Injuries have long been classified in terms of severity. The world’s oldest known surgical document, the Edwin Smith Surgical Papyrus (ca. 17th century BC), classified 48 traumatic injuries from ancient Egyptian battlefields and construction sites as successfully treatable, possibly curable, or untreatable.1 Such predictions about patient outcomes, and attempts to quantify the severity of injury, are today the realm of more than 50 published injury severity scores, scales, and models.
Injury severity scoring quantifies the risk of an outcome following trauma and provides metrics based on elements of clinical acumen and statistical modeling to describe aspects of the patient condition post-injury. The primary outcome of interest is commonly survival or a measure of morbidity (eg, complications) or resource use (eg, hospital or intensive care unit [ICU] length of stay [LOS]), but may be any primary endpoint of interest (eg, compliance to an evidence-based procedure).
The injury scores and models discussed here are generally applied using retrospectively collected data. They are therefore suitable for case mix adjustment or risk prediction in groups of patients but are not appropriate for individual patient predictions to guide clinical management decisions. They have a host of applications including quality improvements in patient care, trauma systems and health care delivery, injury prevention initiatives, and epidemiological studies of injury. Patient-specific clinical prediction applications are more simple, requiring decision rules to categorize patients into low-risk/high-risk “bins” and present less sophisticated statistical demand.
This chapter provides a concise description of injury severity scoring and modeling, with particular emphasis on application to outcomes research and quality improvement. Because there can be confusion about the precise meaning of terminologies used in this process, a few definitions are warranted to distinguish the qualitative from the quantitative.
Scores: Injury severity scores place numerical values on injury descriptions, thus enabling standardized communication about the injury state. Scores should be as objective as possible and their assignment should be supported by inter- and intrarater reliability studies.
Scales: Scales are a ranking, based on consensus, assessment, or mathematics and statistics, of risk or severity. Examples include the Glasgow Coma Scale (GCS), which ranges from 3 (deep coma) to 15 (full consciousness) and the Abbreviated Injury Scale (AIS) score, which generally ranks severity from 1 (minor) to 6 (virtually unsurvivable, Table 5-1).
Scales can be
Nominal: the values of the score are not numerical and have no order
Ordinal: the values of the score are not numerical but have an order (eg, increasing or decreasing injury severity)
Interval: the values of the score are numerical and the distance between the levels of the score is consistent both within and across the system of scoring
Models: Modeling is defined here as the use of scoring or scaling systems to create processes for determining probabilities of risk for specific outcomes (eg, mortality, morbidity). The process of modeling is a means of combining or integrating multiple attributes, for example, multiple injuries, or anatomy and physiology. Examples include logistic regression modeling to formulate coefficients of risk for specific elements within a scale, or risk associated with individual group diagnosis. Injury severity models quantify the risk of an outcome after trauma, for both clinical and research purposes.
Outcomes research: Outcomes research is defined as a method of creating “empirically verified information” to better understand how variables in the real-world setting (from injury to treatment) affect a wide range of outcome variables (from mortality to satisfaction with care).2
Risk adjustment: Risk, or case-mix, adjustment entails isolation of the effects to be studied from the effects of other “noisy” factors that can influence the outcome. Risk adjustment is essential for appropriate outcomes analysis. In injury outcomes research, severity scores are essential for risk adjustment because they allow accurate comparisons among disparate patient populations with varied degrees of risk. The goal is to compare populations with similar degrees of injury so that other risk factors (eg, time to treatment, mechanism of injury, injury prevention, and mitigation equipment) may be properly isolated to examine their relationship to particular outcomes. Risk adjustment might be as simple as defining classes of a variable to stratify risk groups or as complicated as using a risk adjustor in a multivariable regression model.3
Collecting reliable, accurate, and timely data on injury care is central to injury research and surveillance, and is the key to reducing the societal burden of injuries. Ideally, routinely collected data would be linked throughout the care continuum from prehospital care through community care. However, in most health care systems, injury datasets are limited to trauma registries and hospital discharge (administrative) databases.
One of the earliest civilian trauma registries was the Illinois Trauma Registry, which was started to benchmark and track improvements in trauma center implementation in a small geographic area.4 However, it was preceded by an “accidental” trauma registry comprising the Wound Data and Munitions Effectiveness Team (WDMET) data collected in Vietnam in 1967–1969. About 15 years later, the WDMET database was rescued from destruction by a young naval officer and has provided a basis for our understanding of combat injury and future combat trauma registries.5 The next significant step was the Major Trauma Outcome Study (MTOS),6 which collected data from more than 100 hospitals in North America and Canada, and serves as a model for all trauma registries established since the mid-1990s around the world.
