRC during surveillance, suggesting that one of the primary benefits of colonoscopy is the detection and removal of cancer precursor lesions. Using this administrative database, the authors cannot determine if all detected polyps were removed. The limitations of this study are largely caused by the lack of data precision in the administrative database, and potentially compromise the outcome. The authors cannot determine if the result is caused by poor quality colonoscopy, or true biologic differences in the behavior of proximal and distal CRC.
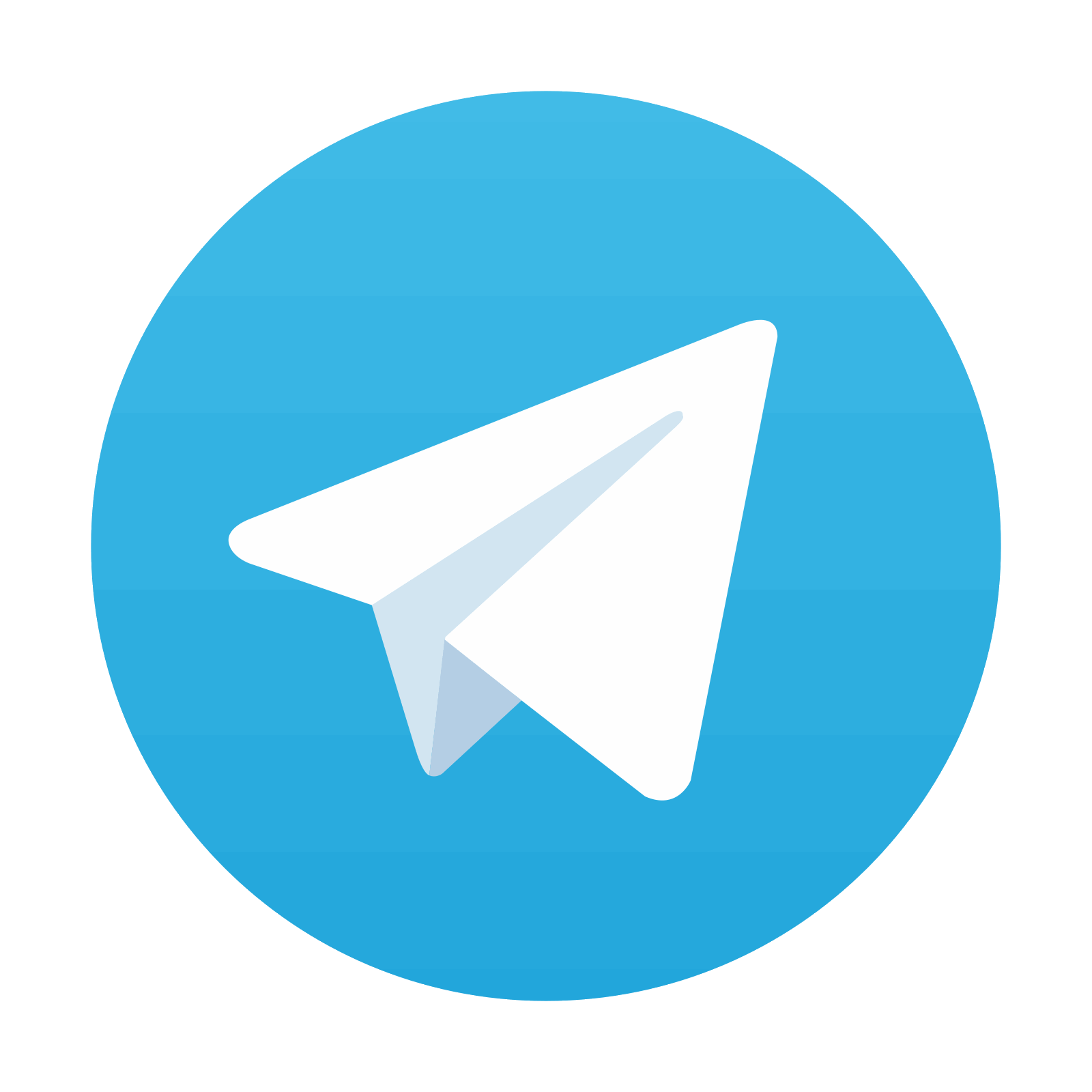
Stay updated, free articles. Join our Telegram channel

Full access? Get Clinical Tree
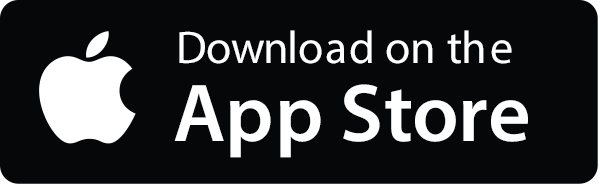
