Computer-Assisted Detection of Polyps
Computer-assisted detection (CAD) software is a fairly recent development in CT colonography. CAD software automatically detects and marks certain image content based on predefined morphological criteria. In mammography CAD has already been approved and is in clinical use for cancer detection. In CT colonography, CAD is used to detect intraluminal polypoid structures in the colon and rectum. The findings are highlighted and presented to the reader, who decides whether the findings marked by the CAD system as “suspicious” are true lesions (true-positives) or not (false-positives). Various manufacturers have developed commercially available CAD software, and CAD is expected to become more routinely used for diagnostic procedures in the near future. It has been shown in recent trials that the clinical use of CAD in CT colonography, when used in second-reader mode, helps improve radiologists’ accuracy. Some CAD algorithms have recently received clearance by the US Food and Drug Administration (FDA).
Principles
Procedure
Most colon CAD algorithms are based on the following (highly simplified) steps:
Segmentation (extraction) of the target organ (colon) which contains the pathology (polyps) from the axial CT colonography dataset
Detection of “candidate” polyps
Classification of the candidates as potential polyps or pseudolesions, with subsequent marking of the suspect lesions (Fig. 3.45)
Presentation of the CAD markers to the radiologist
Segmentation. The first step in a CAD scheme is to extract, or segment, the colon from the axial CT colonography dataset. This is done to reduce the amount of data to be processed, thus speeding up the computation and restricting the CAD marks to the target organ, eliminating marks on structures outside the colon that might mimic real lesions based solely on their local structure. Simple but fast segmentation methods detect air-filled regions. More complex but slower algorithms also analyze the segmented regions, linking isolated bowel segments of the colon if necessary, thus ensuring that all non-collapsed bowel segments are always included and nothing else is.


Detection of candidate polyps. After extraction of the colonic lumen from the dataset, the next step is detection of candidate polyps. In this step the goal is to find any polypoid-looking structure. Usually, geometrical properties are used for the search. Most polyps have a circumscribed, round, elevated cap-like morphology, while semilunar folds have a longitudinal ridge-like appearance. The remainder of the bowel wall is virtually flat except near the haustra, where there are slight depressions. The goal of this step is to detect a small number of candidate polyps, thus further reducing the complexity while maintaining a high sensitivity. For this reason, Yoshida and Dachman (2005), for instance, applied a volumetric shape index (Fig. 3.46).
Classification of candidate polyps. In a final step, the false-positive candidate polyps are eliminated. The candidate lesions are sequentially characterized according to various features such as structure, volume, and shape. By means of statistical classification based on those features, each candidate lesion is then classified as true-positive or false-positive. A CAD classifier is “trained” on a large set of true polyps in such a way that its final operating point achieves a good balance between sensitivity and the number of false-positive marks.
Presentation to the radiologist. The CAD results are then presented to the interpreter for a final analysis. The lesions marked in the image data with numbers, crosshairs, colored dots, etc., are presented to the interpreter on 2D and 3D images (Figs. 3.47 and 3.48). Most manufacturers also offer a numbered list of CAD detections.
Most CAD algorithms are designed for a specific size range of target lesion, e.g., 5–25 mm, and have a reduced sensitivity for recognizing lesions that do not fall within this range. Larger lesions are, however, easily identified by an observer even without CAD, and smaller ones are clinically less relevant.
Possible Settings
CAD systems may be roughly divided into those in which the settings can be modified and those (closed) systems where the settings are predefined and cannot be modified.
Systems with predefined settings. In closed CAD systems the examiner cannot modify the operating point of the computer algorithm in terms of its sensitivity and specificity. An example is the polyp-enhanced view (PEV) algorithm from Siemens. The advantage of a closed system is that it is easy to use and reduces the likelihood of user error. CAD results for the same patient are reproducible and the system will always give exactly the same results. In addition, it allows predictable characterization of the expected reader accuracy impact when the combined human–machine system (CAD system and radiologist) is analyzed as an overall diagnostic system. When the CAD system settings are adjustable by users, this type of reader impact analysis is more difficult to characterize and the overall diagnostic effect of the CAD system in the clinical routine is less predictable.
