Acknowledgments
Original work by the authors was funded by the NIH (grant 1U01AI070107) and National Health and Medical Research Council of Australia. Karen Keung is a recipient of the Jacquot Research Entry Scholarship from the Royal Australasian College of Physicians and the Australian and New Zealand Society of Nephrology. Philip O’Connell is a recipient of an NHMRC Practitioner Fellowship.
As is the case for other complex therapies and diseases, transplantation is at the cusp of transitioning from empirical therapy where “one size fits all” to an era of personalized medicine, where therapy is tailored to meet the specific needs of an individual patient. Although the transplant community has realized the inadequacy of our current treatments, we currently lack the tools to stratify patients according to predictive risk. We have limited options when it comes to induction and maintenance immunosuppression and no approved treatments for antibody-mediated rejection (AMR; acute and chronic), delayed graft function (DGF), or desensitization. Furthermore, apart from a transplant biopsy, we lack the diagnostic tools to differentiate acute kidney injury (AKI) from acute rejection and we lack the prognostic biomarkers that can reliably predict long-term outcomes at a time when a change in therapy may be advantageous.
To be able to evaluate new therapies for these conditions there has been an increasing need for biomarkers and surrogate endpoints (SEPs). In other specialties biomarkers have been shown to reliably assess prognosis and predict how individual patients will respond to treatment. Related to this, SEPs have the potential to more rapidly evaluate the clinical effectiveness of therapeutic interventions in clinical trials and reliably predict clinical endpoints such as patient and graft survival. Although there have been countless papers describing “biomarkers” for specific conditions in transplantation, very few, if any, have undergone the rigorous validation required to fulfill the definition of a biomarker. The first steps to identifying a biomarker for conditions such as AKI or rejection is to identify clinical indicators of each condition, to understand their effect on long-term outcomes, and most importantly, to better understand the pathogenesis of the underlying condition.
Definition of Biomarker and Surrogate Endpoint
Biomarker is one of the most misused terms in transplantation. A PubMed search of “biomarker and kidney transplantation” revealed 5857 articles. Yet most of these potential biomarkers have not undergone the rigorous validation required for clinical use. The definition of biomarker and SEPs are outlined in Table 26.1 . According to the Biomarkers Definitions Working Group a biomarker is: “a characteristic that is objectively measured and evaluated as an indicator of normal biological processes, pathogenic processes, or pharmacologic responses to a therapeutic intervention.” Predictive biomarkers (i.e., those that forecast the likely response to a specific treatment) require extensive external validation, ideally in external randomized clinical trials and meta-analysis. Biomarkers can take on many forms, from cellular assays, to imaging or serum markers. However, as transplant immunosuppression becomes more target specific, such as with newer agents like belatacept and Janus kinase (JAK) inhibitors, molecular and genetic biomarkers will become increasingly important. If transplant immunosuppression is to become more personalized then biomarker discovery is an essential prerequisite. Without validated biomarkers it will not be possible to rationally stratify patients to different treatments based on risk and expected response to therapy. Therefore the first step is a sound understanding of the underlying pathophysiology of what we are trying to prevent (e.g., acute cellular rejection [ACR]). The more biologically plausible the biomarker is, the more likely it is to be adopted for clinical use.
