The natural history of non-muscle invasive bladder cancer (NMIBC) in individual patients can be unpredictable. Although there are known clinical and molecular factors associated with tumor recurrence and progression, it is challenging to reconcile these data during a typical patient encounter within a busy clinic. The authors discuss the European Organization for Research and Treatment of Cancer’s risk tables along with other models for predicting prognosis in patients with NMIBC. The authors also describe their advantages and disadvantages and the barriers to using these risk models in daily clinical practice and provide a future perspective on prognostic models.
Key points
- •
The natural history of non-muscle invasive bladder cancer in individual patients can be unpredictable.
- •
Although there are known clinical and molecular factors associated with tumor recurrence and progression, it is challenging to reconcile these data during a typical patient encounter within a busy clinic.
- •
Prognostic models, such as risk tables and nomograms, aim to facilitate risk stratification, patient counseling, and treatment decision making.
- •
There are many prognostic models available for non-muscle invasive bladder cancer, but they are not commonly used in daily practice because of their complexity and limited usefulness in treatment decision making.
- •
To make prognostic models more useful, the focus should be on the clinical implications of the model for the patient, such as by focusing on negative and positive predictive value, rather than P values, sensitivity, and specificity. The net benefit of the model should be compared with the standard model by means of classification tables and decision analytic techniques to test its additional clinical value.
- •
Biomarkers do not have sufficient additional value, and markers undergoing investigation should first stand the test of time.
- •
Ultimately, even good models will not be translated into clinical practice unless they can be integrated into the standard clinical workflow.
Background
Overall, bladder cancer (BC) is the seventh most common malignancy in men and the 17th in women. The incidence increases with age and is highest at 50 to 70 years of age. Eighty percent of patients with BC are men. Important risk factors are chemical and environmental exposures, such as smoking and aromatic amines, and chronic irritation. In the Western world, more than 90% of BC are urothelial carcinomas or transitional cell carcinoma.
On average, 70% of patients with BC present with non-muscle invasive BC (NMIBC) and the remainder with muscle-invasive disease (MIBC). In the non-muscle invasive group, approximately 70% present as Ta lesions (noninvasive papillary carcinoma), 20% as T1 lesions (invasion into subepithelial connective tissue), and 10% as carcinoma in situ (CIS or Tis lesions; high-grade noninvasive flat tumor). For grading, both the World Health Organization’s (WHO) 1973 and the WHO’s 2004 classifications are advised. The WHO’s 1973 grading system recognizes 3 groups: grade 1 to 3. The WHO’s 2004 classification defines 4 groups of papillary lesions: urothelial papilloma (benign), papillary urothelial neoplasm of low malignant potential, low-grade papillary urothelial carcinoma, and high-grade papillary urothelial carcinoma. For staging, the TNM classification is used.
Another way to stratify patients is by prognostic factors and, thus, outcome. The European Organization for Research and Treatment of Cancer (EORTC) developed a prognostic model for recurrence and progression for patients with NMIBC, which the authors discuss in this article. Other prognostic models with applications in urological practice have been created recently using other techniques: nomograms, neuro-fuzzy models, and artificial neural networks (ANN).
The most well-known prognostic model is the risk table, which divides patients into risk groups based on their score. It gives the probability of an event (recurrence, progression) for patients within a given risk group. It assumes that all patients within a given risk group have a similar prognosis; however, the choice of cutoff values when stratifying patients into groups is somewhat artificial. It is unlikely that all patients within a given group will have the same prognosis, and patients with similar scores who fall into different risk groups might not have different prognoses. Furthermore, when one variable is missing, it is not possible to calculate the probabilities. Nevertheless, risk tables can easily identify the very low- and very high-risk patients.
A nomogram is a graphical device that is used to calculate an individual patient’s probability of an event based on a multivariable model with their specific prognostic factors and, hence, gives a more individualized risk calculation. Nomograms are based on a (continuous) score, whereas risk tables subdivide patients into different categories based on their score. They provide a more individualized probability of the event of interest, and software is usually developed to make them easy to use. Because the nomogram probabilities come directly from the multivariable model, it is important that the model is well calibrated, that is, it has an excellent goodness of fit. Otherwise, the probabilities provided by the model will be incorrect. However, when one of the variables is missing for a patient, the nomogram cannot be used for that patient. As mentioned by Hernandez and colleagues, nomograms are usually developed with very large series, and it has to be determined if they are applicable to lower-volume centers.