Modifiable systems. Modifiable CAD software allows the radiologist to adjust certain morphological search parameters and thus to influence the performance of the CAD system. Examples include a system produced by Medicsight (London, UK), which is included in many workstations, e.g., Vitrea (Vital Images, Inc., Minnetonka, Minnesota, USA) and Viatronix (Viatronix, Inc., Stony Brook, New York, USA).




An important setting is sphericity. Sphericity filters allow the search characteristics of the CAD algorithm to be altered with regard to the morphology of the target lesion (polyp). Sphericity describes the “roundness” of the target lesion (Fig. 3.49). In the CAD algorithm by Medicsight, for instance, the sphericity filter can be adjusted between 0.0 and 1.0 in increments of 0.1. At a filter setting of 1.0, only structures with a perfectly round (spherical) shape are marked. No other structures are identified. At filter settings close to 0.0, structures with a virtually flat surface are also detected. High-sphericity settings, that is, selecting for a round morphology, result in a lower sensitivity (since some less than perfectly spherical polyps will not be recognized) and a higher specificity. If a low-sphericity filter is used, the opposite is true: sensitivity is increased and even flat polyps can be detected. However, the specificity will fall as a result, with other elevated structures or polypoid pseudolesions being detected (Fig. 3.50).
Adjustability of the sphericity filter, although intuitive, may oversimplify the great diversity of polyp shape (including many highly nonspherical polypoid structures) in real patient populations, and it is difficult for users to estimate its impact on ultimate accuracy, especially any decrease in overall reader+CAD specificity, which can affect work-up rates by leading to unnecessary optical colonoscopy.
Conclusion. The ability to modify a CAD system enables the radiologist to adjust the system to his/her expertise level or personal preferences; for example, an inexperienced reader might want to be offered more CAD marks to reduce the risk of overlooking a polyp, accepting that this will also mean being offered more false-positive findings. However, it also means it is possible to use inappropriate settings, with the risk of lowering the sensitivity for relevant lesions and decreasing overall system specificity, leading to more unnecessary diagnostic work-ups. More rigid, fixed systems offer a better way to characterize the CAD algorithms, and therefore have a more predictable impact on overall clinical sensitivity and specificity and thus the cost:benefit ratio of using CAD.
Comparability of CAD Software Systems
Various CAD systems are now available from various manufacturers for CT colonography. However, different manufacturers generally use different computer algorithms in the development of their systems. Thus, even if two systems are comparable in terms of performance characteristics (sensitivity and specificity)—which usually they are not—the CAD algorithms often calculate completely different false-positive and true-positive findings. This can also occur with different versions of a program from the same manufacturer. While sensitivities tend to range from 80% to 90%, the number of false-positive findings may vary considerably. Different systems are often optimized for a particular method of bowel preparation or a particular target group of patients. This is also because CAD algorithms are often trained on specific patient populations (e.g., those with high prevalence of disease). If the software is later used with a different group of patients (e.g., patients with a low prevalence of disease), the results may differ.
Use in Practice
There are several factors that influence the performance of CAD algorithms when used in routine daily work. Most algorithms yield the best results in a patient with a thoroughly cleansed bowel and optimal colonic distension. Factors such as varying visibility of a lesion in the supine and the prone scan due to poor distension or the presence of residual fluid can impair the detectability of a lesion, not just for a radiologist, but also for CAD. Lesions that are located in a collapsed bowel segment or obscured by stool or fluid naturally cannot be identified by CAD. As in conventional evaluation by a human observer, CAD must be used with a prone/supine evaluation to increase sensitivity.
