In the preceding two decades, several milestones have been reached in the management of patients with metastatic renal cell carcinoma (mRCC), including the development of novel targeted agents paralleling an increased understanding of the molecular biology of this disease process. Recently, a renewed enthusiasm for immunotherapy in the form of immune checkpoint blockade has resulted in significant strides in the treatment of mRCC. Despite these advances, treatment remains challenging for clinicians, and only modest survival benefits are observed with current treatment paradigms. The risk-stratification tools and investigated predictive and prognostic biomarkers in patients with mRCC are detailed in this review.
Key points
- •
Renal cell carcinoma (RCC) is the most lethal urologic malignancy and is the eighth leading cause of cancer in the United States, comprising 3% of adult malignancies. The 5-year survival of patients with metastatic disease is approximately 12%.
- •
Distinct clinical variables were found to associate with prognosis, and identifying patients likely to derive benefit from specific therapies or cytoreductive strategies posed a significant clinical challenge.
- •
Prognostic models were developed from these identified clinical factors that were predicated upon disease burden, tumor, and patient-related factors. The most widely used models include the Memorial Sloan Kettering Cancer Center risk model and the International Metastatic RCC Database Consortium model.
- •
Biomarker development in metastatic RCC is an emerging field of research; the most widely studied biomarkers include prognostic models as predictive biomarkers, genomic and gene-expression based biomarkers, and molecular and immunohistochemical biomarkers.
- •
Advances in prognostication and biomarker development will be aided by further insight into the biological processes underlying the development of metastatic RCC and the development of powerful biomarkers validated for use in routine clinical practice.
Introduction
Renal cell carcinoma (RCC) accounts for 3% of adult malignancies and is the eighth leading cause of cancer in the United States. Worldwide, 400,000 people were diagnosed and 175,000 people died of kidney cancer in 2018. Up to 30% of patients diagnosed with RCC present with synchronous metastases, and recurrence is seen in 30% of patients after complete resection of the primary tumor. , Although the 5-year survival of early-stage RCC is 93%, patients presenting with metastatic disease have dismal 5-year survival rates of approximately 12%, and at least half of patients with RCC will eventually require systemic therapy. RCC is generally considered resistant to conventional cytotoxic chemotherapy and radiotherapy but has classically been regarded as an immunogenic tumor as evidenced by occasional spontaneous regressions and mild to moderate success with prior immunotherapeutic approaches.
Before 2005, the only approved treatment of metastatic renal cell carcinoma (mRCC) was high-dose interleukin-2, which exhibited durable responses in approximately 5% to 10% of patients at the cost of severe acute toxicities as a result of the proinflammatory cytokine storm induced by treatment. Low-dose subcutaneous interferon (IFN) was commonly used instead, given its somewhat better safety profile, although durable responses were uncommon. After 2005, the treatment landscape for mRCC shifted from immunotherapeutic approaches to approaches directed at growth factors overexpressed in RCC downstream of altered von Hippel–Lindau ( VHL ) function, particularly vascular endothelial growth factor (VEGF). Several multitargeted tyrosine kinase inhibitors with activity against the VEGF receptor, antibodies directed against VEGF, and small molecule inhibitors of mammalian target of rapamycin were explored in mRCC leading to Food and Drug Administration approval and integration as standard therapies. Despite the plethora of such therapies introduced to treat mRCC, and the convenience of oral administration offered by many of these drugs, chronic administration is required, adverse effects can substantially impact quality of life, and the vast majority of patients ultimately experience disease progression despite treatment.
In just a few years since entering the clinic, immune checkpoint blockade (ICB) has dramatically changed the landscape of treatment of mRCC and has secured a place as a standard pillar of treatment. Despite these successes and a renewed enthusiasm for immunotherapy, mRCC still carries a poor prognosis and remains unpredictable with a highly variable clinical course, spanning from indolent to rapidly progressing disease. Because distinct clinical variables were found to associate with prognosis, identifying patients likely to derive benefit from specific therapies or cytoreductive strategies posed a significant clinical challenge. Indeed, prognostic models were developed from these identified clinical factors, which were predicated upon disease burden, tumor, and patient-related factors. In this review, we outline investigated biomarkers and the risk assessment tools developed to better inform therapeutic decisions and accurately prognosticate patients with mRCC.