More advanced prognostic models are neuro-fuzzy models and ANN. The latter is a mathematical model based on a biologic neural network. It can handle complex relationships between input and output and can find patterns in the data. ANN are adaptive systems that can change their structure during the learning phase. A neuro-fuzzy model is a combination of an ANN and fuzzy logistics, which is a form of logistics that can handle reasoning. Because there is little experience in NMIBC with these models, they are not discussed further.
In the next paragraphs, the advantages and disadvantages of the most well-known prognostic model, the EORTC risk tables, are discussed. Then, several other NMIBC prognostic models are described; the authors discuss the lack of use of prognostic models in the daily urological practice. Finally, the authors provide a future perspective on prognostic models: how should we develop and use prognostic models for patients with NMIBC?
Background
Overall, bladder cancer (BC) is the seventh most common malignancy in men and the 17th in women. The incidence increases with age and is highest at 50 to 70 years of age. Eighty percent of patients with BC are men. Important risk factors are chemical and environmental exposures, such as smoking and aromatic amines, and chronic irritation. In the Western world, more than 90% of BC are urothelial carcinomas or transitional cell carcinoma.
On average, 70% of patients with BC present with non-muscle invasive BC (NMIBC) and the remainder with muscle-invasive disease (MIBC). In the non-muscle invasive group, approximately 70% present as Ta lesions (noninvasive papillary carcinoma), 20% as T1 lesions (invasion into subepithelial connective tissue), and 10% as carcinoma in situ (CIS or Tis lesions; high-grade noninvasive flat tumor). For grading, both the World Health Organization’s (WHO) 1973 and the WHO’s 2004 classifications are advised. The WHO’s 1973 grading system recognizes 3 groups: grade 1 to 3. The WHO’s 2004 classification defines 4 groups of papillary lesions: urothelial papilloma (benign), papillary urothelial neoplasm of low malignant potential, low-grade papillary urothelial carcinoma, and high-grade papillary urothelial carcinoma. For staging, the TNM classification is used.
Another way to stratify patients is by prognostic factors and, thus, outcome. The European Organization for Research and Treatment of Cancer (EORTC) developed a prognostic model for recurrence and progression for patients with NMIBC, which the authors discuss in this article. Other prognostic models with applications in urological practice have been created recently using other techniques: nomograms, neuro-fuzzy models, and artificial neural networks (ANN).
The most well-known prognostic model is the risk table, which divides patients into risk groups based on their score. It gives the probability of an event (recurrence, progression) for patients within a given risk group. It assumes that all patients within a given risk group have a similar prognosis; however, the choice of cutoff values when stratifying patients into groups is somewhat artificial. It is unlikely that all patients within a given group will have the same prognosis, and patients with similar scores who fall into different risk groups might not have different prognoses. Furthermore, when one variable is missing, it is not possible to calculate the probabilities. Nevertheless, risk tables can easily identify the very low- and very high-risk patients.
A nomogram is a graphical device that is used to calculate an individual patient’s probability of an event based on a multivariable model with their specific prognostic factors and, hence, gives a more individualized risk calculation. Nomograms are based on a (continuous) score, whereas risk tables subdivide patients into different categories based on their score. They provide a more individualized probability of the event of interest, and software is usually developed to make them easy to use. Because the nomogram probabilities come directly from the multivariable model, it is important that the model is well calibrated, that is, it has an excellent goodness of fit. Otherwise, the probabilities provided by the model will be incorrect. However, when one of the variables is missing for a patient, the nomogram cannot be used for that patient. As mentioned by Hernandez and colleagues, nomograms are usually developed with very large series, and it has to be determined if they are applicable to lower-volume centers.
More advanced prognostic models are neuro-fuzzy models and ANN. The latter is a mathematical model based on a biologic neural network. It can handle complex relationships between input and output and can find patterns in the data. ANN are adaptive systems that can change their structure during the learning phase. A neuro-fuzzy model is a combination of an ANN and fuzzy logistics, which is a form of logistics that can handle reasoning. Because there is little experience in NMIBC with these models, they are not discussed further.
In the next paragraphs, the advantages and disadvantages of the most well-known prognostic model, the EORTC risk tables, are discussed. Then, several other NMIBC prognostic models are described; the authors discuss the lack of use of prognostic models in the daily urological practice. Finally, the authors provide a future perspective on prognostic models: how should we develop and use prognostic models for patients with NMIBC?