Preparation and examination protocols. Ideally, a CAD system for CT colonography should work on any dataset, regardless of the method of bowel preparation and the examination protocol. However, some CAD algorithms are designed with particular preparation protocols in mind. While many CAD systems only offer good results when used with a thoroughly cleansed bowel, some vendors offer CAD systems that will function with fecal tagging. The majority of CAD systems are designed for a specific range of slice thicknesses. One system that has a high sensitivity when certain bowel preparation techniques or examination methods are used may perform considerably differently when other preparation or examination techniques are used, whereas another program may be more robust and resilient to changing examination conditions, and may perform equally well in both groups. For the use of CAD in clinical practice, therefore, it is important that the algorithm is compatible with the patient preparation and examination protocol being used if the best possible results are to be achieved. Any deviations from the usual protocol may potentially affect the performance of the CAD system.
Poor bowel preparation. Inadequate bowel preparation leaving a large amount of residual fecal matter makes evaluation difficult, both for the radiologist and the CAD. CAD algorithms detect not just colonic polyps, but other polypoid colonic structures, including polypoid stool, especially if the inner density of these residual particles is relatively homogeneous. Consequently, applying CAD to the datasets of poorly prepared patients results in the marking of numerous (often more than 100) false-positive pseudolesions, making useful analysis impossible (Fig. 3.51). In such situations, CAD should not be employed.
Flat lesions. Although the spectrum of false-negative findings varies between CAD systems, most have a low sensitivity for detecting less conspicuous flat or nonpolypoid lesions. This is because most algorithms are optimized to detect round polypoid lesions rather than flat or minimally raised lesions. Adapting the operating point of the algorithm to detect lesions with a less round appearance—for instance, by adjusting the sphericity filter closer to 0.0—is usually associated with an increased rate of false-positive identifications.
Carcinomas. The situation for carcinoma detection is quite similar. The algorithms are intended for detection of polypoid structures and not primarily for cancer detection. A carcinoma may nevertheless be detected if its surface has a polypoid curvature that fits with the CAD algorithm (Fig. 3.52). Detection rates vary depending on the CAD software used, but with some manufacturers they are lower than for polyps. Algorithms that also have sensitivity for carcinomas are currently under development.
Extracolonic structures and organs are not included in the CAD assessment, but have to be evaluated by the radiologist.
False-positive findings. Along with sensitivity, another major parameter in the practical use of CAD is the rate of false-positive detections per patient. In a screening population with a low prevalence of adenomas, the percentage of patients with significant lesions additionally detected by CAD (true-positive detections) may be small. False-positive CAD findings, on the other hand, will occur in nearly every patient and will therefore add considerably to the cumulative evaluation time. Common causes of false-positive markings are residual stool, tagging artifacts, bulbous semilunar folds, the ileocecal valve, the rectal tube, and extracolonic lesions. False-positive CAD markers are also often found in extracolonic hollow organs such as the small intestine and stomach (Fig. 3.53). Presumably, the vast majority of false-positive results on CAD are readily identified by the radiologist. Nevertheless, large numbers of false-positive results on CAD are ultimately detrimental:



As the false-positive rate increases, so does the time taken to interpret the scans.
There is an increased risk that the radiologist will misinterpret false-positives presented by CAD, possibly reducing specificity.
Using CAD software can be fatiguing and the reader′s attention may drop. In the worst case, reader misinterpretation of true-positive findings presented by the CAD software among many false-positive markings will lead to decreased sensitivity.
Minimizing false-positive results is therefore of paramount importance.