Trauma registries are designed to provide information for quality improvement activities within trauma systems. They contain data on patient demographics, injury circumstances, prehospital care and transport (in some cases), emergency department (ED) and in-hospital interventions, anatomic injury, physiological measurements on arrival, complications, outcomes, and patient destinations. Some trauma registries also include information on comorbidities. The information is generally coded from patient charts by trained data abstractors and injuries are usually described using the AIS lexicon. One of the most complete trauma registries in the world is the Victorian State Trauma Registry (VSTR) in Australia, which provides 3-, 6-, and 12-month follow-up data on functional status for injury patients using an opt-out consent strategy that would not be possible in most jurisdictions.7
Trauma registries can be limited to a single provider but are most useful for quality improvement when they contribute to regional, state, or national registries. Trauma registries with a national reach include the US National Trauma Data Bank (NTDB), Canadian National Trauma Registry (NTR), UK Trauma Audit & Research Network (TARN), and the German TraumaRegister Deutsche Gesellschaft für Unfallchirurgie (TR-DGU). Because of its circumstances and integration of military and civilian medical systems, the Israeli Defense Forces (IDF) and Israeli trauma centers have a database that captures both civilian and military injuries, including those resulting from terrorist incidents. Trauma registry inclusion/exclusion criteria vary, with some registries including all injury admissions and ED fatalities, and some applying inclusion criteria based on injury severity, trauma team activation, or LOS.
The most common use of trauma registries is for benchmarking, whereby provider performance is monitored over time or compared to an internal or external standard to identify hospital outliers. Trauma registries are also used for resource allocation planning, injury prevention, and outcome research. Trauma registries most commonly used for research are the NTDB (238 PubMed citations in 2015), VSTR (45 citations), North Carolina Trauma Registry (14 citations), and the Québec Trauma Registry (QTR, 13 citations).
The major advantage of trauma registries is the fact that they include detailed clinical data, essential for accurate benchmarking and outcomes research. Disadvantages include (1) the fact that they are based on retrospectively collected patient chart data subject to data quality issues, (2) they only cover the index acute-care admission for injury, and (3) they are not population-based. Indeed, trauma registries are generally limited to patients treated in designated trauma centers, which can represent a small proportion of regional injury admissions, particularly in exclusive trauma systems. However, trauma registries based on mandatory data collection in inclusive trauma systems such as the VSTR or the QTR generally offer high population coverage (>90% of major trauma admissions in the QTR).8 Another important limit of trauma registries is the investment required in terms of efforts and resources. In 2004, the Victorian State Trauma Outcomes Registry and Monitoring (VSTORM) group estimated the cost of the VSTR at approximately A$100 (US $84) per patient.9 Despite the fact that this represents a fraction of a trauma patient’s health care costs, it is usually perceived as an onerous financial burden and is a major barrier to trauma registry implementation and maintenance.
Hospital discharge data are routinely collected in most high-income countries to meet administrative and reimbursement needs. These datasets generally include patient demographics, injury diagnoses, comorbidities, surgeries, complications, and injuries, coded using the International Classification of Diseases (ICD) lexicon. Advantages of hospital discharge data include population coverage of all hospital admissions and the fact that patients can be tracked through the system via a unique identifier, enabling researchers to obtain information on transfers, readmissions, and pre/post-injury use of health care services. Disadvantages include the lack of clinical information, the absence of injury severity scores, and omission of ED fatalities. In addition, discharge data are often geared to billing and may not include all injury diagnoses. Hospital discharge datasets are most useful when they can be linked to trauma registry data. Systems that enter shared data simultaneously into both databases (as in the QTR) are the most efficient.
A major challenge of data acquisition in trauma systems relates to prehospital clinical data. Few trauma registries capture these data, which, because of the emergency nature of prehospital intervention, are particularly hard to acquire and accurately record. For example, most prehospital providers do not ascertain patients’ GCS scores except by gestalt. Further, although they record interventions, abstracting and converting the information into a database is particularly challenging. This is an important hurdle because around half of all civilian trauma deaths occur in the prehospital period. This percentage is greatly increased in low- and middle-income countries (LMICs). Military data reveal that at least 90% of combat deaths occur prior to reaching a medical treatment facility (MTF)10 and yet the 75th Ranger Regiment instituted a process of complete capture of prehospital data for the decade they were involved in Afghanistan and Iraq.11 This is a fine example of prehospital data collection that influences the process of care, the speed with which it is rendered, and subsequent reduction in preventable deaths.
Other useful sources of injury data include emergency medical services (EMS) systems, police reports, rehabilitation facility databases, physician billing records, and community care databases. Unfortunately, there are considerable barriers to linking these data sources, such as lack of unique identifiers, data-protection acts, and lack of harmonization across datasets.12
The US Department of Transportation National Highway Traffic Safety Administration (NHTSA) houses two large injury databases related to vehicular crashes, based on linkage of multiple data sources. The Fatal Accident Reporting System (FARS) is a near-census population of deaths from vehicular injury in the United States, which contains data from police reports, EMS, and EDs. The National Accident Sampling System (NASS) is a sample of vehicle crash patient data obtained from prehospital and hospital sources throughout the United States. Both of these databases, although imperfect, have been populated for decades and are a valuable resource for tracking, among other information, prehospital and in-hospital deaths from vehicle crashes over the years (Fig. 5-1).