EORTC risk tables
Development of the EORTC Risk Tables
In 2006, Sylvester and colleagues published the EORTC scoring system for NMIBC. They combined individual patient data of 2596 patients from 7 EORTC trials (inclusion period: January 1979–September 1989). The aim was to provide simple tables that would allow urologists to easily calculate the probability of recurrence and progression after transurethral resection of the bladder tumor (TURBT) for patients with NMIBC. The most appropriate adjuvant treatment after TURBT and the frequency of follow-up can then be determined in an individual patient based on their prognosis. Data on patient and tumor characteristics and the endpoints of time to first recurrence and time to progression to MIBC were merged. The most important variables were then determined by regression models. Patients were divided into 4 risk groups for both recurrence and progression according to their total score. Probabilities of recurrence and progression at 1 year and 5 years were calculated. Also, software was provided to calculate these probabilities at 1, 2, 3, 4, and 5 years. The model accuracy using Harrell’s bias corrected concordance index (c index) was calculated. The c index is the probability that for 2 patients chosen at random, the patient who had the actual event first had a higher probability of having the event according to the model; an uninformative model will have a c index of 0.5 or 50% (flipping a coin), and a perfect model will have a c index of 1 or 100%. Area under the curve (AUC) can also provide this information but only for binary outcomes (recurrence yes/no). To adjust for bias (overfitting, overoptimism), models were refit 200 times using the bootstrap technique (internal validation). Bootstrapping is a resampling method in which analyses are repeated many times but with different random samples of subjects each: in each analysis some subjects might not be included, others are included once, twice, and so forth. The 2596 eligible patients had mainly favorable characteristics. A total of 22% received no adjuvant intravesical treatment before recurrence; 78% of the patients received intravesical therapy, mostly chemotherapy. The median follow-up was 3.9 years. In total, 47.8% of patients experienced a recurrence with a median time to recurrence of 2.7 years. The most important factors that influenced the time to first recurrence were prior recurrence rate, number of tumors, and tumor size. Only 10.7% of patients experienced progression to muscle-invasive disease. The median time to progression was not observed, with progression rates at 5 years varying from 0.8% to 45%. The most important factors influencing time to progression were T category, CIS, and grade. Scores were calculated for each patient, varying from 0 to 17 for recurrence and 0 to 23 for progression. Table 1 gives an overview of the probabilities of recurrence and progression. Furthermore, Sylvester and colleagues found that concomitant CIS is the most important prognostic factor in patients with pT1G3-tumors, and that recurrence at first follow-up cystoscopy at three months is associated with a higher chance of progression.
Recurrence Score | Probability of Recurrence at 1 y | Probability of Recurrence at 5 y | Recurrence Risk Group | ||
---|---|---|---|---|---|
(%) | (95% CI) | (%) | (95% CI) | ||
0 | 15 | (10–19) | 31 | (24–37) | Low risk |
1–4 | 24 | (21–26) | 46 | (42–49) | Intermediate risk |
5–9 | 38 | (35–41) | 62 | (58–65) | Intermediate risk |
10–17 | 61 | (55–67) | 78 | (73–84) | High risk |
Progression Score | Probability of Progression at 1 y | Probability of Progression at 5 y | Progression Risk Group | ||
---|---|---|---|---|---|
(%) | (95% CI) | (%) | (95% CI) | ||
0 | 0.2 | (0–0.7) | 0.8 | (0–1.7) | Low risk |
2–6 | 1 | (0.4–1.6) | 6 | (5–8) | Intermediate risk |
7–13 | 5 | (4–7) | 17 | (14–20) | High risk |
14–23 | 17 | (10–24) | 45 | (35–55) | High risk |
As mentioned in an editorial comment by Karakiewicz, the internal validation yielded a c index of 0.66 for recurrence at both 1 and 5 years, which means that 66% of recurrences were accurately predicted at 1 and 5 years. The c index for progression was 0.74 at 1 year and 0.75 at 5 years.
In 2013, the EORTC will start updating these risk tables for patients treated with maintenance bacillus Calmette-Guerin (BCG).
The NMIBC guidelines panel of the European Association of Urology (EAU) classified patients into subgroups of low, intermediate, and high risk based on these tables ( Table 1 , last column) and provided treatment and follow-up recommendations depending on a patient’s risk group. The American Urological Association (AUA) also specified therapy based on clinical risk. However, the panel of the AUA defined only 2 risk groups: low-risk patients (pTa, low grade) and high-risk patients (pT1, high grade, and/or CIS).