Term | Definition |
---|---|
Biomarker | A characteristic that is objectively measured and evaluated as an indicator of normal biologic processes, pathogenic processes, or pharmacologic responses to a therapeutic intervention |
Prognostic biomarker | Biomarker that forecasts the likely course of disease irrespective of treatment |
Predictive biomarker | Biomarker that forecasts the likely response to a specific treatment |
Clinical endpoint | Measurement providing information on how a patient feels, functions, or survives |
Surrogate endpoint | Measurement providing early and accurate prediction of both a clinical endpoint and the effects of treatment on this endpoint |
Validation | Confirmation by robust statistical methods that a candidate prognostic or predictive biomarker or surrogate endpoint fulfils a set of conditions that are necessary and sufficient for its use in the clinic |
Prognostic biomarkers do not necessarily have to predict the effect of treatment on a particular outcome but they still require robust statistical validation to be clinically useful ( Fig. 26.1 ). First, there must be a demonstration that a correlation exists between the biomarker and the outcome of interest (e.g., graft loss). Second, there must be an independent statistical validation of the relationship. Depending on the robustness of the clinical data used to show an association between the biomarker and the endpoint further studies will be required. If the association was demonstrated on retrospectively collected samples or tissues, then it needs to be validated prospectively and then by multicenter validation. Ultimately its clinical usefulness will require evaluation in randomized clinical trials where it is not clear what the most appropriate treatment is for an individual patient based on the best clinical indicators available. For instance, patients at low risk of rejection or graft loss may benefit from minimizing immunosuppression over time, whereas patients at high risk of graft loss would require a completely different strategy. Recent trials have shown that we are not good at predicting this early after transplant, and a prognostic biomarker would be useful if it could reliably stratify patients to different treatment options.

Just as biomarkers are an essential component for stratifying patients for clinical trials, SEPs will be an essential component of trial design. SEPs are measurements that provide early and accurate prediction of both the clinical endpoint (e.g., graft loss) and the effects of treatment on this endpoint. For surrogates to be fully accepted they need to be validated in clinical trials. Whereas observational studies and meta-analysis can demonstrate that a SEP is prognostic for disease outcome, it requires randomized clinical trials to show that the effect of the intervention on the SEP correlates sufficiently with the effect on the true endpoint. This concept is more advanced in oncology than it is in transplantation. Yet even in oncology there are few SEP for solid tumors, whereas the most accepted SEP is in nonsolid hematologic tumors where clinical remission has long been regarded as a marker of time to disease progression and overall survival.
There are several candidates for SEP in transplantation. The most important SEP is one that predicts patient death and/or graft loss. In transplantation, death and graft loss are relatively late events and therefore are impractical endpoints for clinical trials in transplantation. Therefore trials focused on events in the first 1 to 3 years tend to give little insight into important outcomes over the long term. People have proposed several SEPs that are predictive of death and graft failure including: 1-year serum creatinine, doubling of serum creatinine, acute rejection, spot albumin:creatinine ratios, and delta estimated glomerular filtration rate (eGFR). Although none of these is perfect, delta eGFR seems the most promising at this point. A good SEP needs to be both prevalent and predictive. When evaluated in patients enrolled in the ANZDATA registry a decline in eGFR of ≥30% from year 1 to 3 posttransplant not only was found to be reasonably common, occurring in 10% of patients but also was associated with a 2.2-fold risk of death and a 5-fold increase in death-censored graft failure. Other SEPs such as 1-year serum creatinine were less predictive than a delta measurement, whereas a doubling of serum creatinine was more predictive than a ≥30% change in GFR but also less prevalent, occurring in only 1% of recipients. Spot urine albumin:creatinine ratio might be predictive of hard endpoints but is difficult to standardize and lacks specificity. Other more recent predictors of graft loss include inflammation in areas of interstitial fibrosis, inflammation within areas of interstitial fibrosis and tubular atrophy (iIFTA), and de novo donor-specific antibody (DSA). More information is required to determine how predictive iIFTA is of graft loss using modern immunosuppression, but it does require a biopsy to derive the SEP. De novo DSA will be discussed in detail in the later part of this chapter.
The problem with all these measurements is that most have been validated from registry studies, which are retrospective populations recruited over a long timeframe and covering many different eras of immunosuppression. For instance, patients on cyclosporine and azathioprine may have more episodes of rejections than those on other immunosuppressive regimens, often with greater severity, and this may skew the assessments of prediction. What is required now is to validate these tests in prospectively recruited patient cohorts on current standard of care immunosuppression to confirm these original observations. If these observations are confirmed, the next step is to use the SEP in a randomized clinical trial to test its clinical utility. For a SEP to be useful as an endpoint in a clinical trial the effect of any trial therapy on the SEP must be equivalent to the effect on the hard endpoint (e.g., death-censored graft loss).