Risk-stratification models
Prognostic models are critical components of clinical trial design, risk-directed therapy, and patient counseling. Prognostic factors differ from predictive factors in that prognostic factors associate with progression and survival of patients, whereas predictive factors refer to the effects of therapeutic agents to the tumor or host. An observation first noted during the cytokine era, prognosis in mRCC varies with the involved target organ with liver and bone involvement portending worse survival compared with lung and regional lymph node metastasis.
One of the first and most widely used prognostic models, the Memorial Sloan Kettering Cancer Center (MSKCC) model, reported by Motzer and colleagues , used pooled data from patients in clinical trials treated with IFN-alpha accrued from 1982 to 1996 to identify 5 prognostic factors. These factors included Karnofsky performance status (KPS; <80%), serum lactate dehydrogenase (LDH; >1.5× upper limit of normal [ULN]), hemoglobin level (< lower limit of normal), level of corrected serum calcium (>10 mg/dL), and time from initial RCC diagnosis to start of therapy of less than 1 year. Using this model, patients stratified in the favorable-risk group have zero factors (median overall survival [OS], 29.6 months); patients stratified in the intermediate-risk group have one or 2 risk factors (median OS, 13.8 months), and patients stratified in the poor-risk group have 3 or more risk factors (median OS, 4.9 months). In a validation study, Mekhail and colleagues added prior radiotherapy and number of metastatic sites the MSKCC model, terming this extended model the Cleveland Clinic Foundation (CCF) model.
The shift from the cytokine era to the targeted therapy era was accompanied by a need for updated prognostic models and survival estimates. Using a cohort of 645 patients with mRCC treated with sunitinib, sorafenib, and IFN-alpha plus bevacizumab, Heng and colleagues developed a new prognostic model termed the International mRCC Database Consortium (IMDC) model. Four of the 5 MSKCC criteria were found to be prognostic; LDH was not significantly associated with survival in this model. Two additional factors, neutrophilia (> ULN) and thrombocythemia (> ULN), were independently associated with survival. The IMDC model was externally validated in a cohort of patients treated with first-line VEGF-targeted therapy and compared with other prognostic models, including MSKCC, CCF, the International Kidney Cancer Working Group (IKCWG), and Groupe Français d’Immunothérapie. Patients in the favorable-risk group have zero factors with a median OS that was not reached (43.2 months in the external validation cohort); patients in the intermediate-risk group have one or 2 risk factors (median OS, 27 months), and patients in the poor-risk group have 3 or more risk factors (median OS, 8.8 months).
Over a period of 6 years, the Groupe Français d’Immunothérapie enrolled 782 mRCC patients treated with cytokine regimens to identify prognostic factors for survival, demonstrating that performance status, number of metastatic sites, disease-free interval, biological signs of inflammation, and hemoglobin levels can be considered validated prognostic factors. Four independent factors predictive of rapid progression in patients treated with cytokine therapy were likewise identified, including the presence of hepatic metastases, short interval from renal tumor to metastases (<1 year), more than 1 metastatic site, and elevated neutrophil counts. Patients with at least 3 of these factors have greater than 80% probability of rapid progression despite treatment.
The IKCWG model used a database of 3748 patients pooled from clinical trials and was validated using an independent data set of 645 patients treated with tyrosine kinase inhibitors. Nine clinical factors were identified as prognostic, including prior treatment, performance status, number of metastatic sites, time from diagnosis to treatment, pretreatment hemoglobin, white blood cell count, LDH, alkaline phosphatase, and serum calcium. Three risk groups were formed: favorable, intermediate-risk, and poor-risk groups with a median survival of 26.9 months, 11.5 months, and 4.2 months, respectively. Table 1 compares clinical factors across models, and Table 2 demonstrates the risk-stratification and survival estimates of the available prognostic models in mRCC.