Trauma registries generally have rigorous data quality assurance programs to ensure the accuracy of data extraction from medical charts. However, the quality of information in the medical charts themselves remains an important problem. Examples of common data quality issues in trauma registries include underreporting of complications, underestimation of injury severity for early deaths, and missing data on physiological parameters such as the GCS and respiratory rate (RR). Many clinicians ignore these data quality issues and mistakenly believe that large numbers of patients in a dataset ensures quality. However, whatever the sample size, systematic variations in data quality across providers can lead to selection or information bias, whereby observed differences in performance across injury groups is due to differences in coding practices rather than quality of care. These data quality problems can be partly addressed in the analysis phase through sensitivity analyses and strategies to simulate missing data (eg, multiple imputation). However, analytical solutions will never replace high-quality data, and every effort should be made to ensure the accuracy of collected data.
Trauma researchers must be familiar with injury scoring schemes to accurately risk-adjust injury group comparisons in an attempt to isolate the effects of an independent predictor variable on a dependent outcome variable. Four types of severity scores are typically used: (1) anatomic injury scores, (2) physiological scores, (3) comorbidity scores, and (4) combinations of the three and other factors. The scores vary considerably in complexity of calculation and ease of use. Injury severity score selection should be based on a clear sense of what one wants to measure and why, and a good understanding of their strengths and limitations.
Anatomic injury classification systems, scores, and models describe the site of injury and extent of damage. Many anatomic scores have been proposed in the literature, but this review is limited to general scores, scales, and models that have gained practical acceptance. We will not review the huge number of specialty classification and grading systems such as the AO Foundation/Orthopaedic Trauma Association (AO/OTA) Classification of Fractures and Dislocations. Most general scoring algorithms are designed to predict mortality (Table 5-2) and are not specifically validated for other outcomes, such as LOS or functional status, although moderate correlations may exist. The majority of scores are based on either the trauma-specific AIS coding classification or the more general ICD-9-CM taxonomy. Injury scoring and modeling systems are continuously being revised, tested, and compared to each other, and no consensus has been reached on which is best. It is likely that the optimal scoring system is contextual and therefore depends on the research question at hand and on the population under study.
Name (year first introduced) | Predicts what? | How calculated? | Score range/interpretation | Benefits | Limitations |
---|---|---|---|---|---|
Anatomic scoring and modeling systems | |||||
ISS (1974) | Patient survival | Body divided into six anatomical regions: head/neck, face, chest, abdomen/pelvis, extremities, skin/general; injuries in each region given an AIS score; the highest AIS scores in the three most severely injured regions are squared and summed for ISS; any patient with an AIS severity of 6 is automatically given an aggregate ISS of 75 | 44 Distinct possible scores ranging from 1 (least severe) to 75 (unsurvivable); higher scores reflect higher likelihood of mortality | Most widely used trauma severity score; relates AIS to patient outcomes | No physiologic predictors; does not account for >1 injury in the same region, only the highest score; scored on three regions only, not all six; requires specialized training. Obsolete |
NISS (1997) | Patient survival | Sum of the squares of the three most severe AIS injuries, regardless of body region; as with ISS, any patient with an AIS severity of 6 is automatically given an aggregate NISS of 75 | 44 Distinct possible scores ranging from 1 (least severe) to 75 (unsurvivable); higher scores reflect higher likelihood of mortality | Addresses the ISS omission of multiple occurrences of serious injuries within one body region; slight prediction advantage over ISS and ICISS in predicting mortality | Scored on three injuries only; requires specialized training; not widely used but should be |
AP (1990) | Patient survival | Three “modified components” (head/brain and spinal cord injury; thorax and neck injury; all other serious injuries) are scored based on AIS and weighted to form a single APS; only “serious” injuries included | Range varies; higher numbers have worse prognosis | Logical, analytical approach; based on location and severity of injury | Failed to supplant ISS as predominant severity scoring modality |
ICISS (1996) | Patient survival | Computed directly from ICD-9 codes; conversion of a specific ICD-9 code into a Ps for that code, with higher Ps reflecting higher likelihood of survival; the final ICISS is the product of each Ps for each injury | Scores range from 0 (unsurvivable) to 1 (100% likelihood of survival) | One of the few severity scores not based on AIS/ISS; accounts for all injuries; no specialized training required; multiple studies show it outperforms ISS; some evidence that most severe Ps outperforms multiplicative Ps | Multiple databases can be used to calculate SRRs (ICD-9-CM, NTDB, etc), and thus SRRs are database-specific; SRRs from different sources are not comparable; SRRs are not independent of each other; no consensus yet on best practice; some argue ICISS measures hospital survival not injury survival. Not widely adopted |
ICD-MAP-90 (1997) | Injury severity | Translates ICD-9 discharge diagnosis codes into AIS85 “pre-dot” codes, injury descriptors, and severity scores, which in turn are translated into an ISS, NISS, and APS for the patient | ICD/AIS scores vary | Conservative estimates of injury severity | Based on a computer software program; loss of certain injury information in the translation between diagnosis systems |