In essence, the greater the association of the SEP with the clinical endpoint and the greater the statistical validation, the more likely it is to be accepted for interventional clinical trials. Ideally SEP should adhere to the Prentice criteria ( Table 26.2 ). The surrogate should predict the clinical endpoint, treatment or intervention should have a significant effect on both the surrogate and the clinical endpoint, and the treatment effect on the surrogate should mirror the treatment effect on the clinical endpoint. This latter point is ideal but has been proven to be unrealistic. An alternative definition is that the surrogate must be prognostic of transplant outcome and the effect of treatment on the SEP must correlate sufficiently with the effect on the true outcome. Either way the correlation must be validated in clinical trials or meta-analysis. In addition, the SEP should be biologically plausible.
|
|
|
|
New Technologies for Biomarker Assays
The advent of the new “omic” technologies has transformed the field of biomarker development. They provide new tools to accurately identify the underlying pathology associated with graft injury and acute rejection. As with all new technologies they need robust validation in the clinical setting, and initial exploratory findings must be developed into easy-to-use, reproducible, and accessible clinical assays. Older genomic techniques confined the study of disease phenomena to one or a few candidate genes at a given time. These genes were selected based on biologic plausibility or animal experimentation, and the studies were hypothesis-driven. High-throughput technologies, such as microarray and RNA-sequencing, are able to identify the differential expression patterns of thousands of genes associated with a particular outcome or disease state. By combining these with computational analysis it is possible to reveal the cumulative signaling pathways operational in a pathologic state. With this information, it is possible to develop gene-based biomarkers that can be used clinically to stratify patients according to risk.
Important characteristics of commonly employed strategies to examine genomic information in research settings in transplantation are summarized in Table 26.3 . Microarrays work on the principle of DNA complementarity (see review). The NanoString nCounter system is a new technology for molecular profiling that uses a novel color-coded barcode technology, with each barcode representing a single target molecule. It enables digital counting of individual molecules without the need for amplification. Up to 800 transcripts can be profiled in a single reaction, and it has been applied to samples of blood, raw cells, and urine. Its role in transcriptome profiling in other biomedical fields, such as oncology, has been prominent in recent years, but only recently has it been used in the setting of renal transplantation.
Advantages | Disadvantages | |
---|---|---|
Quantitative RT-PCR |
|
|
Microarray |
|
|
Next Generation Sequencing (NGS) |
|
|
NanoString nCounter analysis system |
|
|
Proteomics is the large-scale study of proteins. Previously, profiling of the proteome was limited by its complexity, but has gained significant momentum with technologic advancements, particularly in relation to mass spectrometry (MS). Urine has been frequently used in both nontargeted and targeted proteomic studies of the renal allograft, as has peripheral blood. Earlier studies using MS-based technology in urine proteomic profiling identified protein signals that were present in subjects with acute rejection, but did not further characterize the protein associated with these signals. In a proof of principle study, surface-enhanced laser desorption/ionization—time of flight—mass spectrometry (SELDI-TOF-MS) was used to discover urine protein peaks associated with ACR. A follow-up study revealed that these were protein derivatives of nontryptic cleaved forms of β-2 microglobulin. However, a multicenter study did not identify a statistically significant correlation of intact or cleaved β-2 microglobulin in urine in the presence of acute rejection. This prospective targeted observational study conducted through the Clinical Trials in Organ Transplantation-01 (CTOT-1) protocol is described in more detail later. Other studies have applied a variety of proteomic techniques to identify protein expression in an unbiased high-throughput manner in allograft rejection, before using targeted enzyme-linked immunosorbent assay (ELISA) for validation of biomarker panels. However, as with the majority of promising proteomic studies to date, multicenter prospective trials are required to validate these candidate markers before meaningful conclusions can be made.