Prognostic Factors | MSKCC Motzer et al, 2002 | IMDC Heng et al, 2009 | GFI Negrier et al, 2002 | CCF Mekhail et al, 2005 | IKCWG Manola et al, 2011 |
---|---|---|---|---|---|
Performance status | • | • | • | • | |
Elevated serum LDH | • | • | • | ||
Low hemoglobin | • | • | • | • | • |
High corrected serum Ca | • | • | • | • | |
Time from Dx to Tx <1 y | • | • | • ○ | • | • |
Prior radiotherapy | • | ||||
Number of metastatic sites | • ○ | • | • | ||
Hepatic metastasis | ○ | ||||
Elevated ANC | • | ○ | • (WBC) | ||
Elevated platelets | • | ||||
Inflammation | • | ||||
Elevated ALP | • | ||||
Prior immunotherapy | • |
Risk Category | MSKCC | Median Survival, mo | IMDC | Median Survival, mo | GFI | Median Survival, mo | CCF | Median Survival, mo | IKCWG | Median Survival, mo |
---|---|---|---|---|---|---|---|---|---|---|
Good/favorable | 0 | 30 | 0 | 43 | 0–1 | 42 | 0 | 26 | RS a < −2.76 | 26.9 |
Intermediate | 1–2 | 14 | 1–2 | 22.5 | 2–3 | 15 | 1 | 14.4 | −2.76 < RS ≤ −1.25 | 11.6 |
Poor | ≥3 | 5 | ≥3 | 7.8 | 4–5 | 6 | ≥3 | 7.3 | RS ≥ −1.25 | 4.2 |
a Cutoff points for risk scores are placed at the 25th and 75th percentiles of the models’ risk score distribution as per Manola and colleagues.
The clinical course of patients with mRCC spans a wide spectrum, and distinct factors appear to forecast a more aggressive course of disease. RCC frequently metastasizes to the lung (60%–70%), regional lymph nodes (60%–65%), bone (39%–40%), liver (19%–40%), and brain (5%–7%). Vickers and colleagues examined 15% of patients with mRCC and brain metastases from the IMDC to demonstrate that OS was worse in patients with more than 4 brain metastases, compared with those with less than 4 metastases (3.9 vs 15.4 months, P = .005) McKay and colleagues similarly used the IMDC to analyze outcomes based on the presence of bone and liver metastases in patients treated with targeted therapy. Bone metastases were present in 34% of the 2027 patients in the consortium and were present in all risk groups but highest in poor-risk patients (43%, P <.001). Median OS was worse in patients with bone metastases compared with those without (14.9 vs 25.1 months, P = .0001). Similarly, liver metastases accounted for 19% of the group, were highest in poor-risk patients (23%, P = .003), and demonstrated worse median OS compared with those without liver metastases (14.3 vs 22.2 months, P <.001).
Risk Stratification in the Second-Line Setting
The development of risk-stratification models in the second-line setting was largely hampered by the poor survival seen in patients with mRCC in the cytokine era. As targeted therapy began to supplant traditional immunotherapy in the treatment of patients with mRCC, survival improved from less than 1 year in the cytokine era to approximately 24 months with targeted therapy. , Motzer and colleagues pooled 251 patients from 29 clinical trials between 1975 and 2002 to describe the survival of patients who were candidates for second-line therapy with novel agents after progression on cytokine therapy. Pretreatment features associated with shorter survival appeared to be low KPS, low hemoglobin level, and high corrected serum calcium. By use of these risk factors, patients were categorized into 3 groups: patients with zero risk factors (median OS, 22 months); patients with 1 risk factor (median OS, 11.9 months); and patients with 2 to 3 risk factors (median OS, 5.4 months). Subsequently, in 2015, Ko and colleagues sought to validate the IMDC model in patients with mRCC receiving next-line targeted therapy after progression on first-line targeted therapy. In a cohort of 1021 patients who received second-line targeted therapy, median OS since the start of second-line therapy was 12.5 months, and when compared with the MSKCC model, the IMDC model conferred a better concordance index (0.70 vs 0.66). Using the 3 risk categories per IMDC criteria, median OS was 35.3 months in favorable-risk patients, 16.6 months in intermediate-risk patients, and 5.4 months in poor-risk patients.