TRAIS (2003) | Patient survival | Exact same calculation as ICISS except TRAIS uses AIS injury descriptor codes to create Ps; the TRAIS is the product of AIS-derived Ps for each injury | Scores range from 0 (unsurvivable) to 1 (high likelihood of survival) | Behaves very similarly to ICISS; out predicts its AIS counterparts ISS, NISS, and APS; used in models to predict mortality | Not widely adopted |
OIS (1987) | Not for patient outcomes | Anatomic injury within an organ system graded on an ordinal scale, with Grade 1 being a minor injury and Grade 5 being tissue-destructive and likely fatal | Designed to standardize the descriptive language of injury for 32 organ and body system regions | Enhances communication between trauma surgeons | No predictive abilities; not widely adopted; not used for risk adjustment |
Physiological scoring systems | |||||
GCS (1976) | Patient survival; brain function | Aggregate score of motor activity (scale of 1–6 points), verbal activity (1–5 points), and eye-opening (1–4 points); higher scores indicate more function | Range from 3 (no or minimal neurological function) to 15 (normal or near-normal neurological function) | Simple to use; quickly calculated; well validated; used by many other scoring systems; can use motor score alone | Hard to measure all components in some patients (if sedated, intubated, etc); score changes with time (not fixed on admission) |
RTS (1989) | Patient survival | Computed by logistic regression equation based on indexed values of GCS, SBP, and RR on ED presentation | Range from 0 (severe physiological derangement) to 7.84 (no physiological derangement) | Provides physiological assessment of patient; high association with mortality; contributes to TRISS | Intubation and sedation prior to ED arrival alter accuracy; use limited by missing data |
Combination scoring systems and models | |||||
TRISS (1987) | Patient survival | Combines ISS, RTS (RR, SBP, GCS) and age; regression coefficients derived from MTOS database; equations vary between blunt vs penetrating trauma | Scores range from 0 (unsurvivable) to 1 (high likelihood of survival | Useful for quality improvement initiatives; separate probability equations for blunt and penetrating patients | Requires multiple variables; if one is not captured, TRISS cannot be calculated |
ASCOT (1990) | Patient survival | Uses APS to define injury severity, with different regression coefficients for blunt and penetrating trauma | Range varies | Outpredicts TRISS for penetrating trauma | Complex calculation |
TRISSCOM (2004) | Patient survival | Similar to TRISS with adjustments to age (dichotomized at 65 years old as opposed to 55 years old) and the addition of eight comorbidity variables (recorded as binary yes/no variables) | Scores range from 0 (unsurvivable) to 1 (high likelihood of survival) | Reflects the aging population | Comorbidities are not weighted based on severity |
Kampala Trauma Score (1996, revised in 2004) | Patient survival | Combines number of injuries, RTS and ISS, using anatomic and physiological data to create a score similar to TRISS but simplified for use in sub-Saharan Africa | Scores range from 0–6 (severe), to 7–8 (moderate) to 9–10 (mild) | Simplified for use in resource-poor countries | Does not account for comorbidities or blunt trauma |
HARM (2000) | Patient survival | Based on ICD-9-CM-coded hospital discharge data | Scores range from 0 (no probability of death) to 1 (no probability of survival) | Simpler to use than TRISS | Considers only anatomic, not physiologic data |
TRAM (2009) | Patient survival | An alternative to TRISS that combines anatomic injury (AIS), physiologic response (GCS, SBP, RR), physiological reserve (age and comorbidities), and transfer status | Scores range from 0 (no probability of death) to 1 (no probability of survival) | Flexible modeling techniques are used over categories so all information on quantitative variables (age, GCS, SBP, RR) is used, Interaction term with transfer status | Complex spline functions require statistical expertise |
The AIS was first conceived as a system to define the type and severity of injuries arising from aircraft and motor vehicle crashes.13 It began with a dictionary of 73 injuries in 1971 and now has more than 2000, adding to its precision but also to its cost and complexity. Major revisions to the AIS occur every few years, (eg AIS85, AIS90)14,15 with a new update in 2015. To calculate AIS scores, medical records are transcribed into specific codes that capture individual injuries. The AIS is a proprietary classification system requiring specialized training for coding personnel. Because of this, AIS is not captured at every hospital.
AIS divides the body into nine regions: head, face, neck, thorax, abdomen, spine, upper extremities, lower extremities, and external. The actual AIS code consists of two numerical components separated by a decimal point. The first is a six-digit injury descriptor “pre-dot” code, which classifies the injury by region, type of anatomic structure and specific structure injured, and level of injury. The “post-dot” component is a severity score ranging from 1 (minor) to 6 (virtually unsurvivable), as shown in Table 5-1. AIS severity scores are consensus-derived assessments assigned by a group of experts. The maximum AIS (MAIS), which is the highest AIS severity among all of a patient’s injuries, is often used to quantify a patient’s injury severity. This score is highly correlated with mortality but ignores information on concomitant injuries.16
AIS has many intrinsic shortcomings, including anatomically incorrect body regions, inability to code combat injuries,17,18 and inconsistent scaling of severity across body regions.19 For example, an AIS 3 in the abdomen is associated with a higher probability of mortality than an AIS 3 in the chest or head. Even when performed by experts, AIS coding has low interrater reliability and 65–82% of AIS codes are not used, even in the largest registries.20 Further, the limitations of the AIS are carried forward and multiplied when they are used as the basis for models (eg, the Injury Severity Score [ISS], discussed below).21 Redactions of AIS are now available for both civilian and military injury databases.