Transcriptomics is the study of the RNA transcripts encoded by the genome. Although much of the work in the past decade has been in mRNA evaluation, studies in microRNA (miRNA) expression profiling in the setting of renal transplantation has increased exponentially in recent years. Small, noncoding RNAs, miRNAs regulate gene expression of target mRNAs. Similar to genome and mRNA profiling, large-scale sequencing of miRNAs has been dominated by microarray applications. One of the advantages of this technology is that there are far fewer miRNA than genes and hence the computational analysis is simpler, and there remains the possibility that a more robust marker could be identified as a predictor of either acute rejection or IFTA. Scian and Mas have elegantly summarized miRNA profiling studies of the renal allograft in their reviews. To date no conclusive patterns of miRNA expression have been associated with acute rejection or IFTA.
Metabolomics relates to the global profiling of small molecule (<1500 Da) metabolites. Because the genome, transcriptome, and proteome can undergo further changes after translation, the metabolome reflects the downstream products of these processes. There have been advances in separation techniques, such as capillary electrophoresis and ultrahigh-pressure liquid chromatography (UPLC) systems, and improvements in analytic approaches, being MS-based methods and high-resolution nuclear magnetic resonance (NMR) spectroscopy. This has enabled the simultaneous study of dozens of small molecule metabolites in tissue and biologic fluids. Whereas the use of nontargeted metabolomic approaches in the study of renal diseases and drug metabolism have been well documented, studies demonstrating its potential role in monitoring kidney transplants are limited. It has been used to identify borderline tubulitis and acute T cell mediated rejection in pediatric renal transplant recipients. Smaller studies have also been published evaluating the differential urinary and serum metabolomic profile in adult allograft recipients with acute rejection.
Biomarkers of Acute Kidney Injury
Being able to differentiate AKI from acute rejection would be a useful tool in the management of transplant recipients especially in the first few weeks after transplantation. In addition, a test undertaken in the donor or within the donor allograft that predicted DGF or ultimate graft performance would help in donor graft allocation and the management of patients postoperatively. In nephrology, neutrophil gelatinase associated lipocalin (NGAL), kidney injury molecule-1 (Kim-1), and N-acetyl-b-D-glucosaminidase (NAG) have been identified as biomarkers of AKI. NGAL is released from tubules, tends to rise before serum creatinine, and is not affected by volume depletion. Kim-1 was overexpressed in kidney biopsy specimens in patients with acute tubular injury, and urine excretion of Kim-1 has been shown to distinguish acute tubular injury from other forms of AKI. In kidney transplant recipients, high urinary excretion of Kim-1 was found to be an independent predictor of graft loss within a 4-year follow-up with hazard ratios between 3.6 and 5.1. However, its sensitivity and specificity as a test were not evaluated.
The advantage of these biomarkers is that they are measured in the urine and do not require renal tissue for analysis. However, evidence of the utility of these biomarkers in transplantation has been sparse. When measured in deceased donor urine, NGAL, in combination with liver fatty acid-binding protein (LFABP), were predictors of kidney injury and were associated with worse eGFR at 6 months, whereas expression of YKL-40, a marker of kidney repair, was associated with improved function after DGF. Other studies have shown an association between elevated urinary levels of NGAL and interleukin (IL)-18 in deceased donors and the onset of DGF in the recipient. However, in both cases the association was modest and, as yet, have not been introduced into clinical practice. In a longitudinal study of 182 transplant patients, elevated urinary NGAL levels were somewhat predictive of AKI from all causes other than rejection (specificity 0.86 and sensitivity 0.86), whereas if very high levels of NGAL were present it was highly predictive of acute rejection (sensitivity 1.00 and specificity 0.93). Some of these molecules may ultimately be useful in clinical settings, but further prospective evaluation in clinical trials is required. Other urinary molecules of AKI have been evaluated in the transplant setting. Cystatin C showed an association with DGF but with a receiver operating characteristic (ROC) area under the curve of 0.54 to 0.74, the accuracy in the transplant setting was low.