Non–Clear Cell Metastatic Renal Cell Carcinoma
The predominant histologic subtype of RCC is clear cell RCC, which accounts for 70% to 80% of all kidney cancers. Data collected from the IMDC demonstrated the median OS of patients with non–clear cell mRCC treated with novel targeted agents to be significantly poorer than patients with clear cell RCC (12.8 vs 22.3 months, P <.0001). The prognosis of patients with papillary RCC is significantly worse than their clear cell and chromophobe counterparts, and similarly, sarcomatoid RCC was found to carry an even poorer prognosis, with the percentage of sarcomatoid component potentially inferring worse OS. A report from the IMDC by Kyriakopoulos and colleagues showed patients with sarcomatoid histology have a shorter time to relapse, worse baseline prognostic criteria, and worse clinical outcome with targeted therapy with a progression-free survival (PFS) and median OS of 4.5 and 10.4 months, respectively. The aforementioned prognostic models in mRCC, however, were developed based on clinical and laboratory values, without consideration of histologic subtype. Given the worse clinical behavior of non–clear cell RCCs, Kroeger and colleagues aimed to characterize the applicability of the IMDC prognostic model to non–clear cell subtypes. The same IMDC criteria and risk categories still reliably predicted time to treatment failure and OS in this cohort of patients; the median OS of favorable-, intermediate-, and poor-prognosis groups were 31.4, 16.1, and 5.1 months, respectively.
Biomarkers in advanced renal cell carcinoma
As the era of precision oncology dawns, significant challenges lie in identifying biomarkers applicable in both clinical and research settings. Biomarkers are objective, quantifiable characteristics of biological processes. When used as outcomes in clinical trials, biomarkers are considered to be surrogate endpoints; however, sound scientific evidence that a biomarker consistently and accurately predicts a clinical outcome must exist. In such context, a surrogate endpoint is a biomarker that can be trusted to serve as a stand-in for, but not as a replacement of, a clinical endpoint. Stated differently, biomarkers may effectively be used as true replacements for clinically relevant endpoints if the normal physiology and pathophysiology of a biological process and the effects of interventions are completely understood; because this is rarely the case, the relevance and validity of biomarkers require iterative reassessment.
Risk Models as a Predictive Biomarker
Currently, biomarker development in mRCC is an emerging and evolving field of research because biomarkers are integral in delivering risk-directed therapies. Many of the clinical variables comprising the above risk-stratification models are potential biomarkers predictive of outcome because they are objective and quantifiable. To date, the only predictive biomarker prospectively validated in a phase 3 randomized controlled trial is the IMDC risk model, which was later validated in several other mRCC subsets, including non–clear cell RCC, in the second-, third-, and fourth-line settings, and in patients treated with ICB. Data from Checkmate 214, a phase 3 trial comparing nivolumab plus ipilimumab versus sunitinib for previously untreated advanced clear-cell RCC, demonstrated patients with intermediate- or poor-risk disease had favorable clinical outcomes with combination ICB versus sunitinib.
Genomic Biomarkers
Risk-stratification models leverage clinical and biochemical parameters to prognosticate patients; however, increasingly sophisticated molecular characterizations make it likely that genomic parameters will also be used in the prognostication of patients with mRCC. Large-scale sequencing efforts have helped characterize the genomic landscape of clear cell RCC. , VHL , a tumor suppressor gene located on chromosome 3p25, is the most commonly mutated gene in clear cell RCC and is involved in the regulation of hypoxia-inducible factor-α and angiogenesis. Three additional tumor suppressor genes, PBRM1 , SETD2 , and BAP1 , are located on the same 3p chromosomal region, and after VHL , comprise the most commonly mutated genes in clear cell RCC. Until recently, scant data existed regarding the correlation between genomic alterations and outcomes in patients with metastatic RCC; Carlo and colleagues explored the relationship between mutational profile and cancer-specific outcomes in a review of 105 patients with metastatic clear cell RCC who received prior VEGF-targeted therapy. The presence of BAP1 or TERT mutations was associated with significantly worse OS, whereas mutations in PBRM1 seemed to correlate with longer time to treatment failure and a trend toward superior OS.