Owned by the World Health Organization, the International Classification of Diseases (ICD) is not injury specific, but is a general, all-purpose diagnosis taxonomy for all health conditions. It is well over a century old and is currently in its 11th clinical modification (ICD-11). In most countries, the tenth clinical modification, ICD-10, is being used.22 However, in the United States, ICD-9-CM is the standard for coding hospital diagnoses including injuries.23 In the ICD-9 lexicon, ICD codes exist for more than 10,000 medical conditions, about 2000 of which are physical injuries (the block of ICD-9-CM codes from 800.0 to 959.9 encompasses traumatic injuries). For trauma researchers, AIS codes are generally preferred over ICD-9 because of their greater specificity of injury description (the pre-dot classification) and the availability of an injury severity classification (post-dot code).
In 1987, the American Association for the Surgery of Trauma (AAST) introduced the Organ Injury Scale (OIS).24 The goal of the scale was not to predict outcomes, but to standardize the descriptive language of injuries to improve communication between trauma surgeons and other physicians. Like the AIS, the OIS provides an ordinal scale to each level of organ disfigurement, ranging from grade 1 (relatively minor) to grade 5 (likely fatal). As such, it emulated similar efforts by orthopedic surgeons to classify fractures that go back decades. These scales exist for 32 organ and body region systems.25,26,27,28,29,30 The OIS is rarely used in modern injury outcome research.
The Barell Injury Diagnosis Matrix is a matrix of ICD-9-CM codes for classifying injury diagnosis by type and anatomic region into “injury profiles.”31 The Barell Matrix enables epidemiologic, management, and clinically oriented analysis by serving as a standard for case-mix comparison and characterization of injury patterns. The matrix consists of 12 columns based on ICD-9-CM sequence representing nature of injury (eg, fractures, amputations), and rows with varying levels of detail relating to body region (eg, head and neck, traumatic brain injury [TBI]). Using this matrix, data can be aggregated into summary reports that indicate injury distribution, enabling descriptive comparisons.
Current anatomic injury models are based on the AIS or the ICD, and may also be based on injury scores derived by expert consensus (AIS injury severity score) or derived empirically (probabilities of mortality calculated using large injury databases). AIS-based consensus models include the Injury Severity Score (ISS), the New Injury Severity Score (NISS), and the Anatomic Profile (AP). AIS-based empirical models include the Trauma Registry Abbreviated Injury Score (TRAIS) and the Trauma Mortality Prediction Model (TMPM). ICD-based models are all derived empirically and include the ICD Injury Severity Score (ICISS) and its derivatives, as well as the OIS.
In 1974, Baker et al first posited a multi-injury score by introducing the Injury Severity Score (ISS).32 Injuries in each AIS region are given an AIS score and the highest AIS scores in the three most severely injured regions are squared and summed to form the ISS. The ISS range is an ascending scale of severity from 1 (least severe) to an assigned 75 (originally designated as unsurvivable). AIS 6 automatically translates to ISS 75.
ISS correlates with mortality and remains for researchers the most widely used anatomical scoring system. However, ISS has many limitations.33 Its association with the probability of mortality is neither smooth nor monotonic. Further, it only considers one injury in each body region and thus ignores important injury information, especially in penetrating and ballistic injury (Table 5-3).18,34 Because of these shortcomings, we continue to believe ISS should be retired and replaced by one of the more modern injury models now available (see below).
Body region | Percent mortality |
---|---|
Head and neck | 17.2% |
Abdomen | 10.5% |
Thorax | 6.1% |
Face | 0% |
Pelvis | 0% |
The New Injury Severity Score (NISS) was formulated by Osler et al to address ISS shortcoming in quantifying multiple occurrences of serious injuries within the same body region.36 NISS is the sum of the squares of the three most severe AIS severities, regardless of body region and, as with the ISS, AIS 6 automatically translates to NISS 75. This permutation offers a slight predictive advantage but has several of the same shortcomings as the ISS.
The Anatomic Profile (AP), developed by Sacco et al, uses AIS severity scores with an adjustment for differences across body regions.18 Three modified components are weighted to form a single scalar based on anatomic location of all serious injuries (AIS >3). The AP overcomes many modeling defects inherent in ISS and NISS and avoids the excessive complexity and nontransportability in scores such as the TMPM.37 The fact that the AP has not supplanted the ISS, however, is probably a reflection of the general lack of understanding of injury severity modeling in the injury research community.
The ICD Injury Severity Score (ICISS), created by Osler et al, took an empirical estimation approach to injury severity scoring with the formulation of ICD-9 survival probability (Ps).38 Ps is an ICD-9 code-specific estimate of the survival probability associated with that particular injury. For a set of patients, Ps for a particular injury code is the number of patients who survive that injury divided by the number of patients who display the injury. The traditional ICISS is calculated as the product of Ps for as many as 10 injuries, and ranges from 0 (unsurvivable) to 1 (high likelihood of survival). Other versions of the ICISS include Ps of the worst injury, and independent Ps calculated on patients with isolated injuries. A similar approach was used in the 1970s, with each ICD code being given a conditional and definitive Ps related to the presence of other injuries.39
ICISS offers several advantages over other anatomic scores. First, because it is based on the ICD coding lexicon, it can be used in any clinical setting, including smaller centers that typically do not perform AIS coding. Second, unlike the consensus-derived AIS severity scores, ICISS’ empirical approach enables powerful statistical estimates of injury-specific survival if the patient population used to derive them is large enough. Consequently, unlike ISS and NISS, ICISS is a smooth, if nonlinear, function of mortality.