The problem with many of these assays in the transplant setting is that AKI is often seen in association with acute rejection. Hence, developing an assay that can reliably separate nonimmunologic causes of AKI and rejection-associated AKI has been difficult. More recently, genomics has been used to develop a molecular signature of AKI. Paradoxically it has been difficult to develop such a signature in studies of renal disease, because AKI is self-limiting and renal biopsies are not often performed. Kidney transplants experience AKI and are often biopsied in these circumstances, and are thus ideally positioned to study the gene expression patterns of AKI. cDNA microarrays were used to study the gene expression patterns of 14 living donor kidneys, 15 deceased donor kidneys with immediate function, and 14 deceased donor kidneys with AKI (defined by dialysis in first week). The gene expression patterns of living donor kidneys were observed to cluster separately from deceased donor kidneys (132 genes). In kidney transplants with AKI immediately after transplant there were 48 transcripts related to cell cycle regulation, cell growth/metabolism, and signal transduction that were significantly differentially regulated compared with those with immediate function. In a separate study, the same authors compared gene expression patterns of AKI allografts with carefully selected, pristine 6-week protocol biopsies, and identified 394 transcripts that were differentially expressed in AKI. Using the geometric mean of the fold increase in the top 30 differentially regulated genes (compared with control nephrectomies), they devised an IRRAT (injury-repair-response-associated-transcript) score that correlated inversely with renal function at the time, and directly with eGFR improvement thereafter. The IRRAT score was significantly associated with DGF, deceased donor status and interstitial inflammation, but not histology. IRRAT scores have since been observed to be elevated in both ACR and AMR, suggesting the nonspecific nature of this injury response signature. Of note, AKI-related gene expression patterns identified by different groups in transplantation have shown significant overlap with both mouse orthologs identified in ischemia-reperfusion models, and with each other, suggesting molecular homogeneity in this injury response. Currently, genetic markers of AKI have relied on analysis of biopsy material. From a clinical utility perspective, this makes it no more beneficial than histologic evaluation, which is the current gold standard. For biomarker development IRRAT scores or other genetic markers of AKI would need prospective validation in a clinical trial and ideally would use serum or urine for analysis, thereby avoiding the requirement for a biopsy.
Biomarkers of Acute Rejection in Renal Transplantation
Biopsy of the renal allograft remains the gold standard for the diagnosis of acute rejection and/or other pathologies in the transplanted organ—the histologic profile aids therapeutic decisions and may provide prognostic value. Nevertheless, biopsies are invasive with inherent risks, and variability in sampling and interobserver biopsy scoring raises questions regarding their diagnostic accuracy. Serum creatinine and proteinuria remain established noninvasive markers used for allograft surveillance, but a change in these parameters is neither sensitive nor specific to a specific etiology and still necessitates a biopsy. Furthermore, subclinical rejection, by definition, is not accompanied by an elevation in the serum creatinine. The identification of noninvasive biomarkers of acute rejection with high diagnostic accuracy based on their sensitivity, specificity, positive predictive value (PPV), and/or negative predictive value (NPV), which could assist in screening patients for biopsy or to replace the need for a biopsy altogether, is the holy grail in biomarker discovery in transplantation.
The establishment and widespread application of high-throughput omics technologies over the past two decades has generated large-scale data and provided greater understanding of the molecular pathways and mechanisms in various states of allograft injury, with much of the focus on acute rejection. Furthermore, it has provided opportunities to discover biomarkers in acute rejection of diagnostic, predictive, or prognostic relevance in blood (sera, peripheral blood mononuclear cells) and/or urine. A number of earlier studies in the biomarker search in acute rejection employed more traditional laboratory techniques such as quantitative PCR (qPCR) and ELISA to measure mRNA and protein levels, respectively, of a select number of molecules in a targeted, or “biased” manner. However, genomic/transcriptomic technologies such as microarray, RNA sequencing, and NanoString, and myriad proteomic strategies, coupled with exponential advancement of computational analytical methods have enabled the simultaneous measurement of tens to hundreds of thousands of molecules in a disease state and provided opportunities to identify potential biomarkers using a nontargeted approach. These have enabled us to go beyond the study of genes, RNA, and proteins as potential biomarkers, but to evaluate even smaller molecules, including miRNA and peptides.