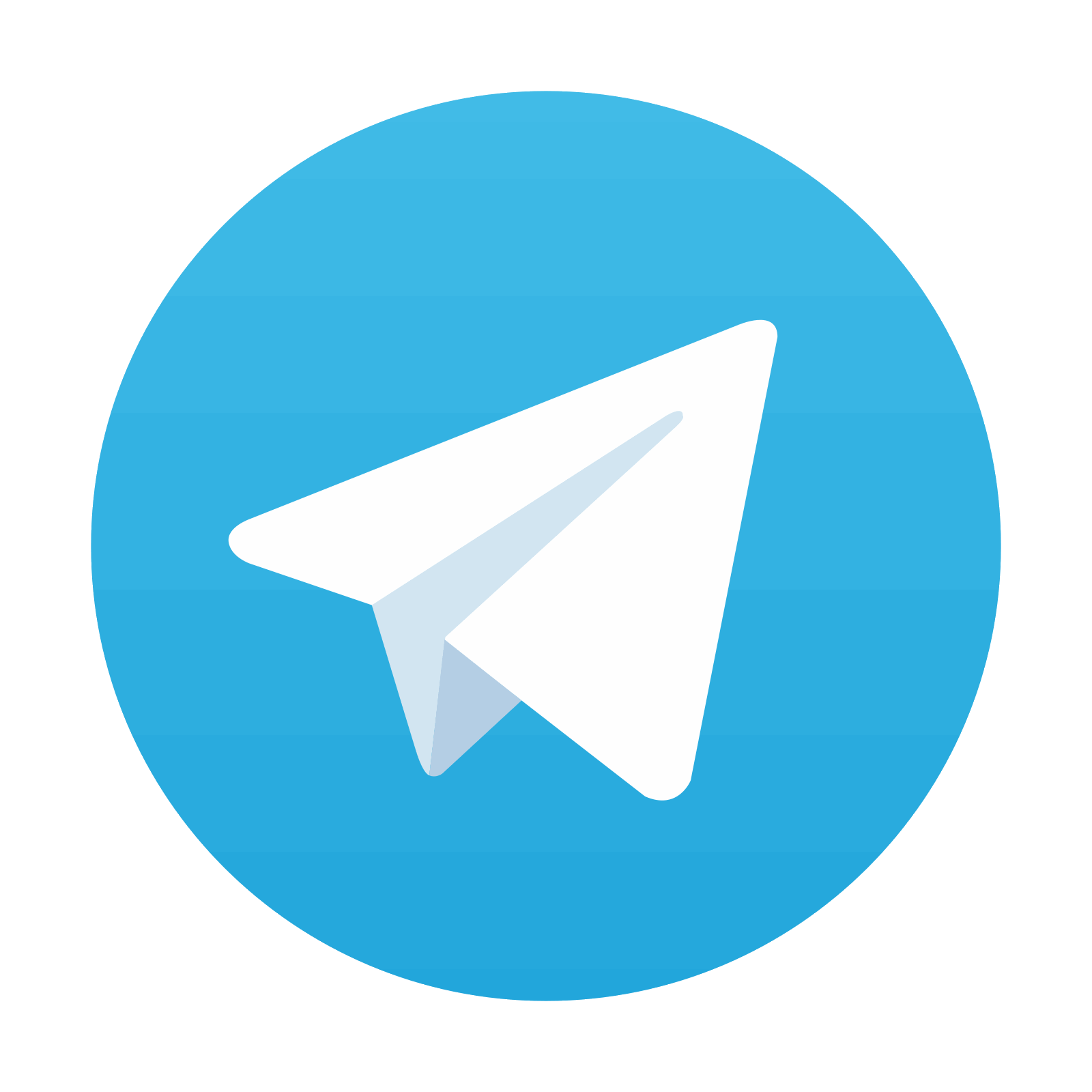
Stay updated, free articles. Join our Telegram channel

Full access? Get Clinical Tree
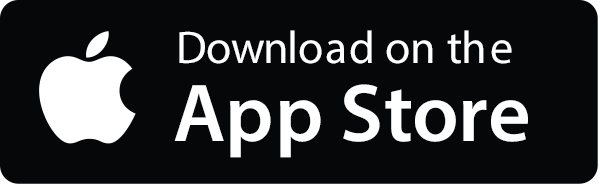