ICISS does, however, have limitations. First, although it resembles an overall probability, ICISS can only be considered a scalar because Ps is often “contaminated” by patients with multiple injuries. Independent Ps can be calculated as mentioned earlier, but these are not available for all codes because many injuries rarely occur in isolation.40 Second, Ps is database-specific and the degree to which it is applicable in injury populations with different patient characteristics remains uncertain.41 Finally, because empirically based injury severity scores are based on observed all-cause mortality, the ICISS is not an independent measure of anatomical injury but inherently incorporates physiological reserve and physiological reaction to injury, which vary across injury diagnoses.
ICD-9 codes are nominal, meaning they are unordered, qualitative categories not ranked by severity. If one ignores the AIS severity score, AIS codes can also be treated nominally, taking advantage of their specificity in injury classification. As such, AIS injury descriptor codes can be used to calculate Ps, similar to the ICISS. The Trauma Registry Abbreviated Injury Score (TRAIS) is the product of AIS-derived Ps. Kilgo et al showed that ICISS and TRAIS behave similarly in a large group of patients coded both ways and that TRAIS predicts mortality more accurately than its AIS counterparts ISS, NISS, and AP.42 Although ICISS and TRAIS are derived from two different coding systems, they behave similarly in terms of their association with mortality. This suggests that empirical approaches might obviate the inherent structure of the coding systems.
The Trauma Mortality Prediction Model (TMPM) is also based on Ps associated with AIS codes, generated using NTDB data. It adds a level of complexity as it is calculated as a weighted sum of coefficients from two probit models of mortality: one based on AIS pre-dot codes and one on body region injured.
To address the lack of an injury severity scoring mechanism in the ICD classification system, MacKenzie and colleagues developed an algorithm to convert ICD-9-CM codes to AIS90 codes.43 The algorithm has more recently been updated to map ICD-10 codes to AIS98 codes.44 Mapped AIS codes can be used to calculate AIS consensus-based severity scores including the ISS, NISS, and AP. This algorithm has proven useful for quantifying injury severity in administrative databases but mapping is not achieved for all ICD codes, and the performance of mapped AIS injury severity scores for predicting mortality is significantly inferior to directly coded AIS severity scores and ICD-based scores (ICISS).16,45
Trauma clinicians, outcomes researchers, and hospital administrators may ask which of these approaches is best. There is no consensus, and many publications each year continue to debate this question.
Several large studies, including ones by Sacco et al and Meredith et al, compared these anatomic scores in terms of their ability to predict mortality.16,46 Both studies found that AP and ICISS better discriminate survivors from nonsurvivors than ISS, NISS, and the ICD-MAP versions of ISS, NISS, and AP. A surprising finding was that MAIS performed better than its multi-injury counterparts ISS and NISS. Based on this result, Kilgo et al showed that the patient’s worst injury, regardless of coding lexicon (ICD-9 or AIS) or estimation approach (AIS severity-consensus or empirical Ps), was a better predictor of mortality than multi-injury scores.42 Harwood et al. reported that the NISS was better than the ISS and equivalent to the MAIS in the prediction of mortality in blunt trauma patients.47
However, many of these studies have not accounted for other risk factors inherently incorporated into empirical scores (age, comorbidities, physiological reaction). Studies that do not account for these risk factors when comparing consensus-based and empirical scores unfairly disadvantage the former.48 As mentioned earlier, each of these scores has strengths and limitations, and the choice of score should be adapted to the outcome, data, and study population at hand.
Anatomic injury unleashes a set of physiological and bio-chemical consequences that require modulation and mitigation as part of the patient’s treatment. The concept of integrating physiology into injury severity modeling recognizes the dynamic and time-dependent changes to physiological status following injury. A patient with a ruptured spleen who is seen within 1 hour of injury might be normotensive and, when appropriately and promptly treated at a trauma center, will have a very low risk of dying. A patient with the identical anatomic injury who is seen 4 hours later, for whatever reason, may present with a systolic blood pressure (SBP) of 60 mm Hg and a significant risk of death despite availability of the same system of in-hospital care. The hospital should not be penalized if the patient dies (but perhaps the system should). Integration of physiological parameters on arrival with other data is therefore essential for accurate case mix adjustment and outcome prediction in injury research.
Early death following injury occurs as a result of central nervous system (CNS) impairment, hemorrhage, or respiratory causes or combinations of causes. Clinical markers, including RR, SBP, base deficit, and reaction to stimuli/state of consciousness are important prognosticators of outcome and are routinely used in clinical management. However, unlike anatomic injuries and preexisting comorbidities, which are fixed at the time of hospital admission, physiological parameters are ever-changing, both spontaneously and in response to therapy. Thus, it is necessary to obtain a “snapshot” of physiological status at one point in time, usually immediately upon ED or trauma center arrival. The main physiological scores currently in use in injury research include the GCS and the Revised Trauma Score (RTS).