To identify any biomarker, understanding the underlying pathogenesis of the condition is essential. The basic mechanisms of acute rejection in renal allografts are well understood and extensively reviewed. Important features to emerge include the need not only to appreciate the shared immune mechanisms between AMR and ACR, but also to recognize the biologic pathways that are unique to each rejection type and thus confer differences in prognosis and therapeutic decisions. This is further complicated by coexistence of both (mixed rejection) and/or coexistence with other pathologies.
Overall, there is a lack of consistency in the identification of candidate biomarkers across individual published studies. The reasons for this are multiple small sample sizes; heterogeneity of patient demographics; variability of the transcriptional milieu of acute rejection in the allograft, urine, and/or peripheral blood, which is further influenced by immunosuppressive agents; acute rejection type or subtype and severity (further complicated by the presence of mixed rejection); the timing of the rejection episode; the type/quality of the allograft; concurrent pathology (e.g., BK virus, IFTA, ATN); and the representativeness of the biopsy sample used for transcriptome analysis. Furthermore, the lack of validation in large prospective, independent cohorts is where a number of noninvasive candidate biomarkers falter and are delayed from proceeding toward potential clinic application. However substantial research has been undertaken and a number of promising approaches have been identified (see detailed review ). This chapter will discuss the more promising noninvasive biomarkers of acute rejection in renal transplantation. The larger multicenter studies and their proposed biomarker candidates have been tabulated in Table 26.4 . It should be highlighted that some of these studies adopted a targeted approach where molecules of interest were preselected for assessment of their biomarker potential, whereas others adopted unbiased techniques to discover candidate molecules before proceeding with targeted validation of a more refined set of molecules.
Study | Biofluid | Biomarker |
---|---|---|
Suthanthiran et al., CTOT-4 | Urine (mRNA) | Combined 3-gene signature: CD3ε, IP-10(CXCL10), and 18s ribosomal RNA |
Hricik et al., CTOT-1 | Urine (protein) | CXCL9, CXCL10 |
Li et al. a | Whole blood (mRNA) | DUSP1, NKTR, MAPK9, PSEN1, PBEF1 |
Roedder et al., AART | Whole blood (mRNA) | kSORT (17-gene set) |
Kurian et al. | PBMCs (mRNA) | 200-gene set b |
a Discovery cohort was from single center, with external validation in a multicenter cohort.
b TruGraf assay from Transplant Genomics Inc. is based on this gene set and undergoing validation at the Mayo Clinic.
Potential Urinary Biomarkers
Being a direct product of the allograft, urine is a particularly attractive biofluid for evaluating potential biomarkers. Before the widespread application of the omics disciplines, substantial progress had been made by using qPCR to interrogate urine obtained concurrently from patients with biopsy-proven acute rejection for preselected immune activation genes of interest. In one of the early landmark studies, Suthanthiran and colleagues selected granzyme B, perforin, and cyclophilin B mRNA, the products of which are cytotoxic proteins implicated in animal and patient studies of allograft rejection, for evaluation in urinary cell pellets from patients with biopsy-proven allograft rejection. Granzyme B and perforin mRNA expression were shown to be increased in acute rejection. Using a similar targeted approach, the same group showed subsequently the increased expression of a number of other immune products known to be relevant to the pathophysiology of acute rejection. These were selected for further evaluation in the prospective, observational multicenter CTOT-4 study, one of projects conducted by the National Institutes of Health (NIH) -sponsored Collaborative Trials in Organ Transplantation study consortium (CTOT). In the CTOT-4 study, the investigators assessed whether urinary cell levels of mRNA encoding CD3e chain, perforin, granzyme B, proteinase inhibitor 9, CD103 (a cell surface marker expressed on alloreactive CD8+ T lymphocytes), interferon (IFN) -inducible protein 10 (IP-10, also known as CXCL10), and the chemokine receptor CXCR3 obtained at time of biopsy: (1) is diagnostic of acute rejection, and (2) whether changing expression levels in sequential urine specimens could predict the subsequent development of acute rejection. In this study 4300 urine specimens were collected from 485 renal transplant recipients at timed intervals and at clinically indicated biopsies. The combined measure of CD3e, IP-10, and 18s ribosomal RNA as a three-gene signature to discriminate ACR from no rejection yielded an ROC curve area under the curve (AUC) of 0.85 in the primary data set and 0.74 in an external validation set. The gene set was less effective at differentiating ACR from borderline changes (AUC 0.78) and AMR. Subsequently, a urinary six-gene signature (that was derived from measuring absolute levels of 26 prespecified mRNAs using qPCR) from a single-center study was shown to distinguish acute rejection from other causes of renal allograft dysfunction, and could further distinguish between ACR and AMR.