In an effort to determine if the addition of the mutation status for several candidate prognostic genes to the MSKCC model could improve the model’s prognostic performance, Voss and colleagues leveraged 2 independent clinical trial datasets (COMPARZ trial [training cohort; n = 357] and RECORD-3 trial [validation cohort; n = 258]) of patients with mRCC treated with first-line targeted therapies. In the training cohort, the presence of any mutation in BAP1 or TP53 , or both, was associated with inferior OS (hazard ratio [HR] = 1.58; P = .0008) as well as absence of any mutation in PBRM1 (HR = 1.58; P = .0035). The mutation status for these 3 prognostic genes was then added to the original MSKCC risk model to create a genomically annotated version. Addition of genomic information improved model performance for predicting OS (c-index: original model, 0.595 vs new model, 0.637) and PFS (0.567 vs 0.602), and analyses in the validation cohort confirmed the superiority of the genomically annotated risk model over the original version. Similar efforts by Hsieh and colleagues aimed to evaluate the relationship between tumor mutations and treatment outcomes in RECORD-3. Among the cohort, prevalent somatic mutations were VHL (75%), PBRM1 (46%), SETD2 (30%), BAP1 (19%), KDM5C (15%), and PTEN (12%). With first-line everolimus, PBRM1 and BAP1 mutations were associated with longer (12.8 vs 5.5 months) and shorter (4.9 vs 10.5 months) median first-line PFS, and with first-line sunitinib, KDM5C mutations were associated with longer median first-line PFS (20.6 vs 8.3 months), providing compelling evidence that molecular subgroups of metastatic clear cell RCC based on the somatic mutations they harbor could have predictive values for patients treated with targeted therapy.
Recent work from several groups pointed to the association of immunotherapy response and mutations in the SWI/SNF chromatin remodeling complex, more specifically the polybromo and BRG-1 associated factors (PBAF) complex, which include the genes ARID2 , PBRM1 , and BRD7 . Miao and colleagues demonstrated that in a series of nearly 100 metastatic clear cell RCC patients, those harboring loss-of-function mutations in PBRM1 had clinical benefit from ICB. Similarly, a recent report validated the association between PBRM1 alterations and ICB response in CheckMate 025, a randomized phase 3 trial of nivolumab versus everolimus that demonstrated a survival benefit for nivolumab in the second- and third-line setting. Further functional and transcriptomic analysis suggested that PBRM1 -deficient tumors possessed altered immune signaling pathways. However, in IMmotion150, a phase 2 trial that randomized patients to first-line therapy with the anti–programmed death-ligand 1 (PD-L1) atezolizumab alone or combined to bevacizumab versus sunitinib, no association was seen between presence of PBRM1 mutations and treatment response to the PD-L1–directed atezolizumab, nor to the combination of atezolizumab plus bevacizumab (n = 136); there was a favorable effect on treatment response in patients receiving sunitinib (anti-VEGF) on the control arm of the same study (n = 72). Given the discordant clinical data on PBAF complex loss and response to immunotherapy, further investigation into its use as a biomarker is warranted.