The GCS was first proposed by Teasdale & Jennett as a means to directly triage brain-injured patients and to monitor postoperative craniotomy patients.49,50 The GCS was subsequently integrated into the Trauma Score/Revised Trauma Score (RTS) and Triage Score to describe level of consciousness without using subjective terms such as “semicomatose” and “lethargic” to classify head injury following trauma.51 GCS measures brain function via three components: (1) motor (GCS-M), (2) verbal (GCS-V), and (3) eye opening (GCS-E), each with ordinal characterizations of severity (Table 5-4). The scale ranges from 3 (completely unresponsive) to 15 (fully conscious) and the GCS score has been shown to be strongly correlated with survival.52 The motor component alone has been shown to be almost as powerful as the full GCS score,53 and could theoretically replace the full GCS score for predicting mortality. However, the verbal and eye components discriminate noncomatose patients and are thus valuable for predicting nonfatal outcomes.54
Function | Description | GCS scaled value |
---|---|---|
Eye | Spontaneous | 4 |
To voice | 3 | |
To pain | 2 | |
None | 1 | |
Verbal responses | Oriented | 5 |
Confused | 4 | |
Inappropriate | 3 | |
Incomprehensible | 2 | |
None | 1 | |
Motor response | Obeys commands | 6 |
Localizes pain | 5 | |
Withdraws (pain) | 4 | |
Flexion | 3 | |
Extension (pain) | 2 | |
None | 1 |
The Trauma Score, later updated to the Revised Trauma Score (RTS), was designed by Champion et al as an approach to combining clinical and observational physiological data into one score.55,56
Two forms of the RTS exist, one for triage (Triage-RTS) and one for outcomes evaluation and risk adjustment (RTS). Both are based on the GCS, SBP, and RR (Table 5-5). The Triage-RTS is calculated by summing the coded values for each of the three variables, and ranges between 0 and 12. The RTS equation for outcomes evaluation computes indexed values of GCS, SBP, and RR by weighting their coded value with logistic regression coefficients and summing them.
The RTS ranges from 0 to 7.84, with lower scores translating into more physiological derangement. RTS correlates strongly with mortality57 and remains important in injury scoring through its contribution to the TRISS model (see below). Studies have also shown that the combined use of SBP and GCS-M are just as effective at predicting patient survival as the RTS.58 Disadvantages of the RTS include the fact that coefficients are based on MTOS data and have not been updated, and that categories of the GCS, SBP, and RR used to calculate the RTS often have very sparse data.57
Injury outcomes research has long recognized the importance of comorbidities to patient risk and outcomes. For that reason, comorbidities were integrated into the American College of Surgeons Committee on Trauma (ACS-COT) field triage decision scheme developed by Champion.59 Morris et al, among others, identified several preexisting conditions that worsen prognosis following trauma, most notably liver cirrhosis, chronic obstructive pulmonary disease (COPD), congenital coagulopathy, diabetes, and congenital heart disease.60 Morbid obesity has now been added to this list.61 The incorporation of preexisting conditions into injury severity models is difficult because so many potential comorbidities exist, each of which may occur with variable severity. Further, many are relatively rare and may be inconsistently recorded.
Specific comorbidity adjustments, such as the Charlson Comorbidity Index (CCI), which are widely used in other disciplines,62 are frequently used in injury severity models in attempts to enhance their predictive abilities. Results, however, have been poor.63,64 This may be because such scores are not adapted to acute injury populations.64 Indeed, the CCI, a weighted sum of 17 preexisting conditions, is based on coefficients derived in a population of cancer patients using a Cox proportional hazards model and is therefore clearly not appropriate for injury admissions. The number of Charlson comorbidities has been shown to predict injury mortality as well as the CCI.64 Other approaches include using the presence of individual comorbidities or classes of conditions (ICD-9-CM ranges) in risk-adjustment methods or simply using patient age as a surrogate for comorbidities. With the aging of trauma populations, comorbidity and multimorbidity will increase, and accounting for these factors in injury research will become increasingly important. Efforts should therefore be made to develop an injury-specific comorbidity index.
Since the addition of descriptors for coding penetrating injuries with the AIS 1985 edition, researchers have had a tool for evaluating both blunt and penetrating injuries. The descriptors of penetrating injuries included in AIS versions since 1985 describe low-kinetic-energy injuries treated in civilian trauma centers and hospitals. Subsequent iterations have been used to code military combat injuries as well.65 These codes, however, did not adequately describe commonly seen penetrating combat injuries such as multiple and massive soft-tissue fragment wounds; high velocity penetration; blast overpressure injuries (mutilating or nonmutilating), and/or bilateral and multiple injuries17,18 that result from explosive devices including improvised explosive devices (IEDs), which account for 55–75% of combat injuries.10 They also did not account for, nor were they designed to, some of the injury phenomena associated with mass casualty incidents, for example, crush injuries in earthquake disasters.