The evaluation of urinary proteins associated with acute rejection has also received much attention over the past decade, with CXCL10 and CXCL9 (monokines induced by IFN-γ) showing the greatest promise as potential biomarkers to date. Both these chemokines are secreted by infiltrating inflammatory cells and renal tubular and endothelial cells. Using an antibody array consisting of 120 chemokines and cytokines to screen the urine of recipients with allograft injury compared with those with stable function and healthy controls, with further confirmation using multiple-bead array, CXCL9 and CXCL10 were significantly elevated in acute renal injury. A number of other groups adopted a more targeted approach using ELISA to evaluate cytokines and tubular injury markers, which supported the potential of CXCL9 and/or CXCL10 as biomarkers in acute rejection (both clinical and subclinical), but, as they are elevated in other inflammatory states, they may not be specific for rejection. The CTOT-01 study, comprising 280 adult and pediatric first transplant recipients, evaluated a number of potential biomarkers including CCR1, CR5, CXCR3, CCL5 (RANTES), CXCL9, and CXCL10 using ELISA. Importantly, whereas the PPV for acute rejection was not high, CXCL9 urinary protein yielded a NPV of 92% (urinary CXCL9 mRNA NPV was 83%), and low urinary CXCL9 urinary protein levels 6 months posttransplant identified patients unlikely to develop an acute rejection episode or a 30% decline in their eGFR between 6 and 24 months (92.5%–99.3%). A more recent prospective, longitudinal single-center study, comprising 300 kidney transplant recipients, evaluated the predictive potential of CXCL9 and CXCL10 for a subsequent acute rejection episode. Early urinary CXCL10:creatinine levels predicted clinical and subclinical rejection and both ACR and AMR.
Although requiring validation in large prospective longitudinal cohorts, untargeted protein expression profiling studies have also uncovered potential candidate biomarkers both in urine and blood. Sidgel, Sarwal, and colleagues have used high-throughput proteomic and peptidomic techniques for both untargeted and targeted potential biomarker discovery in acute rejection. In one of their larger studies, isobaric tags for relative and absolute quantitation (iTRAQ)-based proteomic discovery and targeted ELISA validation were used in 262 renal allograft recipient urine samples from a single-center pediatric and young adult cohort (split into a training and independent validation set) with biopsy-confirmed acute rejection, chronic allograft injury, BK virus nephritis, stable grafts, and nephrotic syndrome, and healthy normal controls. In a more recent study, the same group applied state-of-the-art MS methods (either iTRAQ-based or label-free LC-MS) across 264 renal allograft biopsy-matched urine samples. Centralized and blinded histology data of the biopsies were used to classify urine samples into acute rejection, chronic allograft nephropathy, BK virus nephritis, and stable grafts. The study identified 958 unique urinary proteins, from which a set of 131 peptides was selected, and targeted quantification using the selected reaction monitoring (SRM) assay was performed in an independent validation cohort. Ultimately a set of 35 proteins identified for their ability to distinguish three major clinical phenotypes (acute rejection, chronic allograft nephropathy, and BK virus), with 11 of these urinary peptides for acute rejection yielding an ROC AUC of 0.93. As it stands, the high cost and complexities of the advanced proteomic technologies have limited its more widespread adoption and use in protein biomarker discovery and validation opportunities.