Disadvantages
As mentioned earlier, patient and tumor characteristics influence the probability of recurrence and progression. However, it should be taken into account that 22% of patients received no intravesical treatment at all, and the treatment that was given consisted mostly of intravesical chemotherapy. Only 171 of the patients (7%) received BCG and none received BCG maintenance. Also, less than 10% of patients received a single immediate postoperative instillation with chemotherapy, and a re-TURBT was not performed in high-risk patients. As mentioned in the discussion by Sylvester and colleagues, data for other factors that might be of prognostic importance were not available: depth of lamina propria invasion, location of the tumor on the bladder wall, lymphovascular invasion, and micropapillary tumors. Also, recent developments, such as molecular markers, fluorescence cystoscopy and re-TURBT, that are likely to further reduce the risks of recurrence and progression were not taken into account. Unfortunately, long-term follow-up is not available in most large series of patients where these new treatment developments and more recently identified prognostic factors have been assessed.
Many of the patients included in the EORTC series, particularly those in the high-risk category, would be undertreated according to today’s standards. As such, the recurrence rates and especially the progression rates are likely to be somewhat higher than those found in contemporary practice. Thus, the progression rates and to a lesser extent the recurrence rates published in the EORTC series should be similar to the untreated natural history of the disease, enabling one to determine the most important prognostic factors without having to take into account treatment as a confounding factor. One can, however, ask what the real value of these risk tables is in the treatment decision process because the positive predictive value (PPV) of the EORTC risk table for progression in high-risk patients is low, only 21%. This subject is discussed in more detail later in this article.
External Validation
Several groups have independently validated the EORTC risk tables. Fernandez-Gomez and colleagues performed an external validation in 1062 patients with NMIBC treated with maintenance BCG. For recurrence, Fernandez-Gomez and colleagues found a lower risk in each group of patients than Sylvester and colleagues, but the c index was comparable. For progression, lower risks were found in the cohort from Fernandez-Gomez and colleagues, especially in the highest-risk group at 5 years. The limitations as discussed by the investigators are the lack of re-TURBT and a short maintenance regimen. The investigators also mention the difference in the distribution of patients: there are more patients with aggressive tumor characteristics in this cohort than in the EORTC cohort. As mentioned in an editorial comment by Sylvester, application of the EORTC scoring system in the BCG series to predict progression yields a sensitivity of 88% and a negative predictive value (NPV) of 95%, but the PPV is only 17%. PPV is the proportion of positive test results that are true positive; thus, it reflects the probability that a positive test reflects the underlying condition being tested for. NPV is the proportion of subjects with a negative test result who are correctly diagnosed and, thus, without the investigated disease. Overall, this is a well-performed external validation, which shows that although the EORTC risk tables provided an adequate discrimination between patients with a different prognosis, their calibration was poor in patients treated with BCG.
Van Rhijn and colleagues validated the EORTC risk scores in 230 Dutch patients with primary NMIBC. Additionally, they proposed an alternative to pathologic grade with molecular grade (mG) based on fibroblast growth factor receptor 3 (FGFR3) gene mutation and MIB-1 expression. The median follow-up was 8.6 years. In general, 5-year recurrence-free survival (RFS) and progression-free survival (PFS) rates were lower in the cohort of Van Rhijn and colleagues than in the EORTC-cohort. According to the authors, the differences in PFS may be explained by the lower number of patients, by the selection of only primary patients, by the longer median follow-up, or because 32% of patients died of other causes. Furthermore, they found that mG was related to progression and disease-specific survival, and adding mG increased the predictive accuracy for progression from 74.9% to 81.7%. These data suggest a potential advantage to incorporating molecular markers into the EORTC risk score.
Sakano and colleagues validated the EORTC risk group stratification in 529 Japanese patients with NMIBC. The investigators concluded that the risk stratification as mentioned in the EAU’s guidelines is probably not applicable for Japanese patients but the subgroup classification on intermediate risk could be. Seo and colleagues compared recurrence and progression rates between the EORTC risk tables and their own cohort of 251 Korean patients. All recurrence rates of the Korean patients were lower than in the EORTC cohort, except for the 1-year recurrence rate in the intermediate-risk group, which was comparable with that of the EORTC cohort. In general, rates for progression in the Korean cohort were quite comparable with the rates in the EORTC risk tables despite the more aggressive patient and tumor characteristics of the Korean cohort. Hernandez and colleagues performed an external validation in 417 patients with primary NMIBC. In general, probabilities for both recurrence and progression in this cohort were higher than in the EORTC cohort. Their results validate the EORTC risk tables in terms of recurrence but not in terms of progression because of the low number of patients that progressed. Pillai and colleagues validated the EORTC risk model in 109 patients with primary and recurrent NMIBC. They found significantly higher 1- and 5-year probabilities of recurrence for all 4 groups compared with the EORTC model. However, it was not possible to draw firm conclusions about the validity because of the low number of patients in the individual groups.