To address these issues, a committee of military physicians was formed to work with the International Injury Scaling Committee (IISC) of the Association for the Advancement of Automotive Medicine (AAAM) to propose guidelines for developing a version of the AIS specifically for coding combat injuries. These physicians represented all three services of the US military (Army, Navy/Marines, Air Force) as well as a spectrum of medical specialties relevant to combat casualty care including emergency medicine and trauma, orthopedic, neurosurgery, and general surgery. AIS 2005-Military is used for coding of all injuries in the three combat trauma registries: (1) the DoD Trauma Registry (DoDTR, formerly the Joint Theatre Trauma Registry [JTTR] based in San Antonio, TX; (2) the Navy/Marine Combat Trauma Registry (CTR) Expeditionary Medical Encounter Database (EMED) based in San Diego, CA; and (3) the Mortality Trauma Registry (MTR) based at the Office of the Armed Forces Medical Examiner (OAFME) in Rockville, MD. These registries also code in AIS 2005 (civilian version) and AIS 1998 for future comparisons with civilian trauma registry data.
Development of AIS 2005-Military coincided with the revision efforts that would culminate in publication of the civilian AIS 2005, which included additional expanded descriptors for orthopedic trauma based on the OTA scale and expanded bilateral injury codes, particularly for vessel injuries. The same consensus model used in determining changes to each injury description by the IISC was used to determine AIS 2005-Military scores.
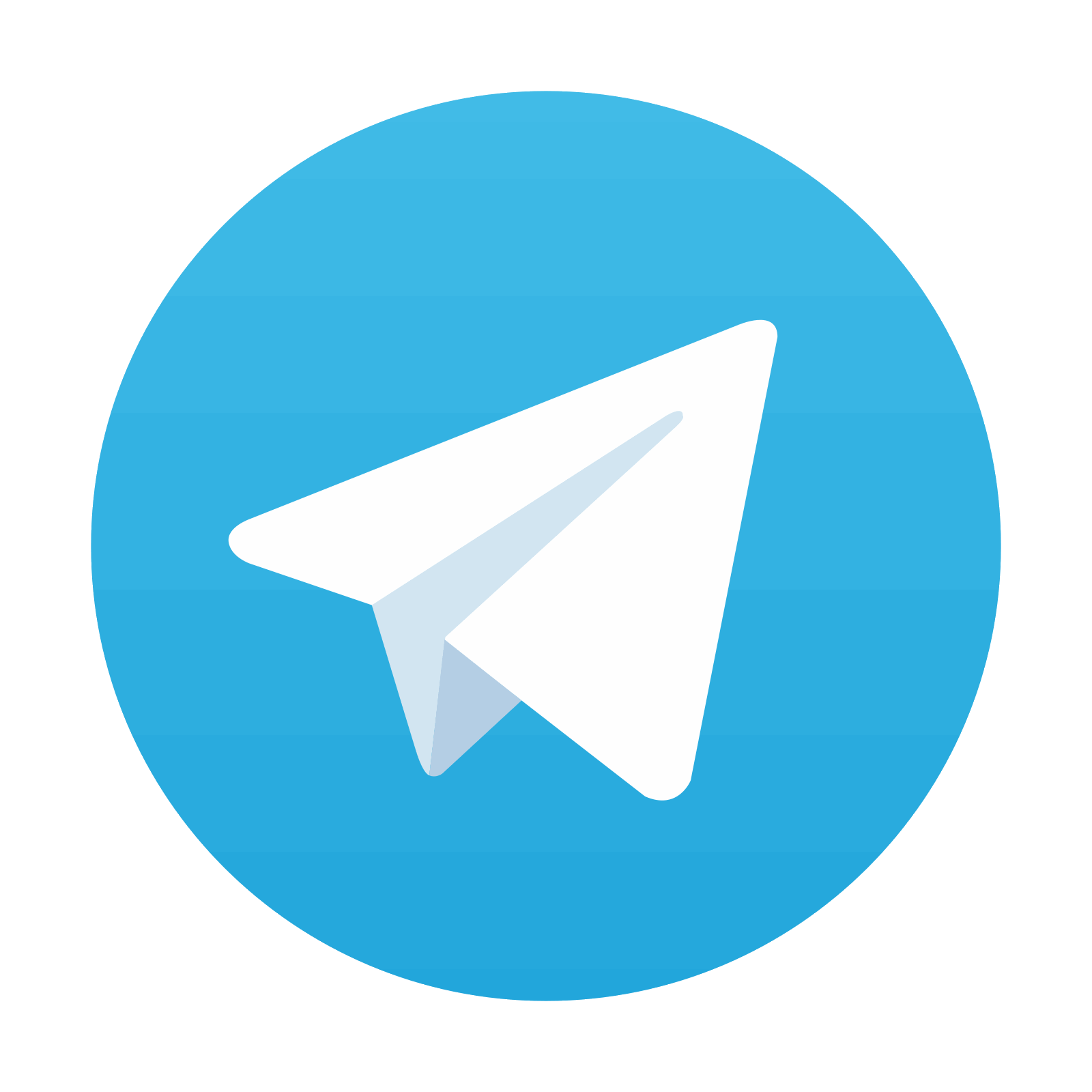
Stay updated, free articles. Join our Telegram channel

Full access? Get Clinical Tree
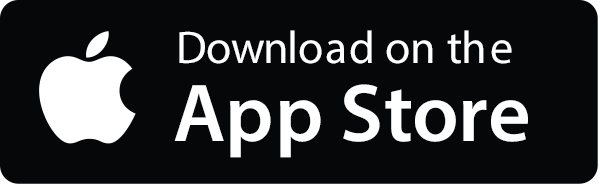