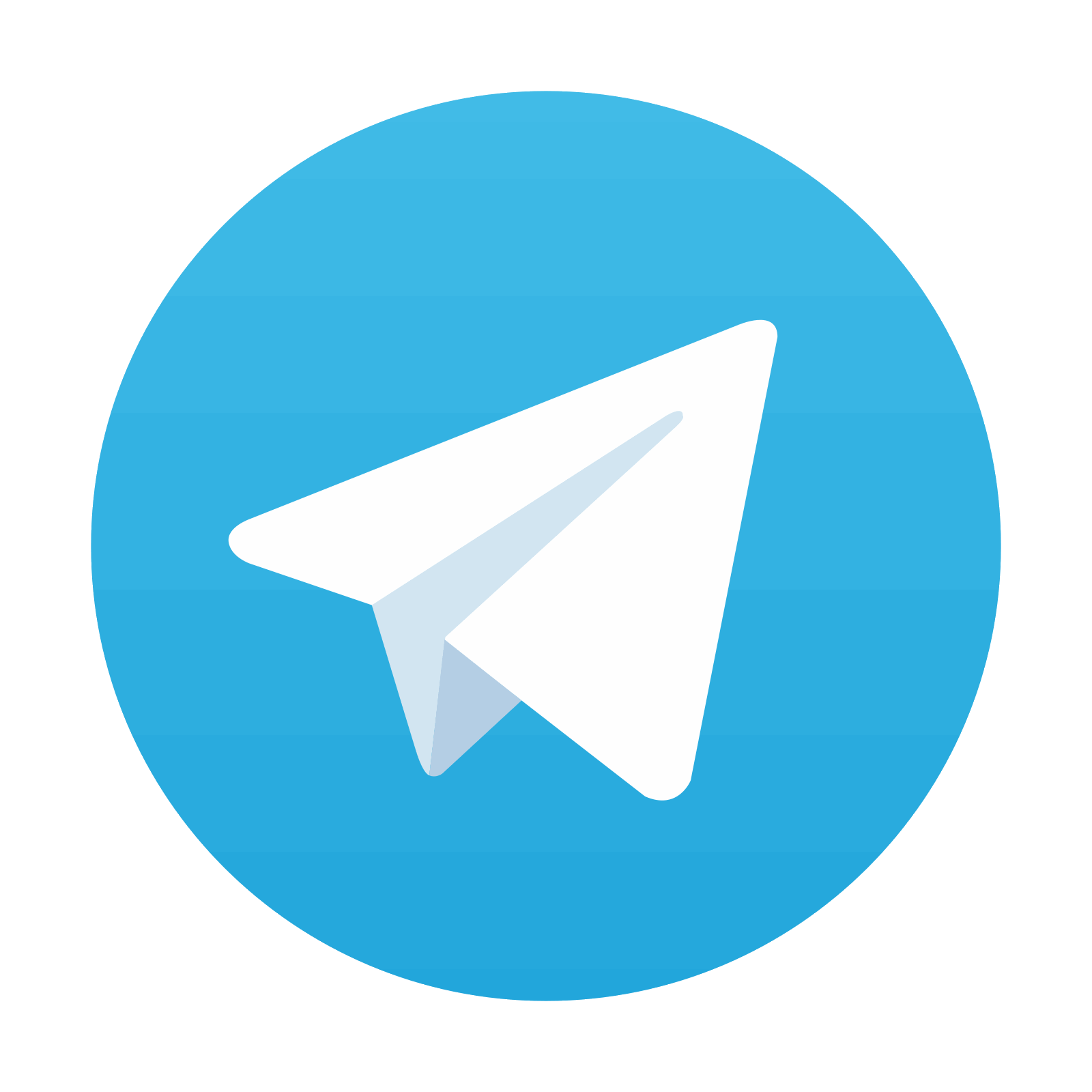
Stay updated, free articles. Join our Telegram channel

Full access? Get Clinical Tree
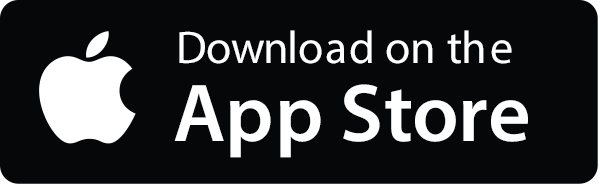