In all, it is likely that the recurrence and especially progression rates reported by the EORTC risk tables are higher than those found in current clinical practice. As mentioned earlier, the progression probabilities and to a lesser extent the recurrence probabilities mentioned in the EORTC study are likely to be similar to the untreated natural history of the disease.
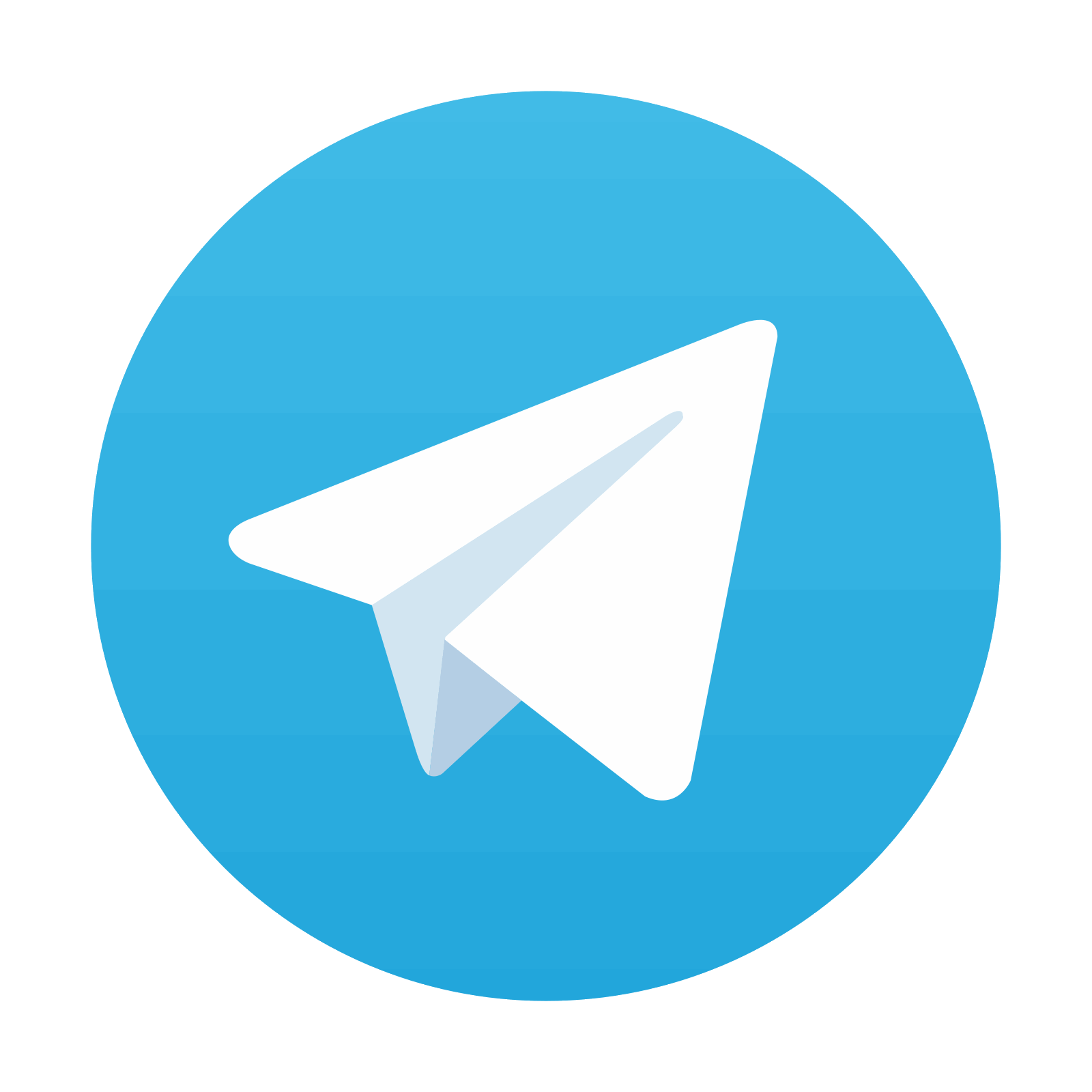
Stay updated, free articles. Join our Telegram channel

Full access? Get Clinical Tree
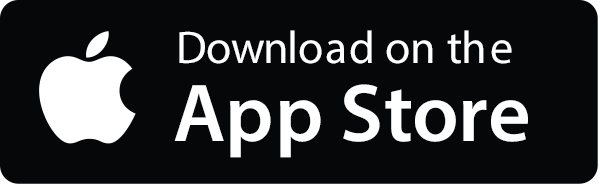