Gene Expression Signatures
Several molecular gene signatures have been postulated and investigated as predictive of the efficacy of targeted therapy and immunotherapy. Beuselinck and colleagues performed global transcriptome analyses on 53 primary resected clear cell RCC tumors from patients who developed metastatic disease treated with first-line sunitinib and identified 4 discrete molecular subtypes of clear cell RCC that were associated with outcome and response to sunitinib (ccrcc 1–4). The ccrcc2 (53%) and ccrcc3 (70%) subtypes were enriched in patients responding to sunitinib compared with ccrcc1 (22%) and ccrcc4 (27%), and ccrcc1 and ccrcc4 tumors had significantly shorter PFS and OS compared with the ccrcc2 and ccrcc3 subtypes. Similarly, recent data demonstrate that these molecular subtypes correlate well with IMDC risk groups. Most good-risk patients (77%) had ccrcc2 tumors, which display a proangiogenic signature, whereas the ccrcc1 and ccrcc4 subtypes were almost exclusively found in intermediate- or poor-risk patients. These findings may explain the increased benefit of sunitinib over combined checkpoint blockade in good-risk patients in Checkmate 214.
A correlative study including 823 patients from IMmotion 151 demonstrated patients with tumors characterized by angiogenesis-high signatures experienced longer PFS with sunitinib when compared with those with angiogenesis-low tumors. In addition, tumors with T-effector/IFN-γ-high or angiogenesis-low gene signatures displayed favorable outcomes with combination therapy compared with sunitinib. MSKCC favorable-risk patients were noted to be enriched with angiogenesis-high signatures, akin to the biologic rationale of the aforementioned CheckMate 214 trial, which suggested a greater dependency on angiogenic pathways in favorable-risk disease.
Key genomic and transcriptional determinants of response to tyrosine kinase inhibitors were derived by Hakimi and colleagues , from molecular features of archival tumor specimens collected in patients receiving pazopanib versus sunitinib in the phase 3 COMPARZ trial. Clustering of the expression microarray data identified 4 biologically distinct clusters that were associated with significant differences in median OS and response to tyrosine kinase inhibitors. Detailed characterization of these clusters emphasized the central role of the tumor microenvironment (TME) and identified angiogenesis and macrophage infiltration as critical determinants of response to therapy. Superior outcomes for patients with higher angiogenesis scores were noted, independent of IMDC risk category (HR for PFS and OS 0.68 and 0.68, respectively). The investigators postulate that upregulation and suppression of angiogenesis observed with loss-of-function mutations in PBRM1 and BAP1 , respectively, provide a plausible explanation for the different clinical behaviors associated with these mutations.
Programmed Death-Ligand 1 Immunohistochemical Expression
The first and most widely studied potential biomarker for response to anti–programmed cell death 1/PD-L1 agents is the immunohistochemical expression of PD-L1. CheckMate 025 demonstrated PD-L1 staining to be associated with worse survival but not associated with response to nivolumab, suggesting PD-L1 staining has more prognostic than predictive value in mRCC. In both arms of the trial, an inferior OS was observed in patients with PD-L1 expression ≥1% compared with those with PD-L1 expression less than 1%. Nivolumab demonstrated a survival benefit over everolimus in both PD-L1 subgroups (median OS 21.8 vs 18.8 months in PD-L1 ≥1%, and 27.4 vs 21.2 months in PD-L1 <1%, respectively). Further supporting the notion of PD-L1 as a prognostic biomarker, Flaifel and colleagues combined tumor tissue from 2 clinical trials, METEOR (n = 306) and CABOSUN (n = 110), showing higher PD-L1 expression resulted in worse clinical outcomes in mRCC treated with targeted therapy. In addition, PD-L1 expression was not predictive of response to cabozantinib.
With regard to response to ICB, exploratory analyses of intermediate- and poor-risk patients in CheckMate 214 demonstrated OS was 86% versus 66% at 12 months and 81% versus 53% at 18 months, favoring combination ICB over sunitinib, with median survival not reached versus 19.6 months (HR = 0.45, 95% confidence interval = 0.29–0.71) among patients with PD-L1 expression ≥1%. Similarly, PD-L1 expression appeared to associate with improved overall response rate (ORR; 58% vs 22%) and median PFS (22.8 vs 5.9 months) in patients receiving combination ICB. Subsequently, in JAVELIN Renal 101, a phase 3 trial comparing avelumab plus axitinib with sunitinib in previously untreated patients with advanced RCC, the ORR among patients who received avelumab plus axitinib was double that among patients who received sunitinib, both among the patients with PD-L1–positive tumors and in the overall population (55.2% vs 25.5% and 51.4% vs 25.7%, respectively). Similarly, among patients with PD-L1–positive tumors, median PFS was significantly longer among patients who received avelumab plus axitinib than among those who received sunitinib (13.8 vs 7.2 months, P <.001). The IMmotion150 trial demonstrated a consistent trend of increasing efficacy with increasing levels of PD-L1, a finding further supported in the phase 3 study IMmotion151. , Finally, in KEYNOTE 426, the benefit of pembrolizumab plus axitinib over sunitinib with regards to OS and PFS was observed regardless of PD-L1 expression.
In sum, PD-L1 expression has proven to be an inconsistent biomarker in RCC. The optimal threshold for PD-L1 positivity is ill defined, and the heterogeneity that exists between primary (including tumoral vs tumoral and immune infiltrate expression) and metastatic sites lends clinical dilemma as to which sites should be sampled for PD-L1 expression. Variability in expression on the basis of specimen age, assay, and vendor choice is likewise an important consideration. Because PD-L1 is also a dynamic marker, expression may be altered on the basis of prior therapy.
Tumor Mutational Burden
Tumor mutational burden (TMB) refers to the total number of mutations per coding area of tumor genome. The rationale behind using TMB as a predictive biomarker of response to ICB is that a higher mutational load may be accompanied by increased production of neoantigens, thereby stimulating an antitumoral immune response. Prior studies in large cohorts of other cancer types, including non–small cell lung cancer and metastatic melanoma, demonstrate favorable responses to immunotherapy in patients with high TMB.
Evidence from Checkmate 025 and Checkmate 214 showing immunotherapy to be associated with favorable clinical benefit in poor-risk patients, a subgroup of patients harboring a high mutational load, supported the rationale for research of TMB as a biomarker in RCC. , , However, TMB does not appear to correlate with MSKCC or IMDC prognostic criteria, and no difference is seen in mutational burden between clear cell and more aggressive sarcomatoid components, suggesting that TMB is not associated with aggressivity of disease. , Furthermore, exploratory analysis of the previously mentioned IMmotion 150 trial showed no association between TMB or neoantigen burden and clinical benefit from ICB. Recently, Samstein and colleagues analyzed a large pan-cancer cohort of ICB (n = 1662) versus non-ICB (n = 5371) -treated patients whose tumors underwent targeted next-generation sequencing. For most histologies, an association between higher TMB and improved survival was observed; however, the same association was not seen in RCC.
Several parameters spanning a wide spectrum of radiologic, pathologic, molecular, genomic, urine-, and serum-based factors have been investigated as predictive and prognostic biomarkers, although their study remains preliminary and not validated. A brief summary of biomarkers in RCC is outlined in Fig. 1 . Angiogenesis-related parameters detected in blood are attractive as blood biomarkers because they are not subjected to tumor heterogeneity and allow longitudinal follow-up. Immune-related adverse events have been explored as surrogate markers of pharmacodynamic effect, suggesting that mechanism-based toxicities may potentially serve as biomarkers. Interestingly, a recent study by Sanchez and colleagues found aspects of the TME that vary by body mass index in the tumor and peritumoral adipose tissue, possibly contributing to the observed survival advantage in obese patients with clear cell RCC.
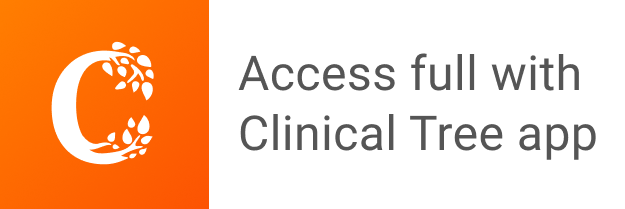